Comparing Rule-based, Feature-based and Deep Neural Methods for De-identification of Dutch Medical Records.
CoRR(2020)
摘要
Unstructured information in electronic health records provide an invaluable resource for medical research. To protect the confidentiality of patients and to conform to privacy regulations, de-identification methods automatically remove personally identifying information from these medical records. However, due to the unavailability of labeled data, most existing research is constrained to English medical text and little is known about the generalizability of de-identification methods across languages and domains. In this study, we construct a varied dataset consisting of the medical records of 1260 patients by sampling data from 9 institutes and three domains of Dutch healthcare. We test the generalizability of three de-identification methods across languages and domains. Our experiments show that an existing rule-based method specifically developed for the Dutch language fails to generalize to this new data. Furthermore, a state-of-the-art neural architecture performs strongly across languages and domains, even with limited training data. Compared to feature-based and rule-based methods the neural method requires significantly less configuration effort and domain-knowledge. We make all code and pre-trained de-identification models available to the research community, allowing practitioners to apply them to their datasets and to enable future benchmarks.
更多查看译文
关键词
dutch medical records,deep neural methods,rule-based,feature-based,de-identification
AI 理解论文
溯源树
样例
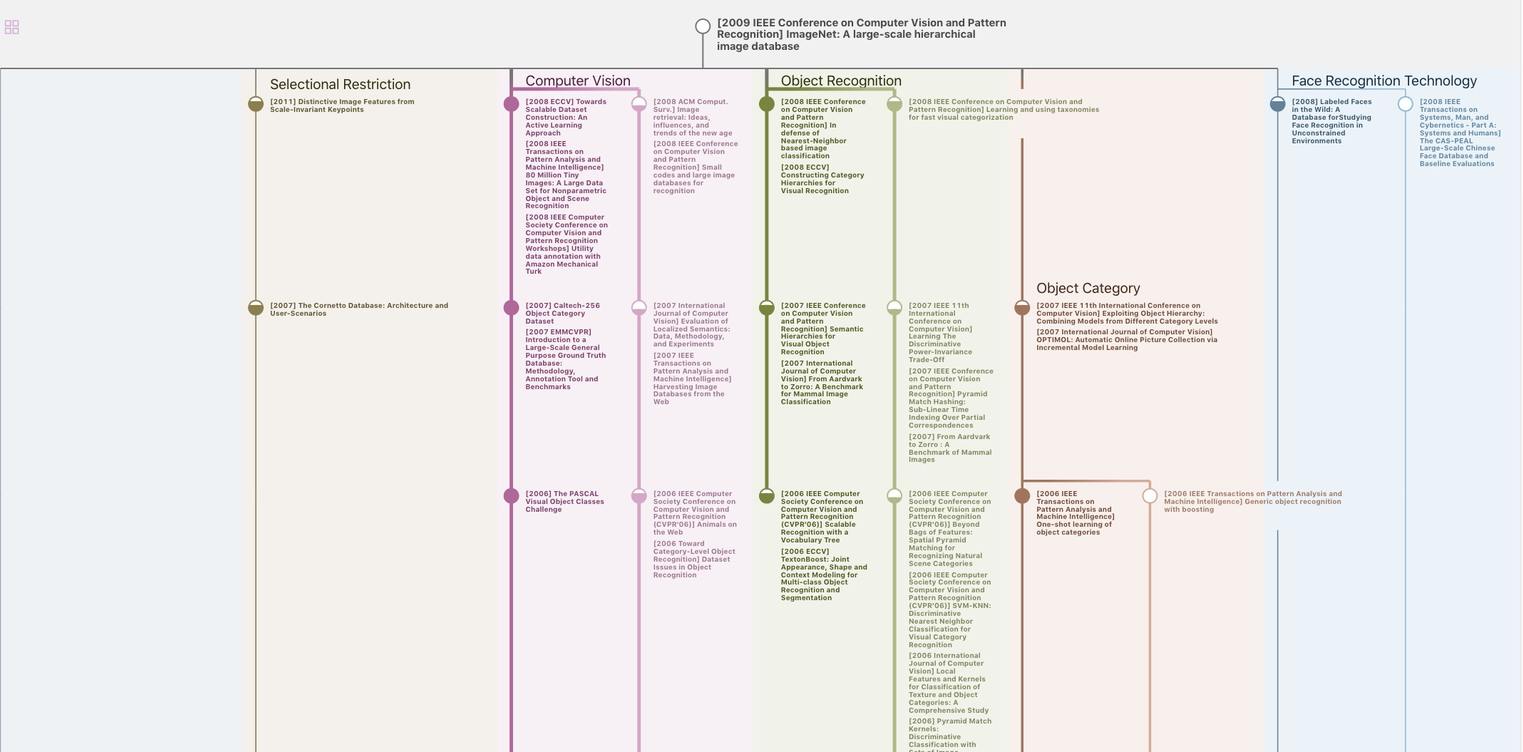
生成溯源树,研究论文发展脉络
Chat Paper
正在生成论文摘要