Entropy Regularized Power k-Means Clustering
arxiv(2020)
摘要
Despite its well-known shortcomings, $k$-means remains one of the most widely used approaches to data clustering. Current research continues to tackle its flaws while attempting to preserve its simplicity. Recently, the \textit{power $k$-means} algorithm was proposed to avoid trapping in local minima by annealing through a family of smoother surfaces. However, the approach lacks theoretical justification and fails in high dimensions when many features are irrelevant. This paper addresses these issues by introducing \textit{entropy regularization} to learn feature relevance while annealing. We prove consistency of the proposed approach and derive a scalable majorization-minimization algorithm that enjoys closed-form updates and convergence guarantees. In particular, our method retains the same computational complexity of $k$-means and power $k$-means, but yields significant improvements over both. Its merits are thoroughly assessed on a suite of real and synthetic data experiments.
更多查看译文
关键词
clustering,k-means
AI 理解论文
溯源树
样例
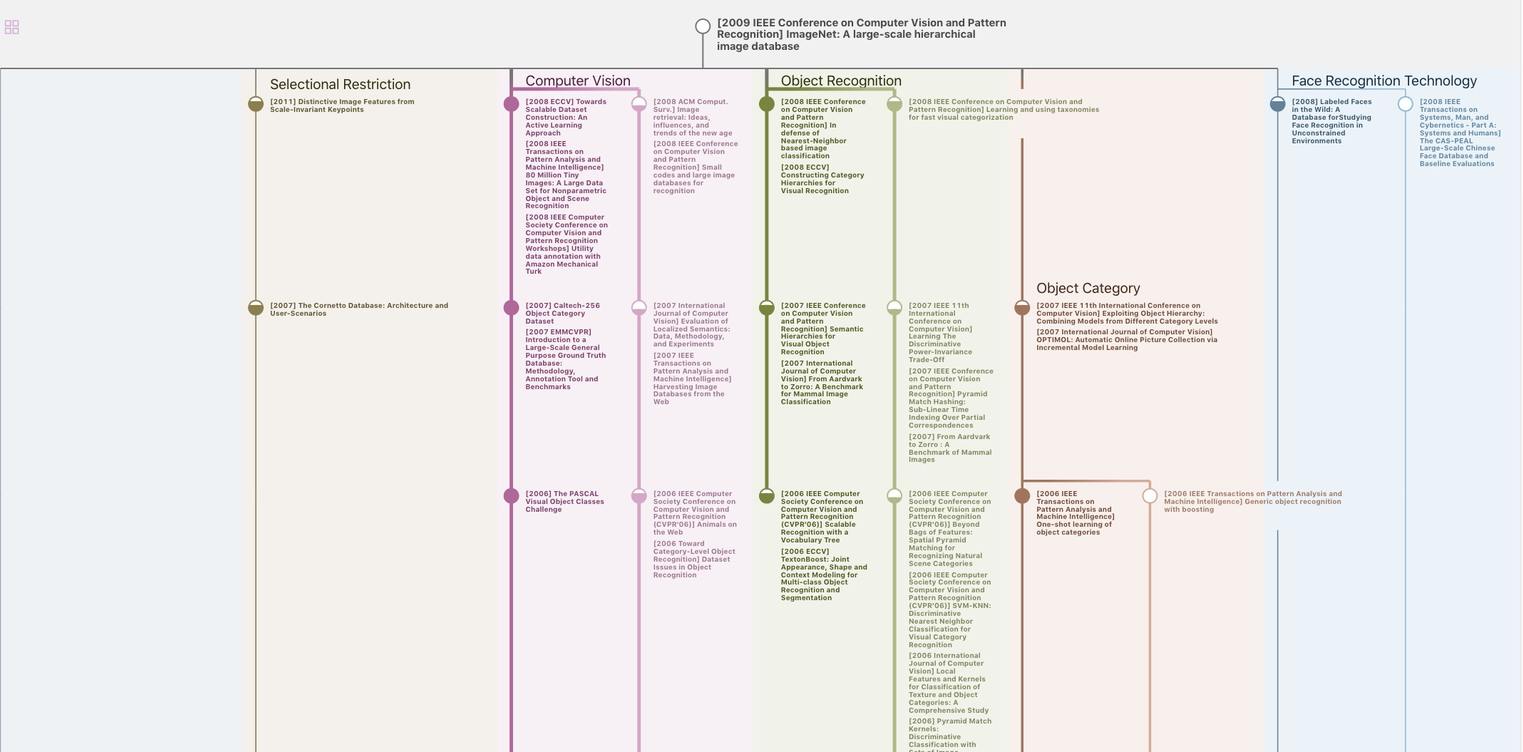
生成溯源树,研究论文发展脉络
Chat Paper
正在生成论文摘要