Near-Optimal Rapid MPC Using Neural Networks: A Primal-Dual Policy Learning Framework
IEEE Transactions on Control Systems Technology(2021)
摘要
In this article, we propose a novel framework for approximating the MPC policy for linear parameter-varying systems using supervised learning. Our learning scheme guarantees feasibility and near-optimality of the approximated MPC policy with high probability. Furthermore, in contrast to most existing approaches that only learn the MPC policy, we also learn the “dual policy,” which enables us to keep a check on the approximated MPC's optimality online during the control process. If the check deems the control input from the approximated MPC policy safe and near-optimal, then it is applied to the plant; otherwise, a backup controller is invoked, thus filtering out (severely) suboptimal control inputs. The backup controller is only invoked with a bounded (low) probability, where the exact probability level can be chosen by the user. Since our framework does not require solving any optimization problem during the control process, it enables the deployment of MPC on resource-constrained systems. Specifically, we illustrate the utility of the proposed framework on a vehicle dynamics control problem. Compared with online optimization methods, we demonstrate a speedup of up to 62× on a desktop computer and 10× on an automotive-grade electronic control unit, while maintaining high control performance.
更多查看译文
关键词
Deep neural networks (DNNs),explicit model predictive control,model predictive control (MPC),policy learning,randomized algorithms,safe learning,sample complexity
AI 理解论文
溯源树
样例
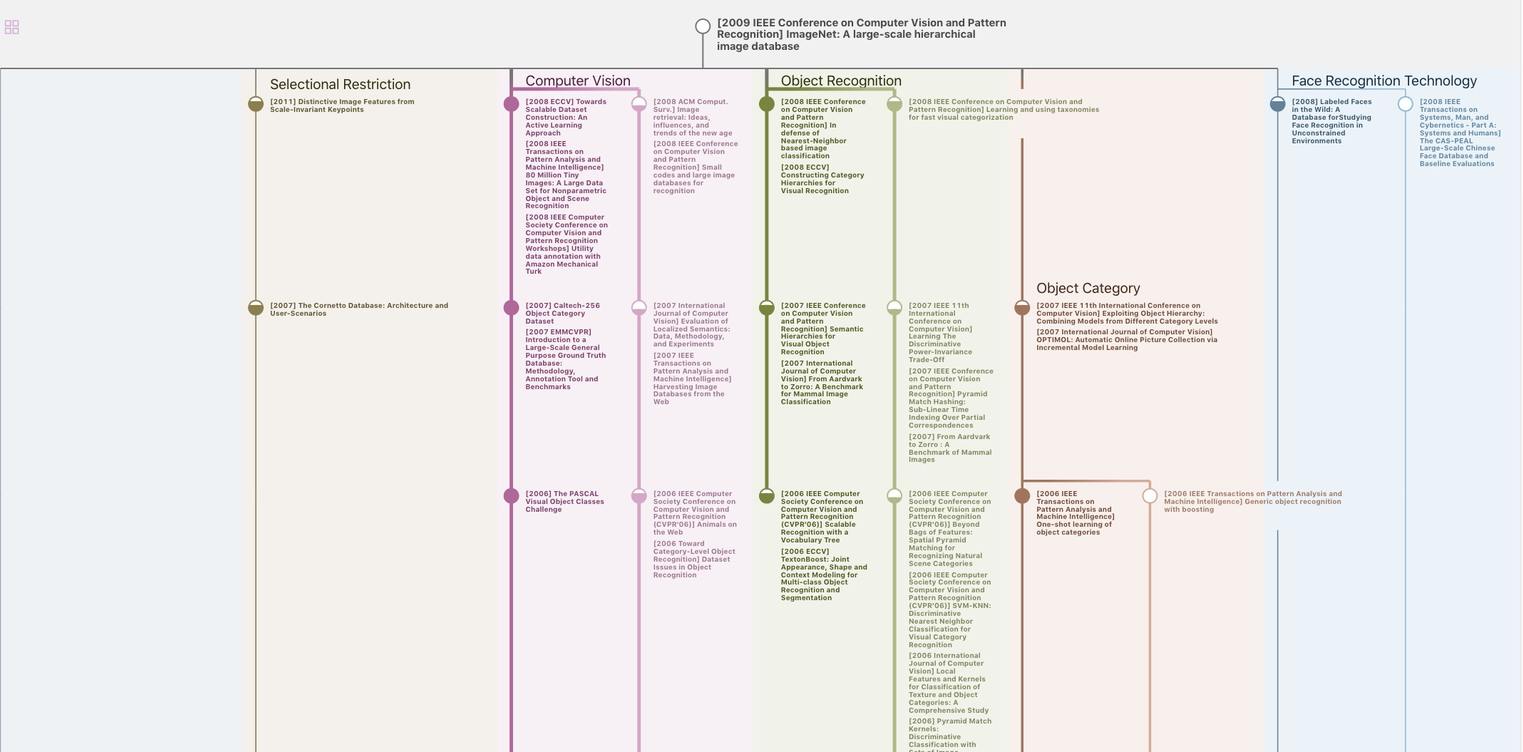
生成溯源树,研究论文发展脉络
Chat Paper
正在生成论文摘要