Learning and Uncertainty-Exploited Directional Antenna Control for Robust Long-Distance and Broad-Band Aerial Communication
IEEE Transactions on Vehicular Technology(2020)
摘要
Aerial communication using directional antennas (ACDA) is a promising solution to enable long-distance and broad-band unmanned aerial vehicle (UAV)-to-UAV networking. The automatic alignment of directional antennas allows the transmission energy to focus in certain direction and significantly extends the communication range and rejects interference. Robust automatic alignment of directional antennas is not easy to achieve, considering practical issues such as the limited on-board sensing devices due to the physical constraints of UAV payload and power supplies, uncertain and varying UAV movement patterns, and unstable GPS and unknown communication environments. In this paper, we develop reinforcement learning (RL)-based online antenna control solutions for the ACDA system to conquer these challenges. The control solution adopts an uncertain UAV mobility modeling and intention estimation framework to capture and predict the uncertain intentions of UAV maneuvers and hence permit robust tracking. To account for an unstable GPS environment, the control solution features a learning of communication channel models to provide additional measurement signals in GPS-denied settings. A novel stochastic optimal control solution for nonlinear random switching dynamics is developed that integrates RL, an effective uncertainty evaluation method called multivariate probabilistic collocation method (MPCM), and unscented Kalman Filter (UKF). Simulation studies are conducted to illustrate and validate the proposed solutions.
更多查看译文
关键词
Directional antennas,Antenna measurements,Global Positioning System,Optimal control,Switches,Stochastic processes
AI 理解论文
溯源树
样例
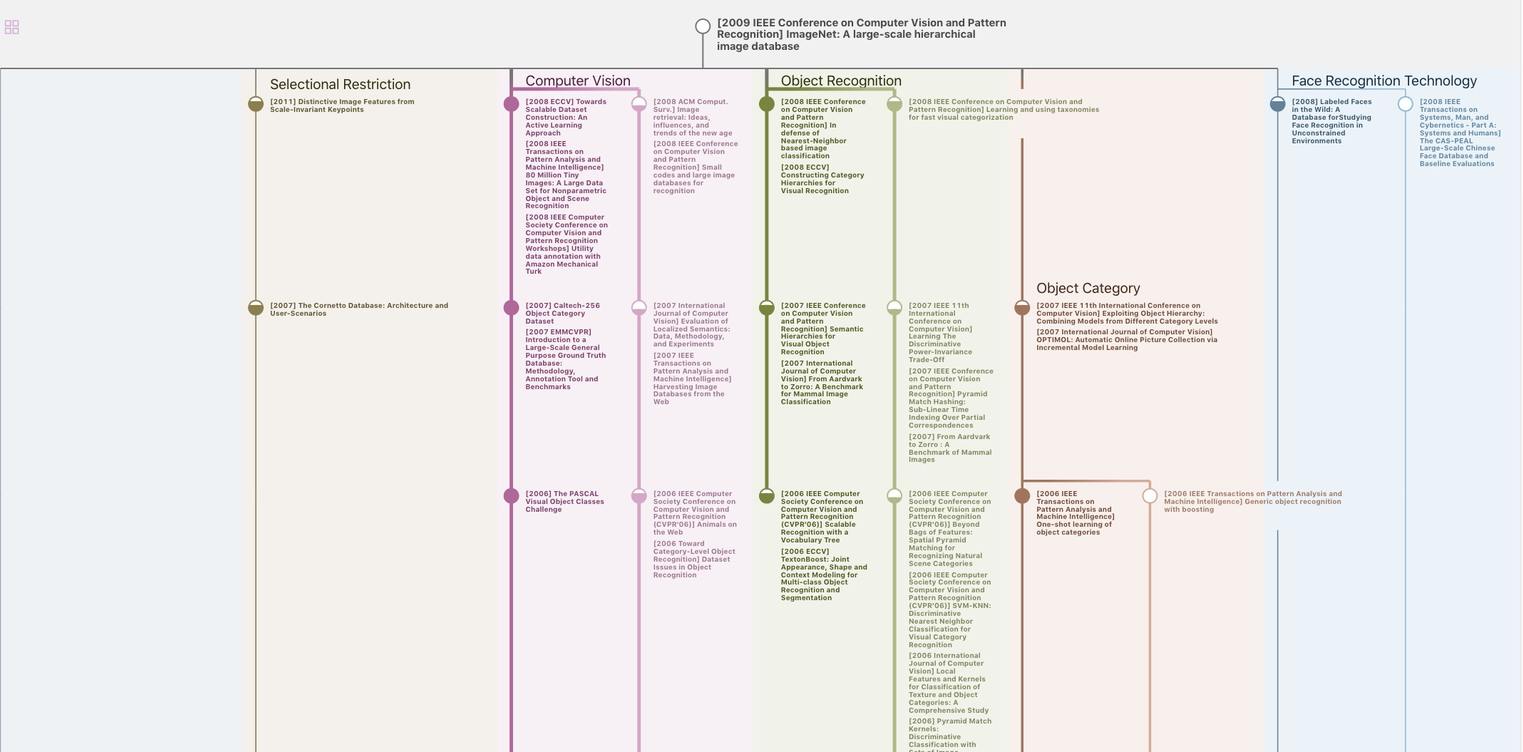
生成溯源树,研究论文发展脉络
Chat Paper
正在生成论文摘要