Image-Based Service Recommendation System: A JPEG-Coefficient RFs Approach.
IEEE ACCESS(2020)
摘要
Online shopping platforms are growing at an unprecedented rate all over the world. These platforms mostly rely on search engines, which are still primarily based on the knowledge-base and use keywords matching for finding similar products. However, customers want an interactive setup that is convenient and reliable for querying related products. In this paper, we propose a novel idea of searching for products in an online shopping system using an image-based approach. A user can provide, select, or click an image, and similar image-based products will be presented to the user. The proposed recommendation system is based on content-based image retrieval and is composed of two major phases; Phase 1 and Phase 2. In Phase 1, the proposed approach learns the class/type of the product. In Phase 2, the proposed recommendation system retrieves closely matched similar products. For Phase 1, the proposed approach creates a model of products using Machine Learning (ML). The model is then used to find the category of the test products. From the ML perspectives, we employ the Random Forests (RF) classifier, and for feature extraction, we use the JPEG coefficients. The dataset used for proof of concepts includes 20 categories of products. For image-based recommendation, the proposed RF model is evaluated for Phase 1 and Phase 2. In Phase 1, the evaluation of the proposed model generates a 75% accurate model. For performance enhancements, the RF model has been integrated into the Deep Learning (DL) setup achieving 84% accurate predictions. Based on the custom evaluation approach for Phase 2, the proposed recommendation approach achieves 98% correct recommendations, thus demonstrating its efficacy for the product recommendation in practical applications.
更多查看译文
关键词
Recommendation system,services,machine learning,random forests,deep learning,SVM
AI 理解论文
溯源树
样例
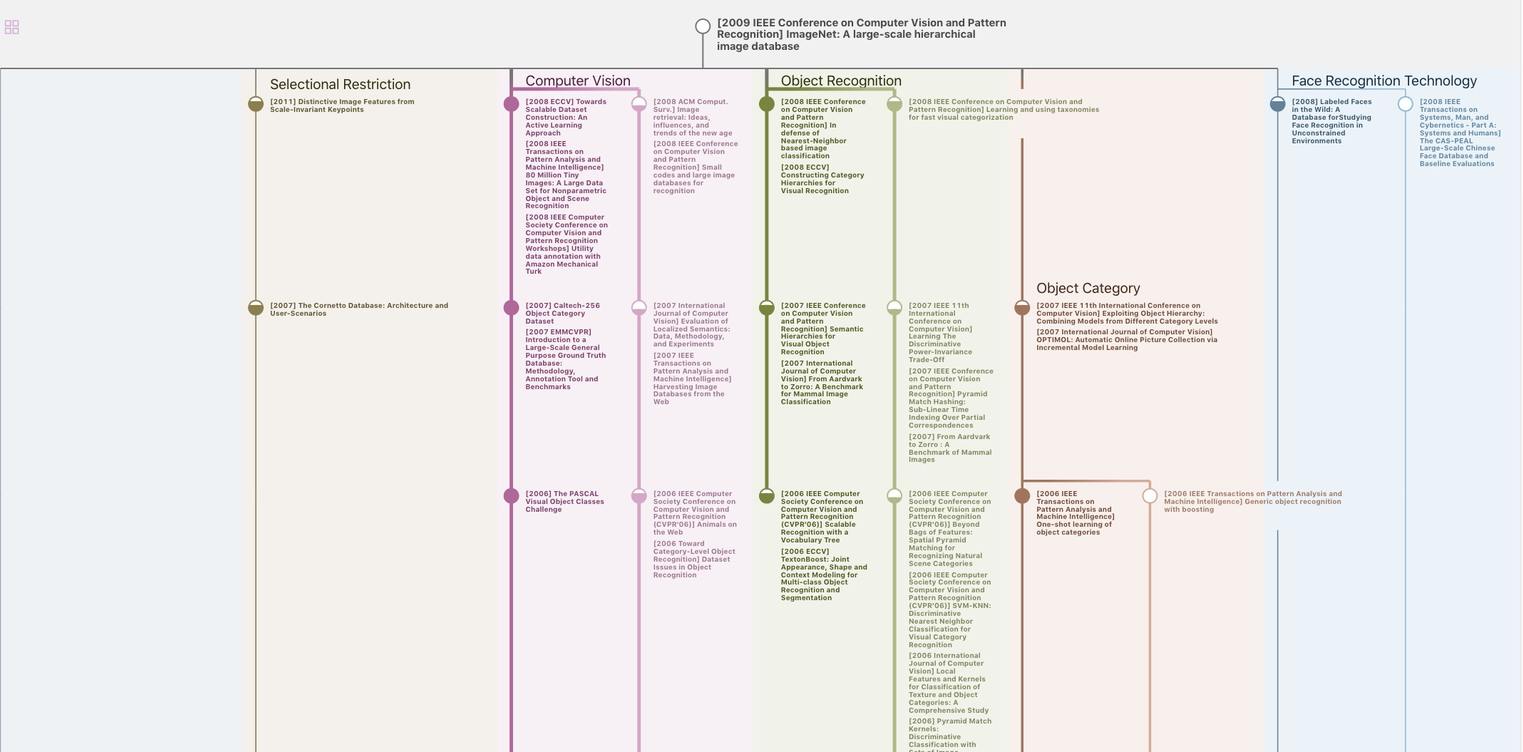
生成溯源树,研究论文发展脉络
Chat Paper
正在生成论文摘要