Scalable Gradients for Stochastic Differential Equations
INTERNATIONAL CONFERENCE ON ARTIFICIAL INTELLIGENCE AND STATISTICS, VOL 108(2020)
摘要
The adjoint sensitivity method scalably computes gradients of solutions to ordinary differential equations. We generalize this method to stochastic differential equations, allowing time-efficient and constant-memory computation of gradients with high-order adaptive solvers. Specifically, we derive a stochastic differential equation whose solution is the gradient, a memory-efficient algorithm for caching noise, and conditions under which numerical solutions converge. In addition, we combine our method with gradient-based stochastic variational inference for latent stochastic differential equations. We use our method to fit stochastic dynamics defined by neural networks, achieving competitive performance on a 50-dimensional motion capture dataset.
更多查看译文
关键词
stochastic differential equations,differential equations
AI 理解论文
溯源树
样例
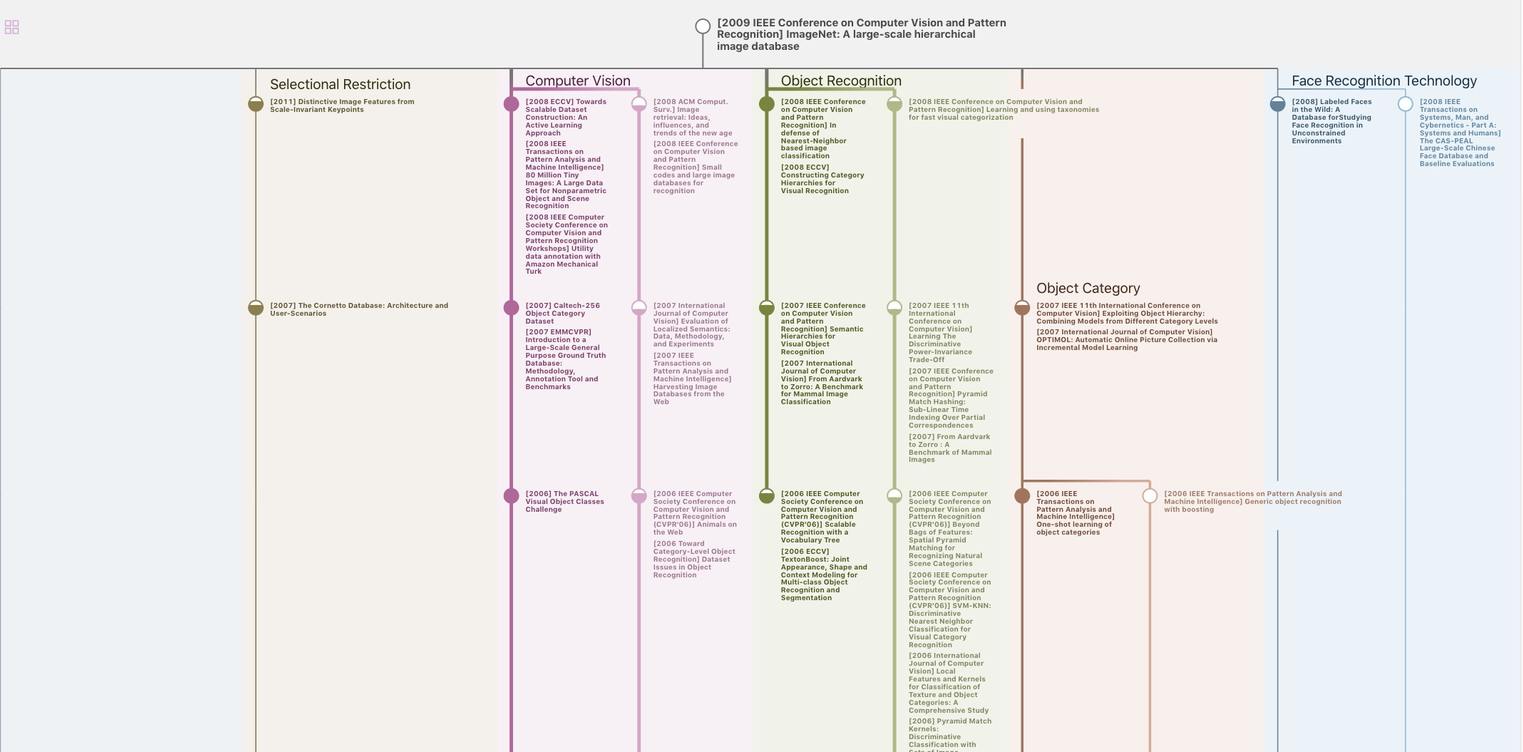
生成溯源树,研究论文发展脉络
Chat Paper
正在生成论文摘要