Associative Alignment for Few-Shot Image Classification
European Conference on Computer Vision(2020)
摘要
Few-shot image classification aims at training a model from only a few examples for each of the “novel” classes. This paper proposes the idea of associative alignment for leveraging part of the base data by aligning the novel training instances to the closely related ones in the base training set. This expands the size of the effective novel training set by adding extra “related base” instances to the few novel ones, thereby allowing a constructive fine-tuning. We propose two associative alignment strategies: 1) a metric-learning loss for minimizing the distance between related base samples and the centroid of novel instances in the feature space, and 2) a conditional adversarial alignment loss based on the Wasserstein distance. Experiments on four standard datasets and three backbones demonstrate that combining our centroid-based alignment loss results in absolute accuracy improvements of 4.4%, 1.2%, and 6.2% in 5-shot learning over the state of the art for object recognition, fine-grained classification, and cross-domain adaptation, respectively.
更多查看译文
关键词
Associative alignment, Few-shot image classification
AI 理解论文
溯源树
样例
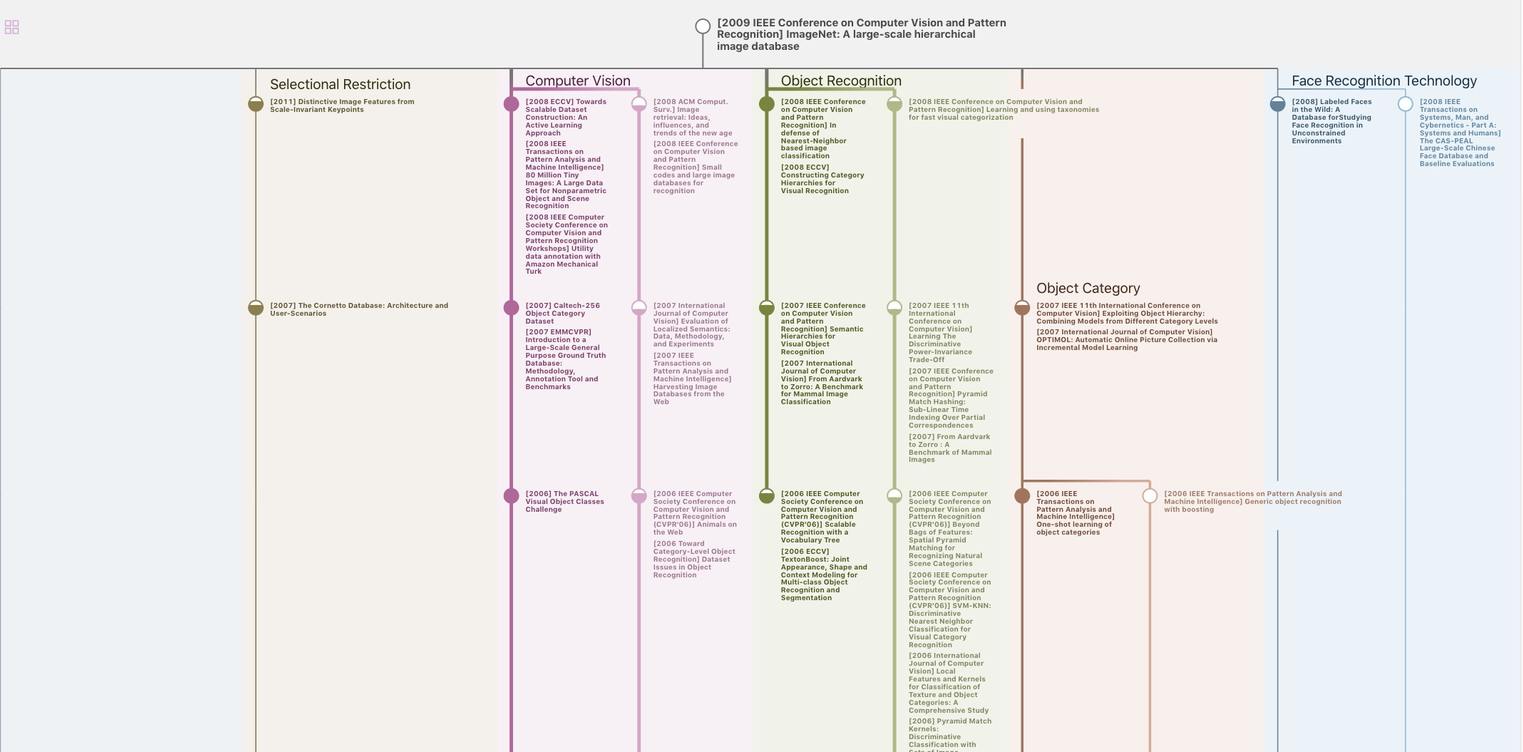
生成溯源树,研究论文发展脉络
Chat Paper
正在生成论文摘要