Exploring Unlabeled Faces For Novel Attribute Discovery
2020 IEEE/CVF CONFERENCE ON COMPUTER VISION AND PATTERN RECOGNITION (CVPR)(2020)
摘要
Despite remarkable success in unpaired image-to-image translation, existing approaches still require a large amount of labeled images. This is a bottleneck against their realworld applications; in practice, a model trained on a labeled dataset, such as CelebA dataset, does not work well for test images from a different distribution - limiting their applications to unlabeled images of a much larger quantity. In this paper; we attempt to alleviate this necessity for labeled data in the facial image translation domain. We aim to explore the degree to which you can discover novel attributes from unlabeled faces and perform high-quality translation. Ib this end, we use prior knowledge about the visual world as guidance to discover novel attributes and transfer them via a novel normalization method. Experiments show that our method trained on unlabeled data produces high-quality translations, preserves identity, and is perceptually realistic, as good as, or better than, state-of-the-art methods trained on labeled data.
更多查看译文
关键词
normalization method,unlabeled data,high-quality translation,facial image translation domain,unlabeled images,test images,labeled CelebA dataset,labeled images,unpaired image-to-image translation,novel attribute discovery,unlabeled faces
AI 理解论文
溯源树
样例
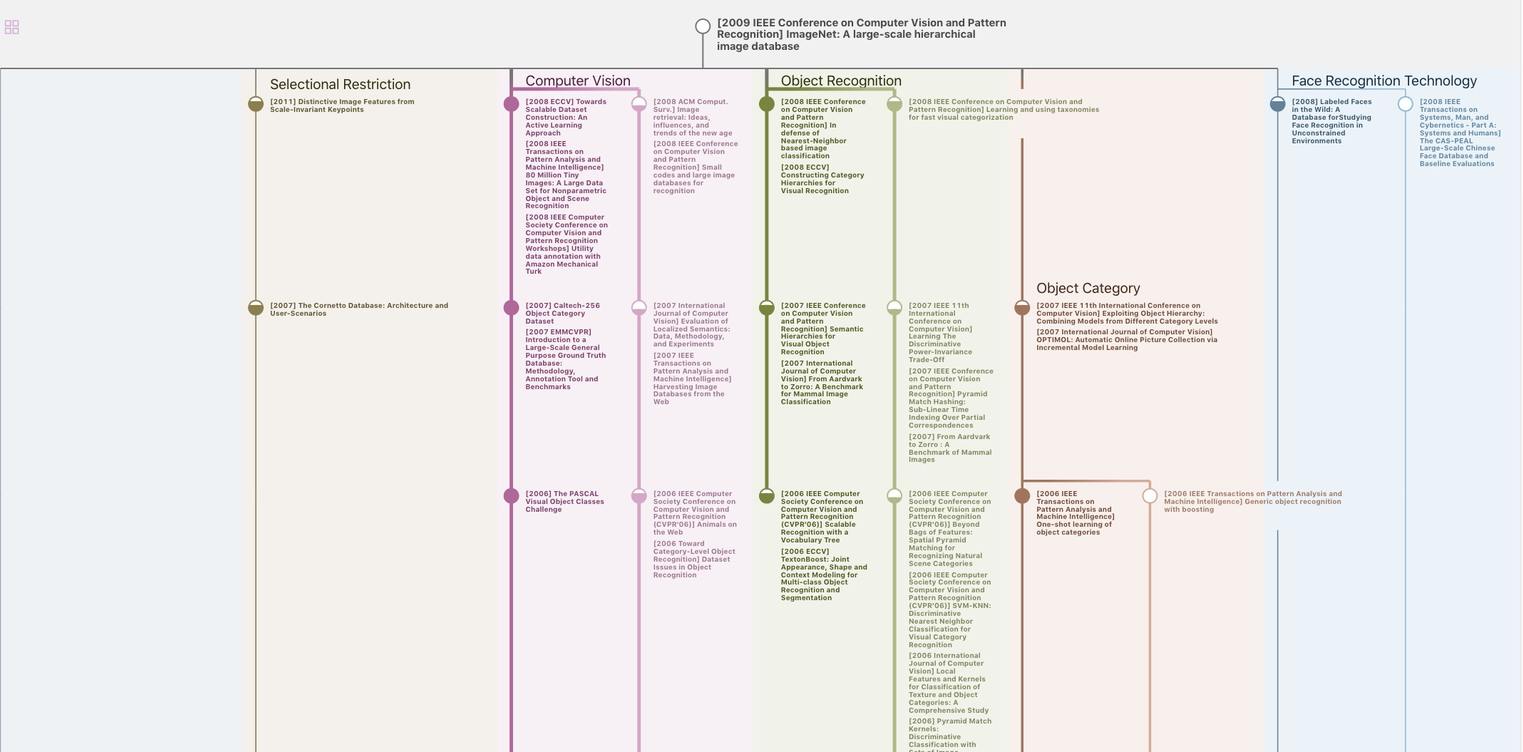
生成溯源树,研究论文发展脉络
Chat Paper
正在生成论文摘要