Training contextual neural networks with rectifier activation functions: Role and adoption of sorting methods.
JOURNAL OF INTELLIGENT & FUZZY SYSTEMS(2019)
摘要
Contextual neural networks are effective and very usable machine learning models being generalization of multilayer perceptron. They allow to solve classification problems with high accuracy while strongly limiting activity of connections between hidden neurons. Within this article we present novel study of properties of contextual neuronal networks with Hard and Exponential Rectifier activation functions and of their influence on behavior of the Generalized Error Backpropagation method. It is used to show how to optimize efficiency of the sorting phase of this algorithm when applied to train evaluated models. This considerably extends our previous related paper which was limited to analysis of contextual neuronal networks with Leaky Rectifier and Sigmoidal activation functions. This article includes wide description of contextual neural networks and generalized error backpropagation algorithm as well as the discussion of their connection with self-consistency paradigm, which is frequently used in quantum physics. Also the relation of the latter with sorting methods and considered rectifier functions during training of contextual neural networks is studied in details. Conclusions are backed up by the results of performed experiments. Reported outcomes of simulations confirm the ability of contextual neural networks to limit activity of connections between their neurons and - what is more important - indicate the detailed rules of selection of the most efficient sorting algorithm for updating scan-paths of contextual neurons that are using Hard and Exponential Rectifier activation functions. Presented results have considerable value both for research and practical applications - especially where the efficiency of training of contextual neural networks is crucial.
更多查看译文
关键词
Classifiers,self-consistency,aggregation functions,scan-paths
AI 理解论文
溯源树
样例
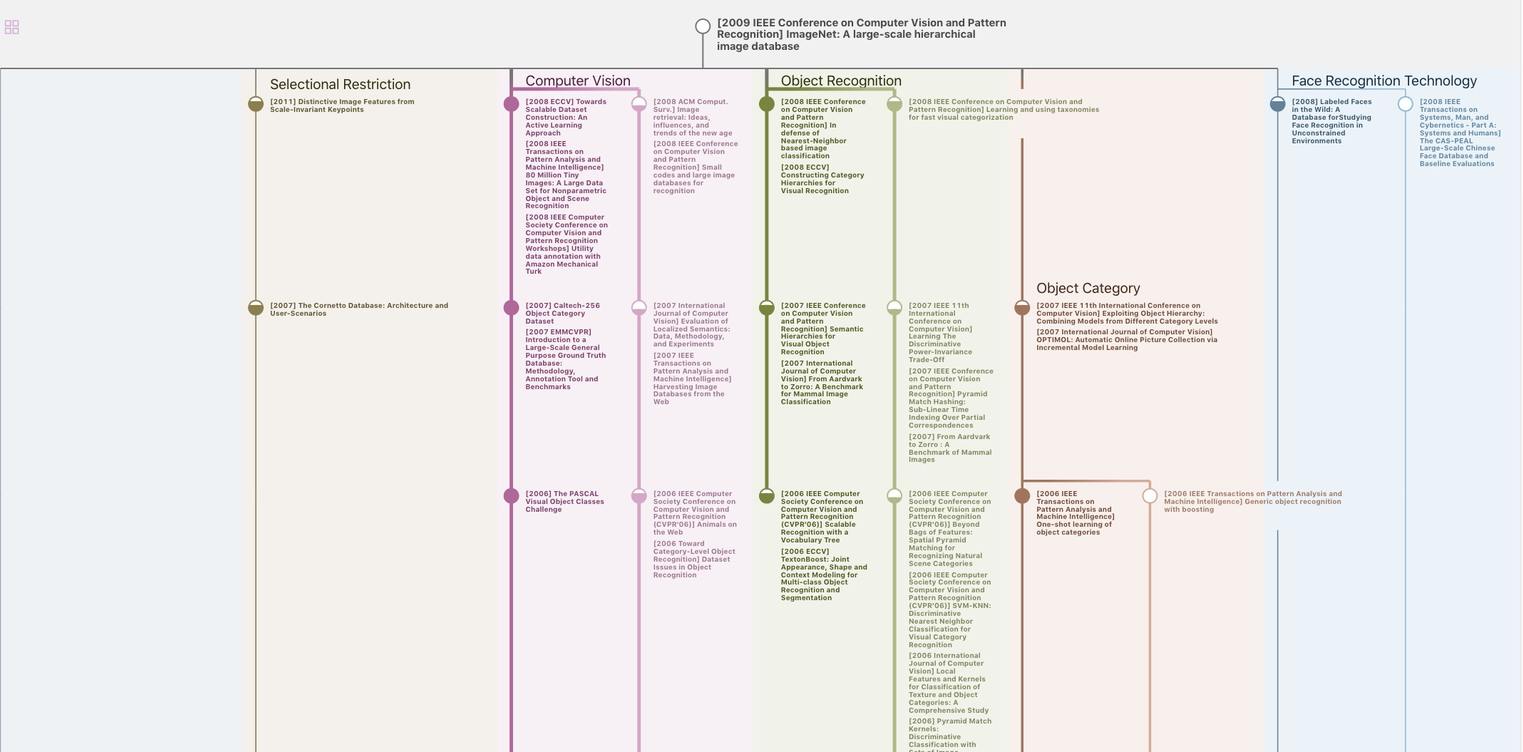
生成溯源树,研究论文发展脉络
Chat Paper
正在生成论文摘要