A Deep Learning-Based End-to-End Composite System for Hand Detection and Gesture Recognition.
SENSORS(2019)
摘要
Recent research on hand detection and gesture recognition has attracted increasing interest due to its broad range of potential applications, such as human-computer interaction, sign language recognition, hand action analysis, driver hand behavior monitoring, and virtual reality. In recent years, several approaches have been proposed with the aim of developing a robust algorithm which functions in complex and cluttered environments. Although several researchers have addressed this challenging problem, a robust system is still elusive. Therefore, we propose a deep learning-based architecture to jointly detect and classify hand gestures. In the proposed architecture, the whole image is passed through a one-stage dense object detector to extract hand regions, which, in turn, pass through a lightweight convolutional neural network (CNN) for hand gesture recognition. To evaluate our approach, we conducted extensive experiments on four publicly available datasets for hand detection, including the Oxford, 5-signers, EgoHands, and Indian classical dance (ICD) datasets, along with two hand gesture datasets with different gesture vocabularies for hand gesture recognition, namely, the LaRED and TinyHands datasets. Here, experimental results demonstrate that the proposed architecture is efficient and robust. In addition, it outperforms other approaches in both the hand detection and gesture classification tasks.
更多查看译文
关键词
hand detection,hand gesture recognition,human-computer interaction,deep learning
AI 理解论文
溯源树
样例
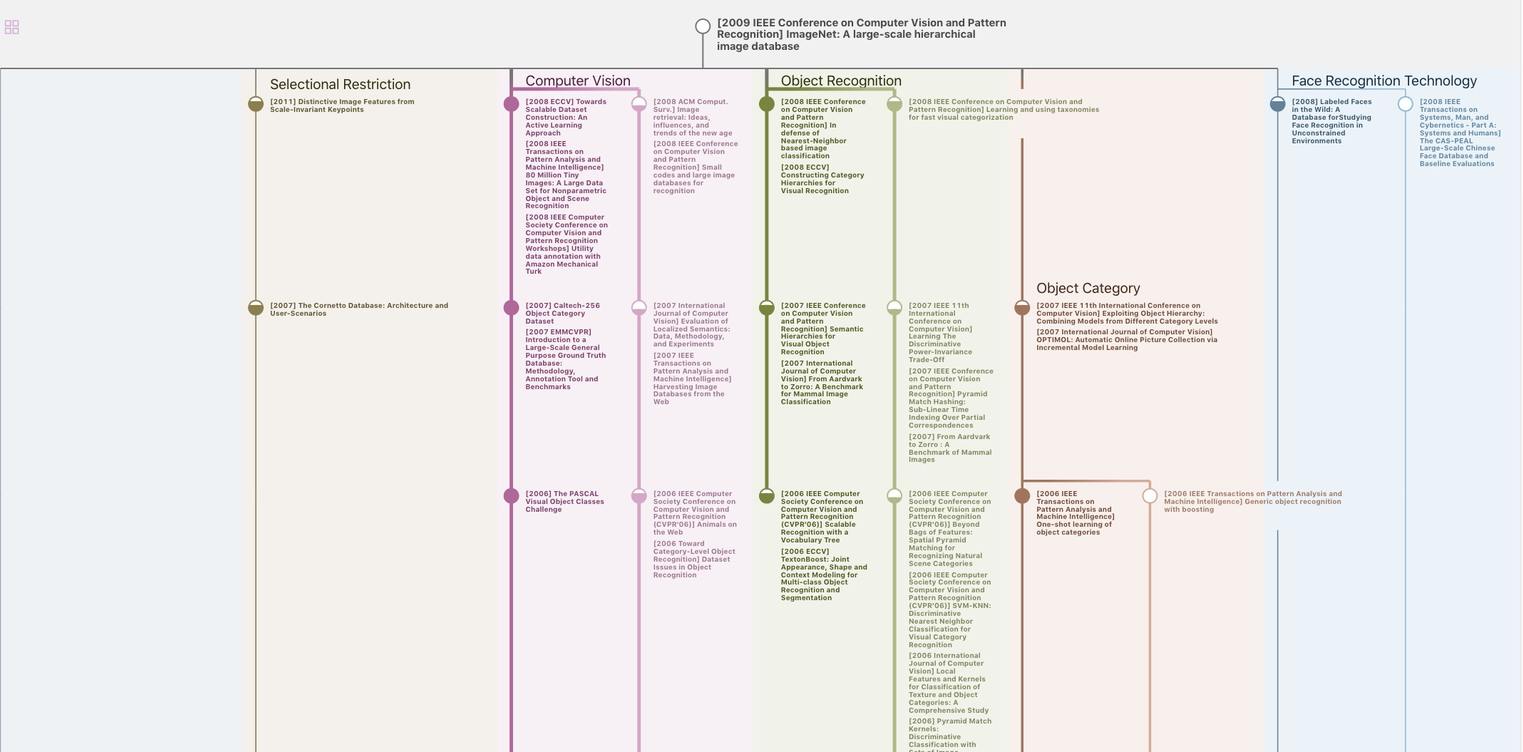
生成溯源树,研究论文发展脉络
Chat Paper
正在生成论文摘要