Symblicit exploration and elimination for probabilistic model checking
Symposium on Applied Computing(2021)
摘要
ABSTRACTBinary decision diagrams can compactly represent vast sets of states, mitigating the state space explosion problem in model checking. Probabilistic systems, however, require multi-terminal diagrams storing rational numbers. They are inefficient for models with many distinct probabilities and for iterative numeric algorithms like value iteration. In this paper, we present a new "symblicit" approach to checking Markov chains and related probabilistic models: We first generate a decision diagram that symbolically collects all reachable states and their predecessors. We then concretise states one-by-one into an explicit partial state space representation. Whenever all predecessors of a state have been concretised, we eliminate it from the explicit state space in a way that preserves all relevant probabilities and rewards. We thus keep few explicit states in memory at any time. Experiments show that very large models can be model-checked in this way with very low memory consumption.
更多查看译文
关键词
symblicit exploration,model
AI 理解论文
溯源树
样例
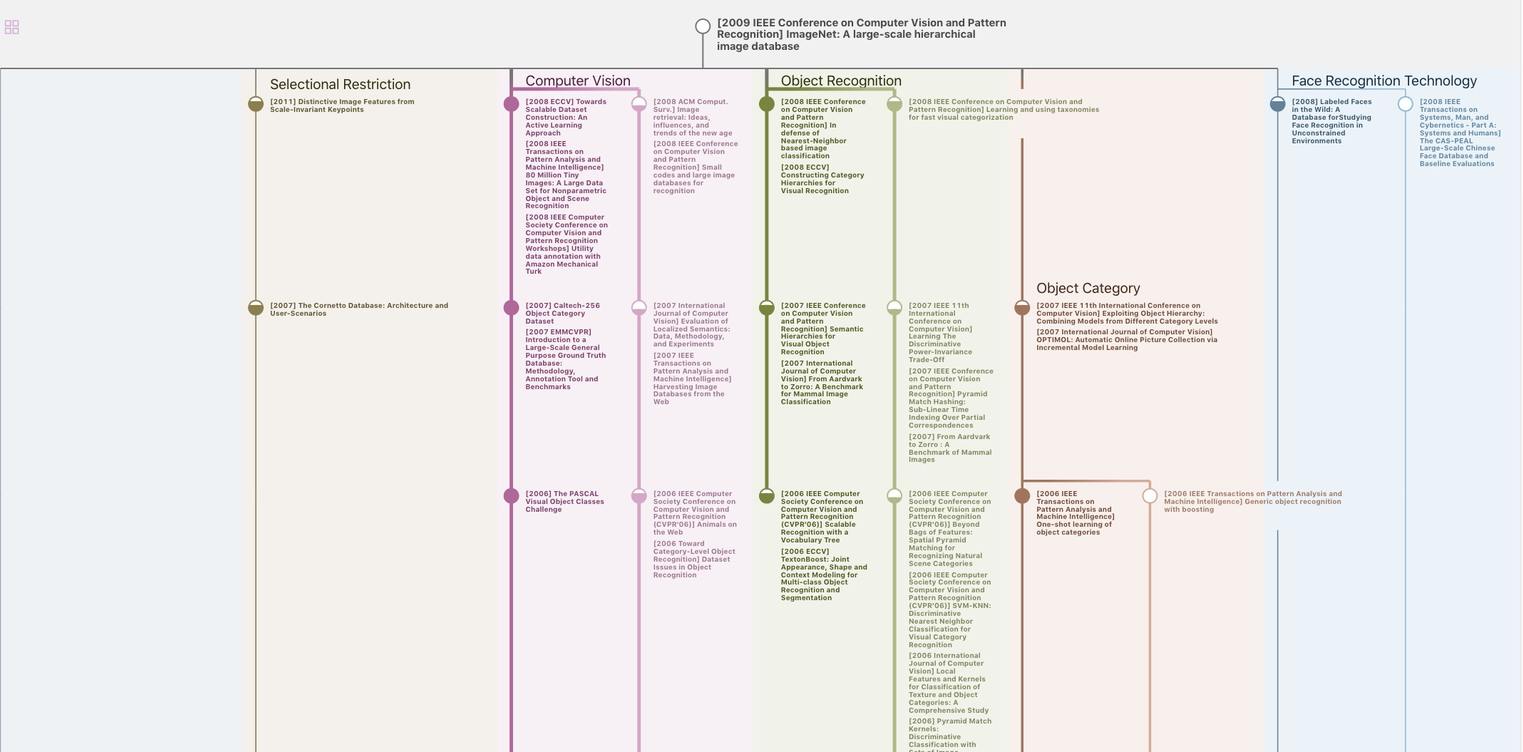
生成溯源树,研究论文发展脉络
Chat Paper
正在生成论文摘要