Asynchronous Spiking Neurons, the Natural Key to Exploit Temporal Sparsity
IEEE Journal on Emerging and Selected Topics in Circuits and Systems(2019)
摘要
Inference of Deep Neural Networks for stream signal (Video/Audio) processing in edge devices is still challenging. Unlike the most state of the art inference engines which are efficient for static signals, our brain is optimized for real-time dynamic signal processing. We believe one important feature of the brain (asynchronous state-full processing) is the key to its excellence in this domain. In this work, we show how asynchronous processing with state-full neurons allows exploitation of the existing sparsity in natural signals. This paper explains three different types of sparsity and proposes an inference algorithm which exploits all types of sparsities in the execution of already trained networks. Our experiments in three different applications (Handwritten digit recognition, Autonomous Steering and Hand-Gesture recognition) show that this model of inference reduces the number of required operations for sparse input data by a factor of one to two orders of magnitudes. Additionally, due to fully asynchronous processing this type of inference can be run on fully distributed and scalable neuromorphic hardware platforms.
更多查看译文
关键词
Neural networks,Artificial neural networks,Inference algorithms,Algorithm design and analysis,Asynchronous communication
AI 理解论文
溯源树
样例
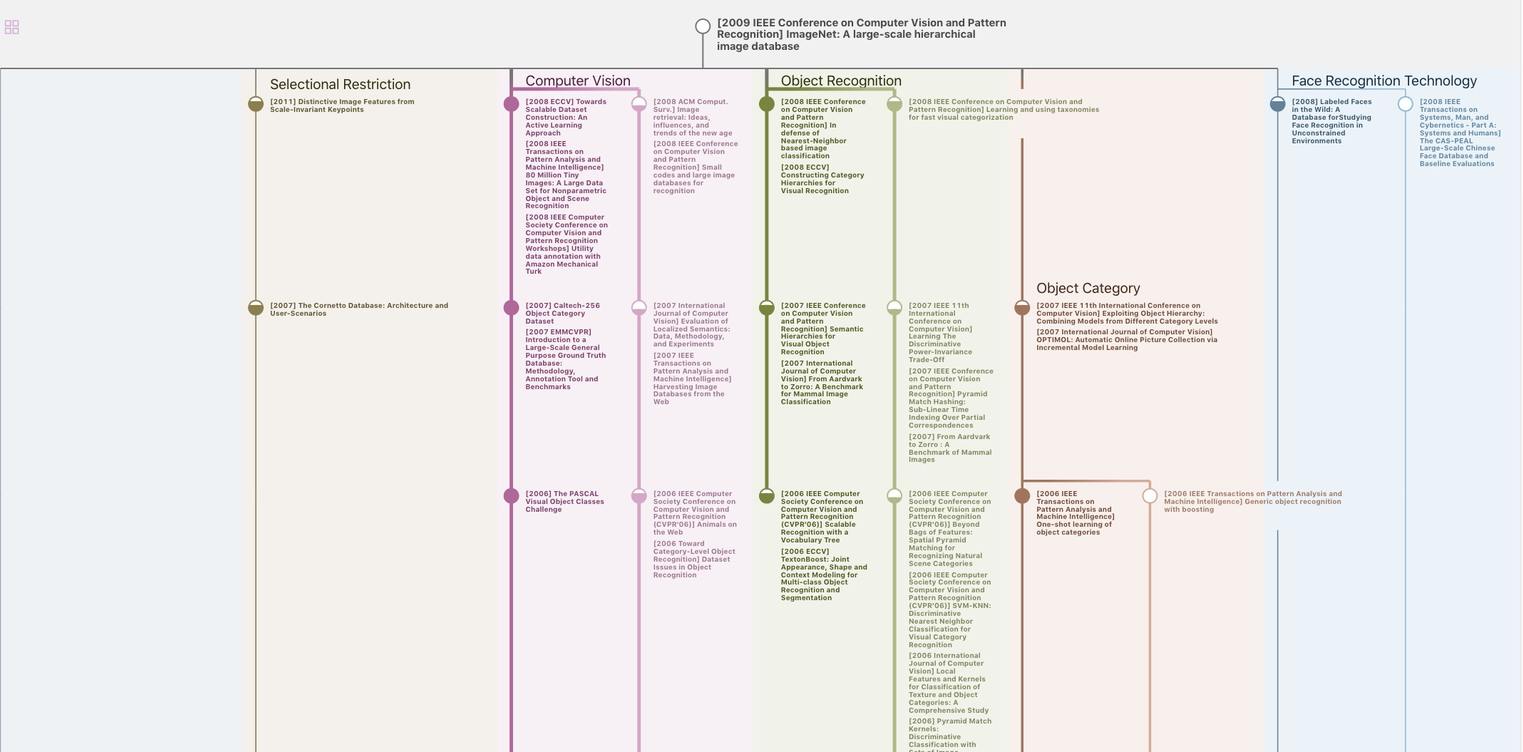
生成溯源树,研究论文发展脉络
Chat Paper
正在生成论文摘要