Universal Adversarial Triggers for Attacking and Analyzing NLP WARNING: This paper contains model outputs which are offensive in nature
EMNLP/IJCNLP (1)(2019)
摘要
Adversarial examples highlight model vulnerabilities and are useful for evaluation and interpretation. We define universal adversarial triggers: input-agnostic sequences of tokens that trigger a model to produce a specific prediction when concatenated to any input from a dataset. We propose a gradientguided search over tokens which finds short trigger sequences (e.g., one word for classification and four words for language modeling) that successfully trigger the target prediction. For example, triggers cause SNLI entailment accuracy to drop from 89.94% to 0.55%, 72% of "why" questions in SQuAD to be answered "to kill american people", and the GPT-2 language model to spew racist output even when conditioned on non-racial contexts. Furthermore, although the triggers are optimized using white-box access to a specific model, they transfer to other models for all tasks we consider. Finally, since triggers are input-agnostic, they provide an analysis of global model behavior. For instance, they confirm that SNLI models exploit dataset biases and help to diagnose heuristics learned by reading comprehension models.
更多查看译文
关键词
universal adversarial triggers,analyzing nlp,attacking
AI 理解论文
溯源树
样例
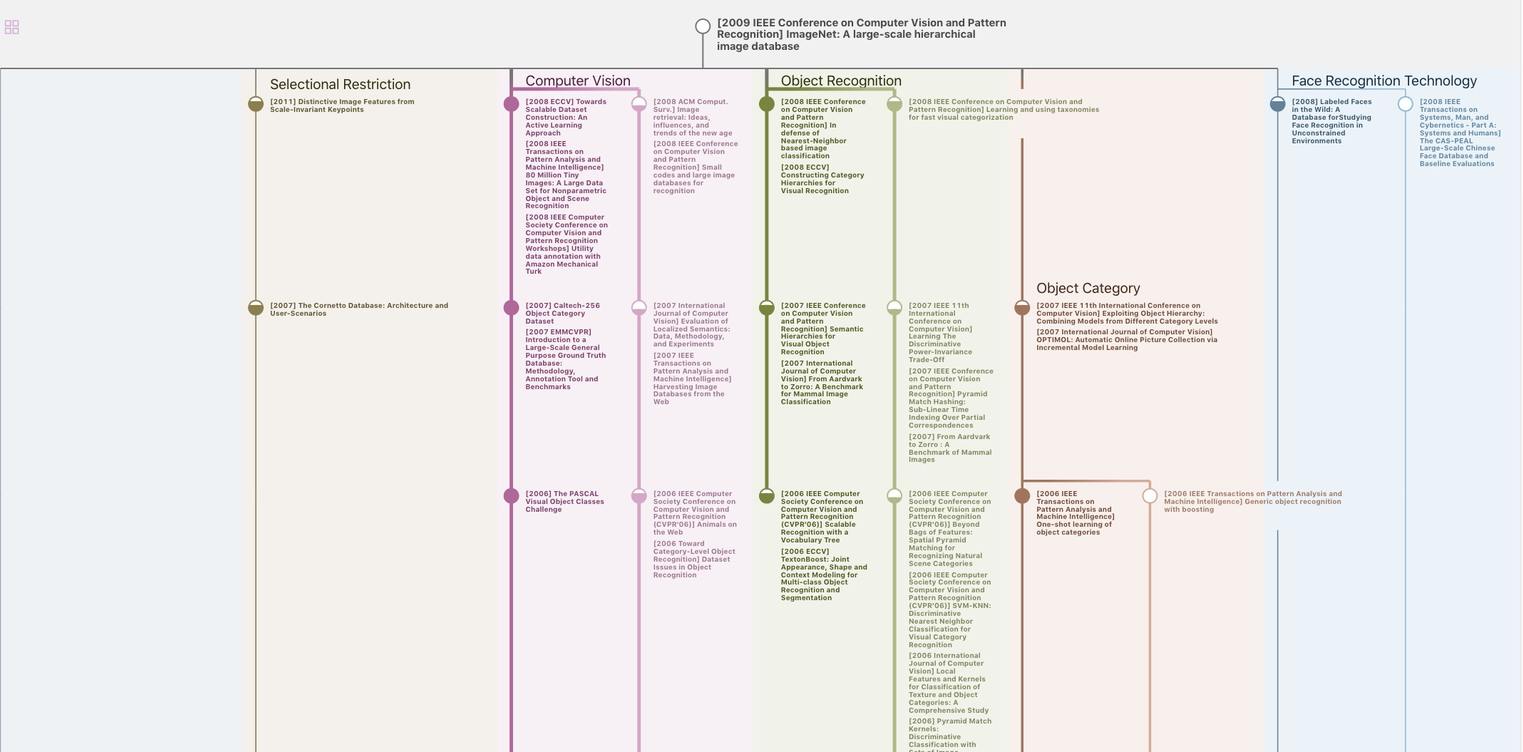
生成溯源树,研究论文发展脉络
Chat Paper
正在生成论文摘要