Weighted Personalized Factorizations for Network Classification with Approximated Relation Weights.
ICAART(2019)
摘要
Classifying Multi-Label nodes in networks is a well-known and widely used task in different domains. Current classification models rely on mining the network structure either by random walks or through approximating the laplacian of the network graph which gives insight about the nodes’ neighborhood. In implicit feedback relations, these models assume all relation edges to be equally strong and important. However, in real life, this is not necessarily the case as some edges might have different semantic weights such as friendship relation. To tackle this limitation we propose in this paper a weighted two-stage multi-relational matrix factorization model with Bayesian personalized ranking loss for network classification that utilizes different weighting functions for approximating the implicit feedback relation weights. Experiments on four real-world datasets show that the proposed model significantly outperforms the state-of-art models. Results also show that selecting the right weighting functions for approximating relation weights significantly improves classification accuracy.
更多查看译文
关键词
Multi-relational learning, Network representations, Multi-label classification, Recommender systems, Document classification
AI 理解论文
溯源树
样例
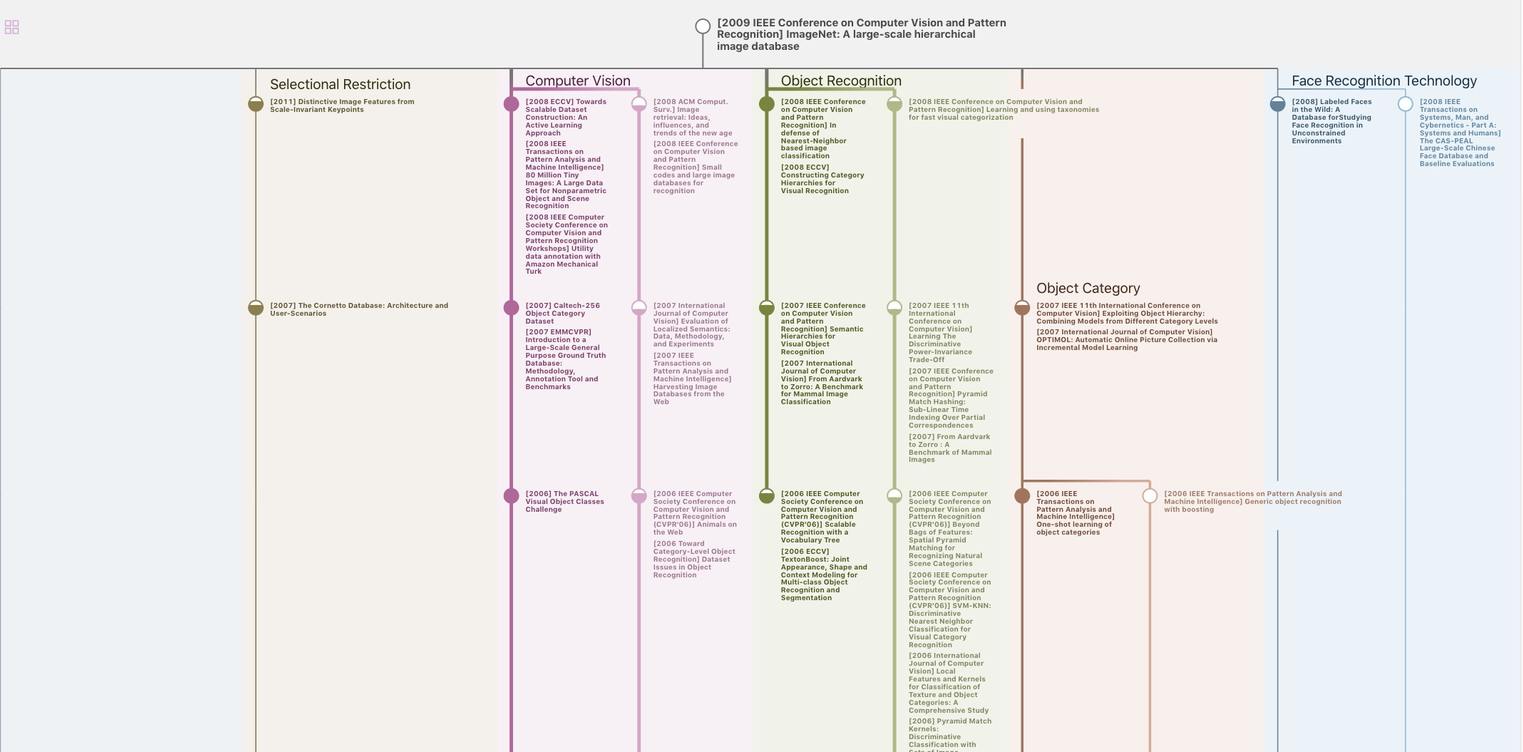
生成溯源树,研究论文发展脉络
Chat Paper
正在生成论文摘要