A Machine Learning Imaging Core using Separable FIR-IIR Filters
arxiv(2020)
摘要
We propose fixed-function neural network hardware that is designed to perform pixel-to-pixel image transformations in a highly efficient way. We use a fully trainable, fixed-topology neural network to build a model that can perform a wide variety of image processing tasks. Our model uses compressed skip lines and hybrid FIR-IIR blocks to reduce the latency and hardware footprint. Our proposed Machine Learning Imaging Core, dubbed MagIC, uses a silicon area of ~3mm^2 (in TSMC 16nm), which is orders of magnitude smaller than a comparable pixel-wise dense prediction model. MagIC requires no DDR bandwidth, no SRAM, and practically no external memory. Each MagIC core consumes 56mW (215 mW max power) at 500MHz and achieves an energy-efficient throughput of 23TOPS/W/mm^2. MagIC can be used as a multi-purpose image processing block in an imaging pipeline, approximating compute-heavy image processing applications, such as image deblurring, denoising, and colorization, within the power and silicon area limits of mobile devices.
更多查看译文
关键词
machine learning imaging core,fir-iir
AI 理解论文
溯源树
样例
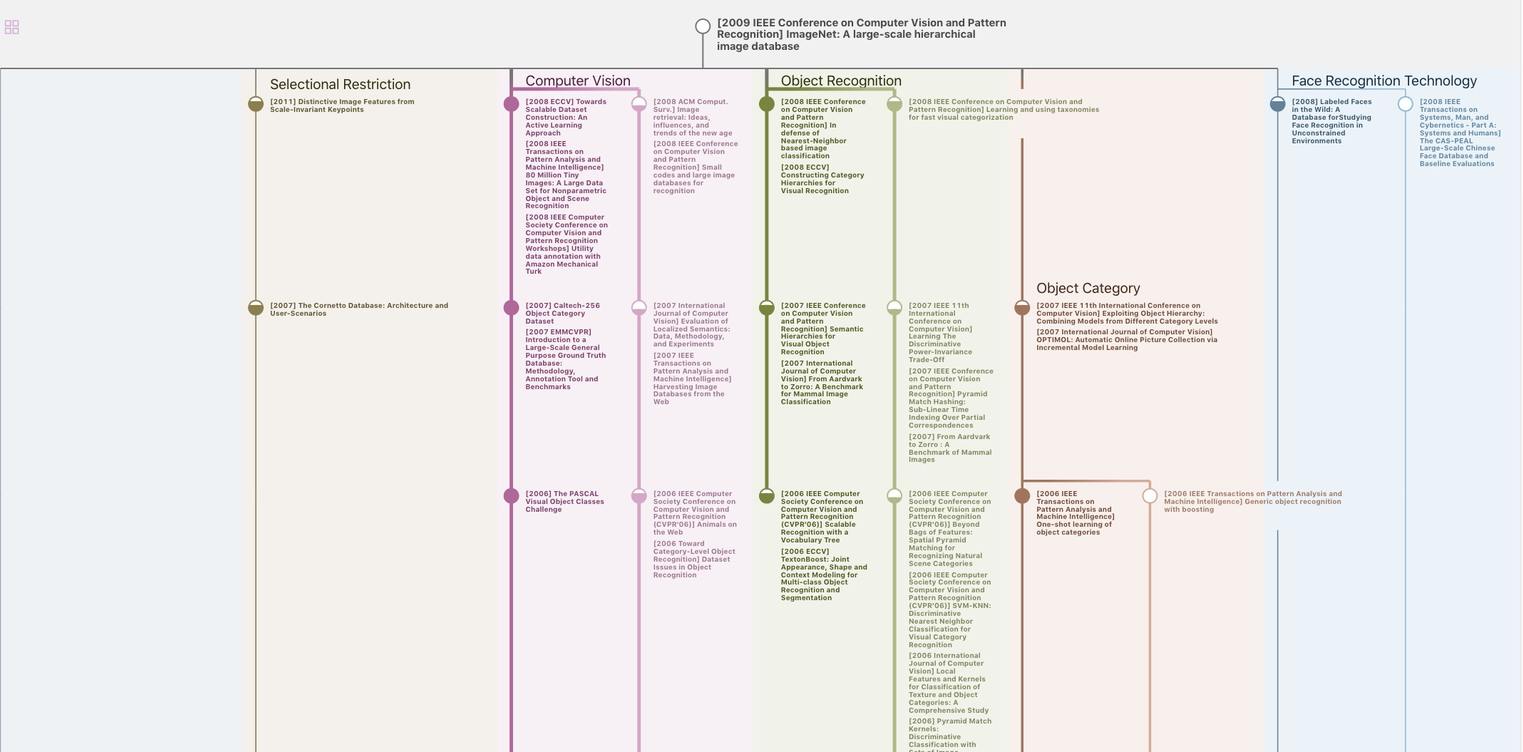
生成溯源树,研究论文发展脉络
Chat Paper
正在生成论文摘要