Self-Attention Temporal Convolutional Network for Long-Term Daily Living Activity Detection
2019 16th IEEE International Conference on Advanced Video and Signal Based Surveillance (AVSS)(2019)
摘要
In this paper, we address the detection of daily living activities in long-term untrimmed videos. The detection of daily living activities is challenging due to their long temporal components, low inter-class variation and high intra-class variation. To tackle these challenges, recent approaches based on Temporal Convolutional Networks (TCNs) have been proposed. Such methods can capture long-term temporal patterns using a hierarchy of temporal convolutional filters, pooling and up sampling steps. However, as one of the important features of convolutional networks, TCNs process a local neighborhood across time which leads to inefficiency in modeling the long-range dependencies between these temporal patterns of the video. In this paper, we propose Self-Attention - Temporal Convolutional Network (SA-TCN), which is able to capture both complex activity patterns and their dependencies within long-term untrimmed videos. We evaluate our proposed model on DAily Home LIfe Activity Dataset (DAHLIA) and Breakfast datasets. Our proposed method achieves state-of-the-art performance on both DAHLIA and Breakfast dataset.
更多查看译文
关键词
long-term temporal patterns,temporal convolutional filters,long-range dependencies,complex activity patterns,self-attention - temporal convolutional network,long-term untrimmed videos,long-term daily living activity detection,low inter-class variation,high intra-class variation,SA-TCN,daily home life activity dataset,DAHLIA,breakfast datasets
AI 理解论文
溯源树
样例
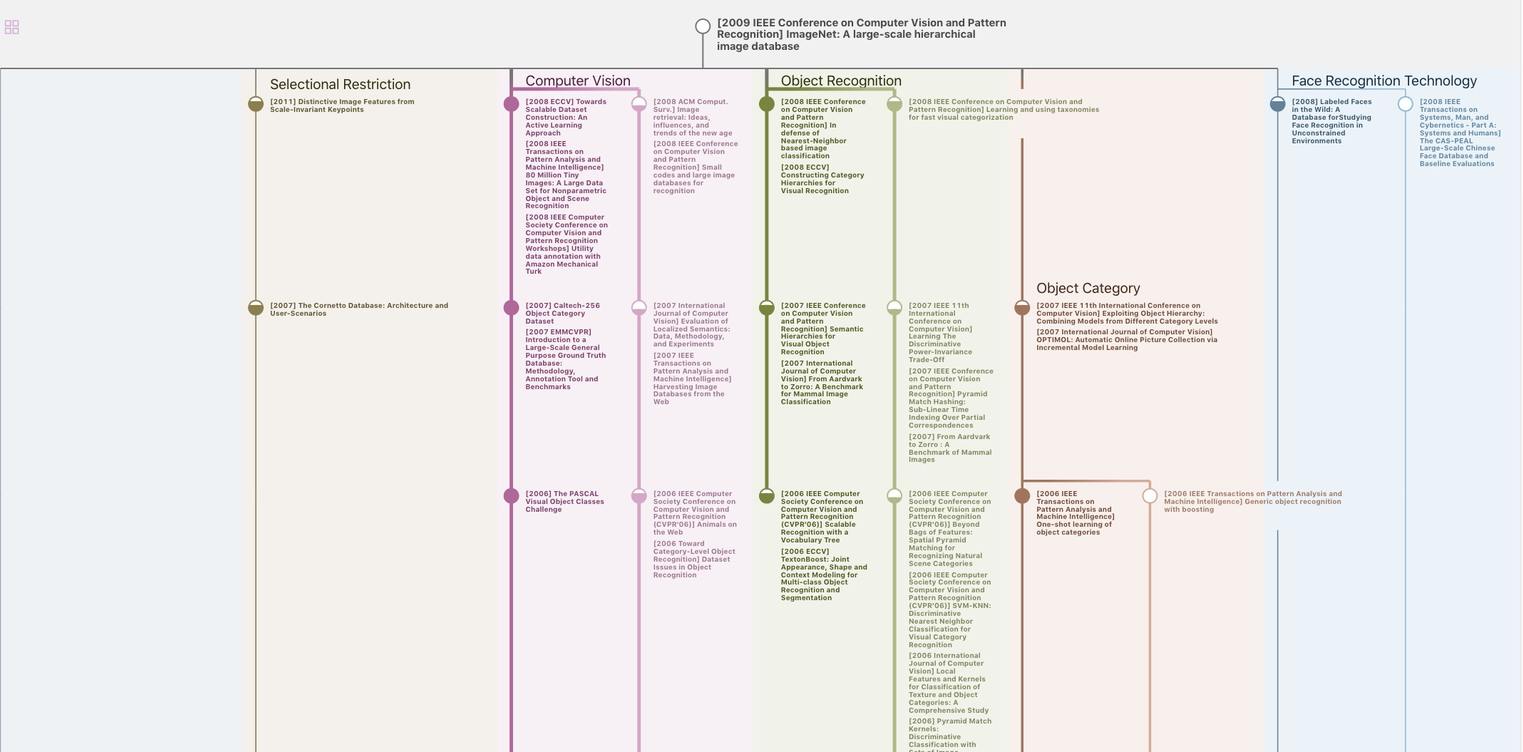
生成溯源树,研究论文发展脉络
Chat Paper
正在生成论文摘要