SEXTANT: A Computational Framework for Scalable and Efficient Correlation of Spatio-Temporal Trajectories
2019 IEEE INTERNATIONAL CONFERENCE ON BIG DATA (BIG DATA)(2019)
摘要
Discovering pairs of related entities based on their spatio-temporal behavior has applications of scientific, commercial, and public interest. For example, inferring relationships between animals based on data collected from tracking devices can help biologists better understand group behavior and family structures; identifying commonalities in customer behavior can enable better targeted marketing; and discovering correlations in the movement patterns of maritime vessels can help crack down on illegal fishing or smuggling activity. However, this task becomes especially challenging when many entities are being observed simultaneously and when observations of different entities are made asynchronously and at different frequencies. We propose SEXTANT, a computational framework for performing spatio-temporal correlation at scale, implemented using efficient data structures over a distributed architecture. SEXTANT's core capability consists of algorithms that, given a large set of time-stamped and geo-located observations, identify pairs of trajectories with high spatial proximity over time, demonstrating shared pattern-of-life behavior. Experiments on real-world and synthetic datasets demonstrate SEXTANT's ability to efficiently capture correlated behavior at scale while being robust to asynchronicity, sparsity, and heterogeneity in the data, out-performing existing methods.
更多查看译文
关键词
correlated behavior,SEXTANT's ability,pattern-of-life behavior,geo-located observations,time-stamped observations,SEXTANT's core capability,data structures,spatio-temporal correlation,illegal fishing,maritime vessels,movement patterns,customer behavior,group behavior,biologists,tracking devices,spatio-temporal behavior,spatio-temporal trajectories,computational framework
AI 理解论文
溯源树
样例
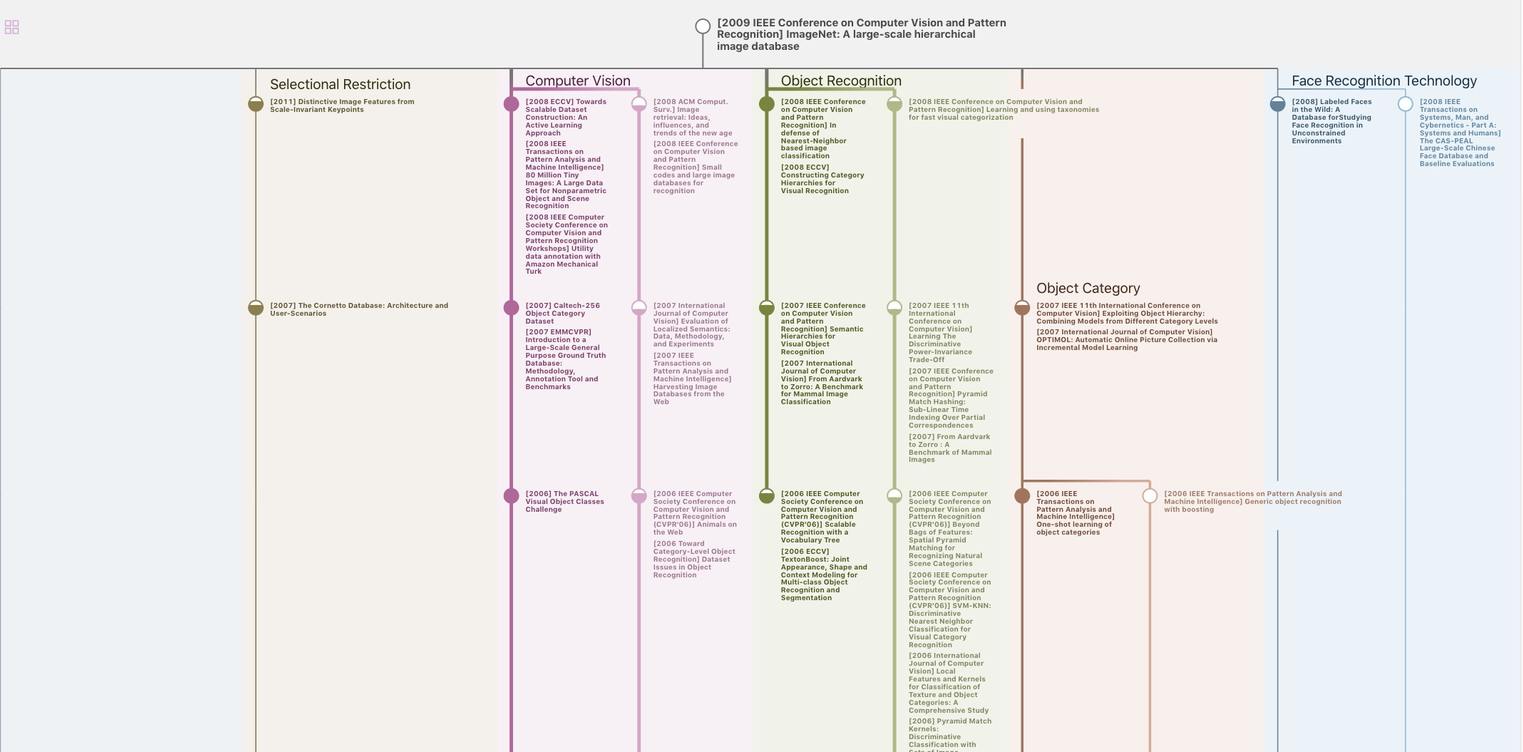
生成溯源树,研究论文发展脉络
Chat Paper
正在生成论文摘要