The Labeling Distribution Matrix (LDM): A Tool for Estimating Machine Learning Algorithm Capacity
ICAART: PROCEEDINGS OF THE 12TH INTERNATIONAL CONFERENCE ON AGENTS AND ARTIFICIAL INTELLIGENCE, VOL 2(2020)
摘要
Algorithm performance in supervised learning is a combination of memorization, generalization, and luck. By estimating how much information an algorithm can memorize from a dataset, we can set a lower bound on the amount of performance due to other factors such as generalization and luck. With this goal in mind, we introduce the Labeling Distribution Matrix (LDM) as a tool for estimating the capacity of learning algorithms. The method attempts to characterize the diversity of possible outputs by an algorithm for different training datasets, using this to measure algorithm flexibility and responsiveness to data. We test the method on several supervised learning algorithms, and find that while the results are not conclusive, the LDM does allow us to gain potentially valuable insight into the prediction behavior of algorithms. We also introduce the Label Recorder as an additional tool for estimating algorithm capacity, with more promising initial results.
更多查看译文
关键词
Machine Learning,Model Complexity,Algorithm Capacity,VC Dimension,Label Recorder
AI 理解论文
溯源树
样例
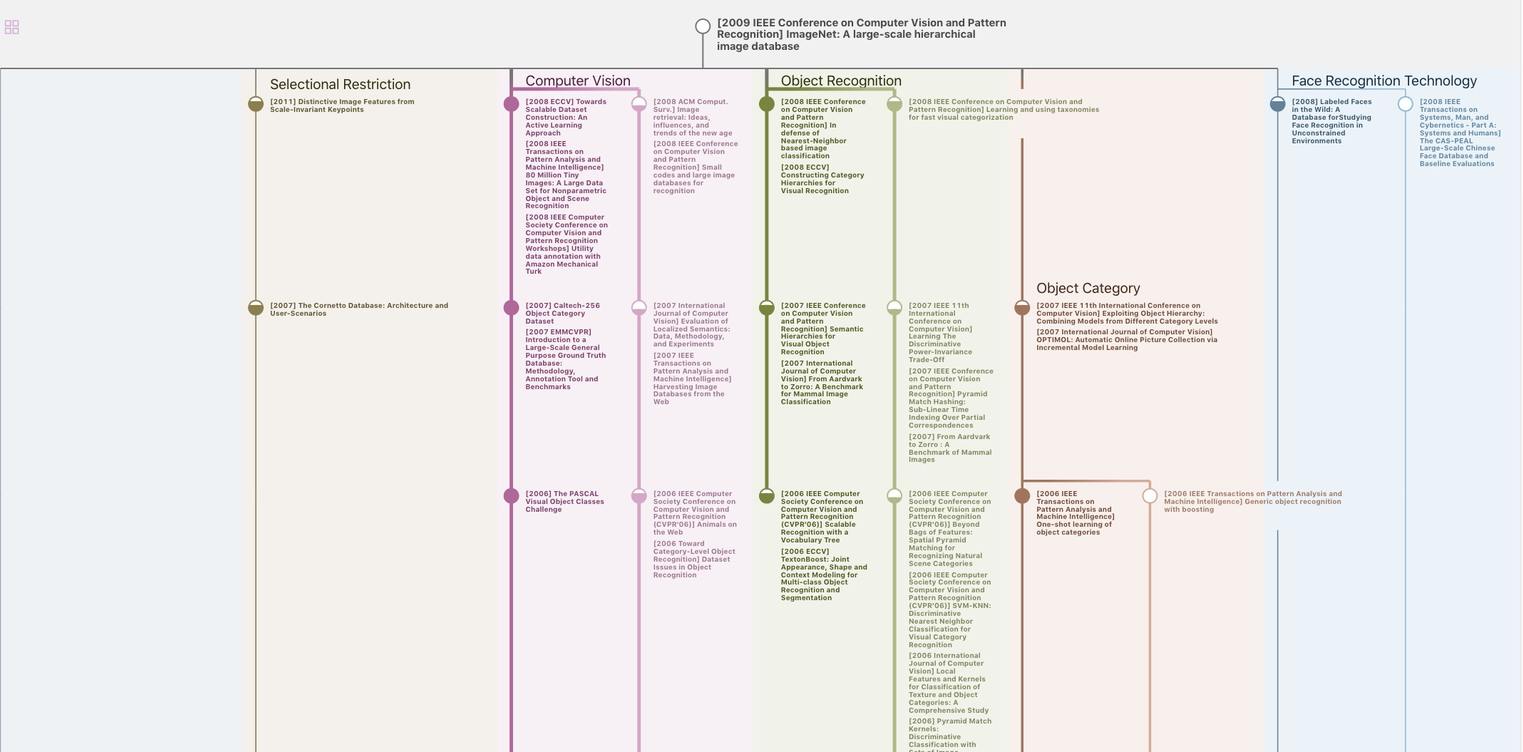
生成溯源树,研究论文发展脉络
Chat Paper
正在生成论文摘要