An unsupervised ensemble framework for node anomaly behavior detection in social network
Soft Computing(2019)
摘要
Large-scale and dynamic networks arise in cyberspace and financial security. Given a dynamic network, it is crucial to detect structural anomalies, such as node behaviors deviate from underlying majority of the network. However, anomaly analysis for dynamic networks is difficult to precisely detect the anomalous behaviors of nodes because it usually ignores the evolutionary behaviors of different nodes. Our work taps into this gap and proposes an unsupervised ensemble framework for node temporal behavior modeling and node behavior real-time anomaly detection. Specifically, a latent space model is used to model the node behavior; each node is assigned a probability distribution across a small set of roles based on that node’s features. The evolutionary behavior of node is represented as node roles change over time and the anomalies of node are identified as deviations from expected roles. The entropy-based ensembles method is proposed to combine with multiple unsupervised anomaly detectors to yield robust performances, which achieves the real-time anomaly detection for different types of node behaviors. Finally, we show the effectiveness of the proposed method on Enron network in the experiments.
更多查看译文
关键词
Network node modeling, Anomaly behavior detection, Entropy-based ensembles
AI 理解论文
溯源树
样例
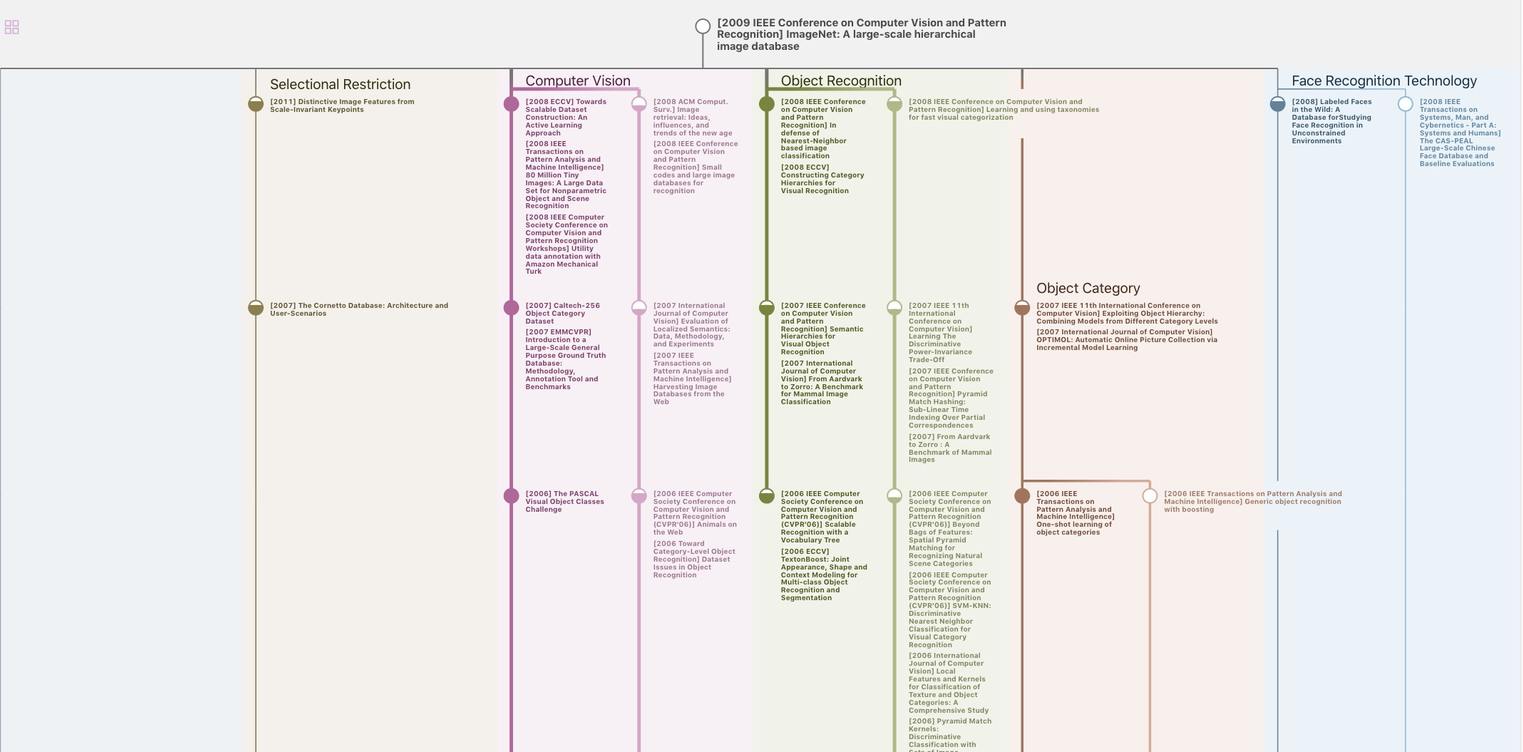
生成溯源树,研究论文发展脉络
Chat Paper
正在生成论文摘要