Target Discrimination Based on Weakly Supervised Learning for High-Resolution SAR Images in Complex Scenes
IEEE Transactions on Geoscience and Remote Sensing(2020)
摘要
To design a highly automatic and practical discrimination method for high-resolution synthetic aperture radar (SAR) images in complex scenes, a novel target discrimination framework based on weakly supervised learning (WSL) of the mid-level features is proposed in this article. First, we extract the dense SAR scale-invariant feature transform (SAR-SIFT) features of the candidate regions obtained from the detected SAR images. Then, the dense SAR-SIFT descriptors are transformed into richer mid-level features by coding and pooling. Finally, the mid-level features are input into a WSL-based target discrimination method, where the training set is initially selected by the unsupervised latent Dirichlet allocation (LDA) and iteratively updated by the linear support vector machine (SVM) discriminator. In the proposed method, only the image-level annotations (weak labels), which indicate whether the images containing the targets of interest or not, are required. By introducing WSL, the manual annotations of target regions from SAR images can be avoided, which is generally expensive in complex scenes and may tend to be less accurate and unreliable for the occluded or camouflaged targets. The comprehensive and specific experiments on the measured SAR data have demonstrated the effectiveness of the proposed method in benchmarking with the supervised learning-based linear SVM and linear support vector data description (SVDD) discriminators.
更多查看译文
关键词
Feature extraction,Synthetic aperture radar,Radar polarimetry,Training,Support vector machines,Optical imaging,Clutter
AI 理解论文
溯源树
样例
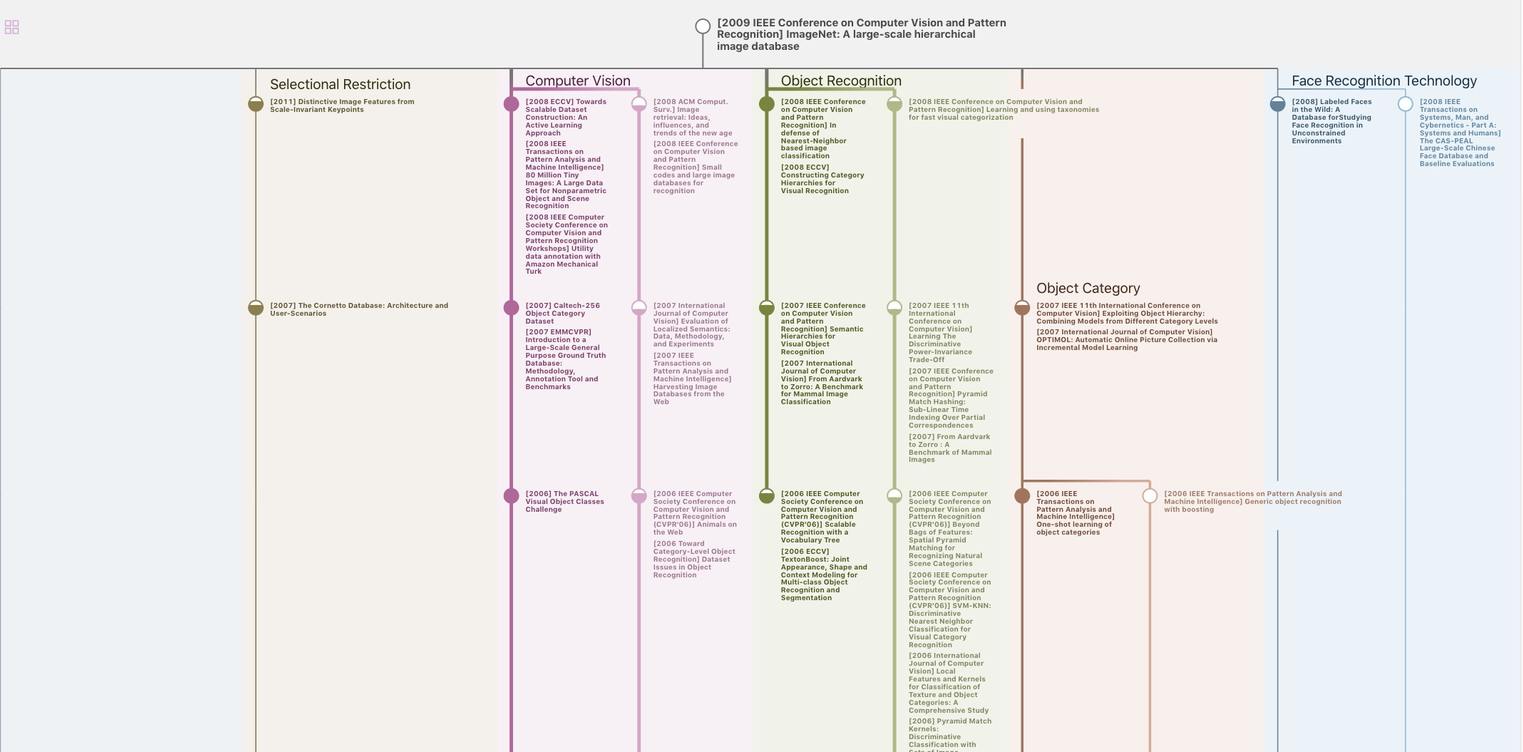
生成溯源树,研究论文发展脉络
Chat Paper
正在生成论文摘要