Defending from adversarial examples with a two-stream architecture
arxiv(2019)
摘要
In recent years, deep learning has shown impressive performance on many tasks. However, recent researches showed that deep learning systems are vulnerable to small, specially crafted perturbations that are imperceptible to humans. Images with such perturbations are the so called adversarial examples, which have proven to be an indisputable threat to the DNN based applications. The lack of better understanding of the DNNs has prevented the development of efficient defenses against adversarial examples. In this paper, we propose a two-stream architecture to protect CNN from attacking by adversarial examples. Our model draws on the idea of "two-stream" which commonly used in the security field, and successfully defends different kinds of attack methods by the differences of "high-resolution" and "low-resolution" networks in feature extraction. We provide a reasonable interpretation on why our two-stream architecture is difficult to defeat, and show experimentally that our method is hard to defeat with state-of-the-art attacks. We demonstrate that our two-stream architecture is robust to adversarial examples built by currently known attacking algorithms.
更多查看译文
关键词
adversarial examples,architecture,two-stream
AI 理解论文
溯源树
样例
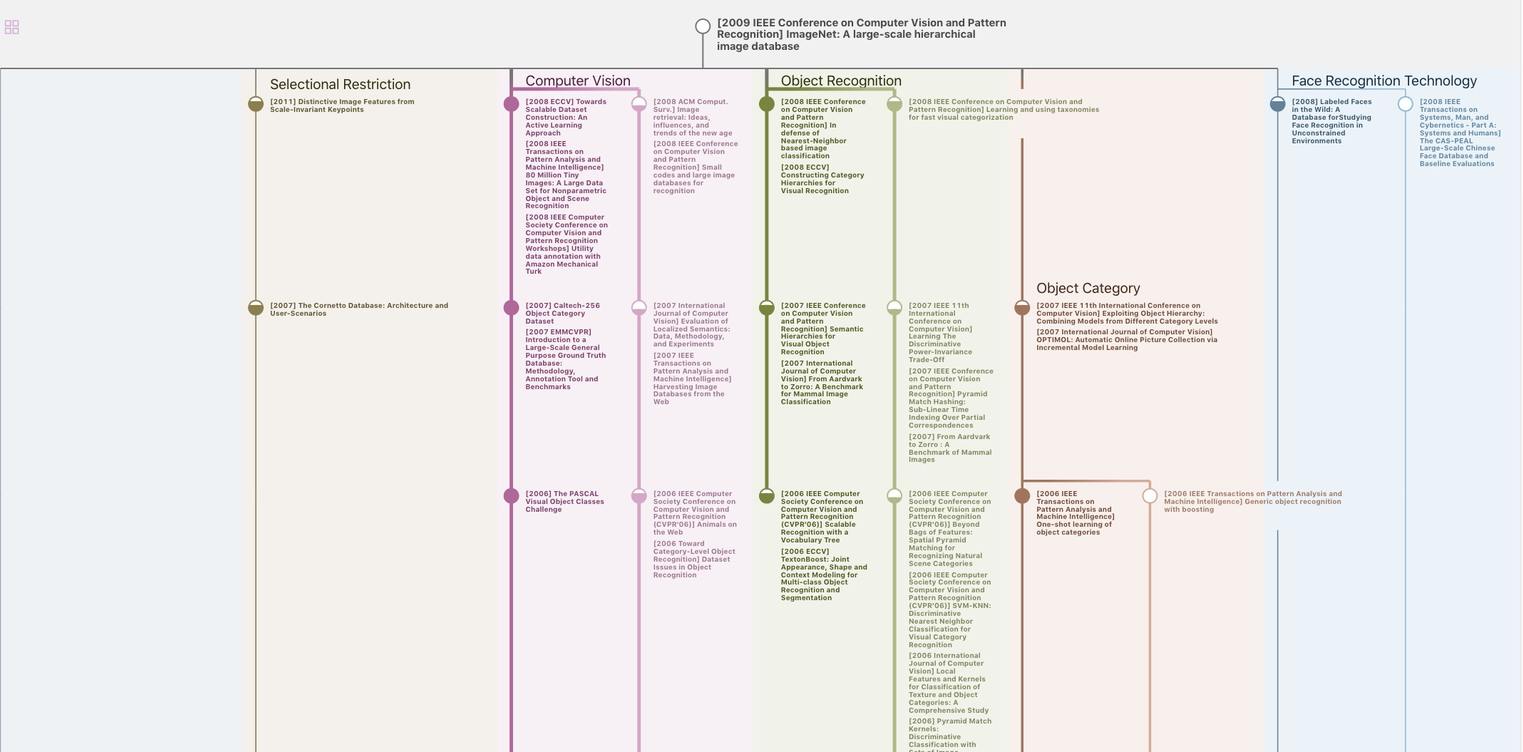
生成溯源树,研究论文发展脉络
Chat Paper
正在生成论文摘要