Masking schemes for universal marginalisers
arxiv(2020)
摘要
We consider the effect of structure-agnostic and structure-dependent masking schemes when training a universal marginaliser (arXiv:1711.00695) in order to learn conditional distributions of the form $P(x_i |\mathbf x_{\mathbf b})$, where $x_i$ is a given random variable and $\mathbf x_{\mathbf b}$ is some arbitrary subset of all random variables of the generative model of interest. In other words, we mimic the self-supervised training of a denoising autoencoder, where a dataset of unlabelled data is used as partially observed input and the neural approximator is optimised to minimise reconstruction loss. We focus on studying the underlying process of the partially observed data---how good is the neural approximator at learning all conditional distributions when the observation process at prediction time differs from the masking process during training? We compare networks trained with different masking schemes in terms of their predictive performance and generalisation properties.
更多查看译文
关键词
schemes,masking
AI 理解论文
溯源树
样例
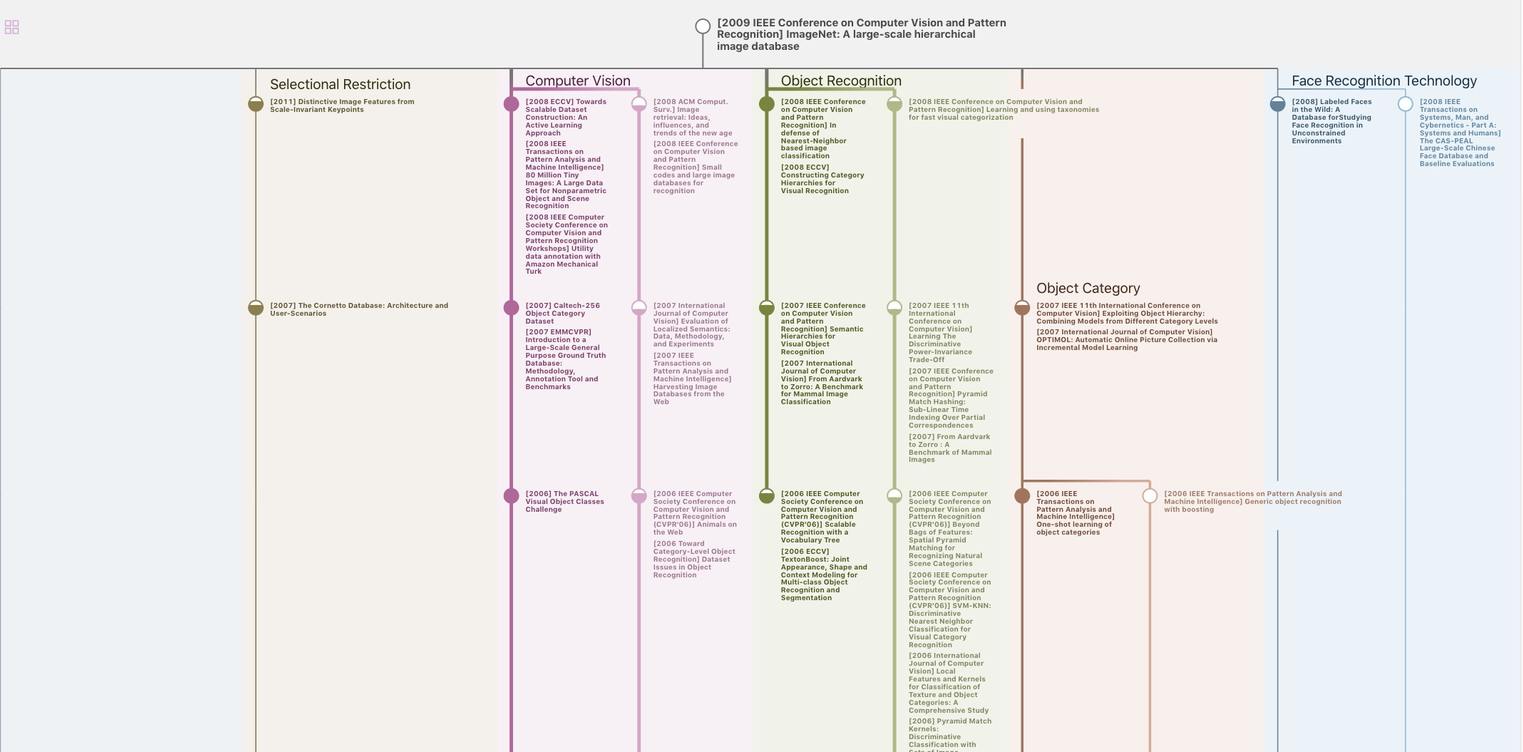
生成溯源树,研究论文发展脉络
Chat Paper
正在生成论文摘要