From Text Classification To Keyphrase Extraction For Short Text
2019 IEEE INTERNATIONAL CONFERENCE ON BIG DATA (BIG DATA)(2019)
摘要
Existing keyphrase extraction approaches often suffer from issues such as the sparsity and brevity of short text (e.g., headlines, queries, and tweets). In this paper, we propose a novel keyphrase extraction method for short text by utilizing recurrent neural networks. The main idea behind our approach is to classify short text into a relevant class or category and extract keyphrases from important words in the class or category. Unlike previous supervised approaches that need the information of annotated keyphrases, our approach requires only a text classification dataset (i.e., DBpedia), which is easier to use and requires less human effort. In our approach, we first feed short text into the attention-based neural network for text classification. We then compute attention weights of each word in input short text. Subsequently, we detect keyphrase candidates by chunking phrases and summing the attention weights of compositional words in the chunked phrase. The experimental results clearly show the efficacy of our approach on real-world datasets, such as headlines, queries, and tweets. The proposed method outperforms the Microsoft Cognitive Services and IBM Watson Natural Language Understanding service for keyphrase extraction in terms of F1-score and acceptable percentage on the NYT and Question datasets. Further, we confirm that the proposed method is comparable to supervised methods for keyphrase extraction from short text in the Tweet dataset.
更多查看译文
关键词
Keyphrase extraction, Text classification, Attention mechanism, Deep neural network, Knowledge base
AI 理解论文
溯源树
样例
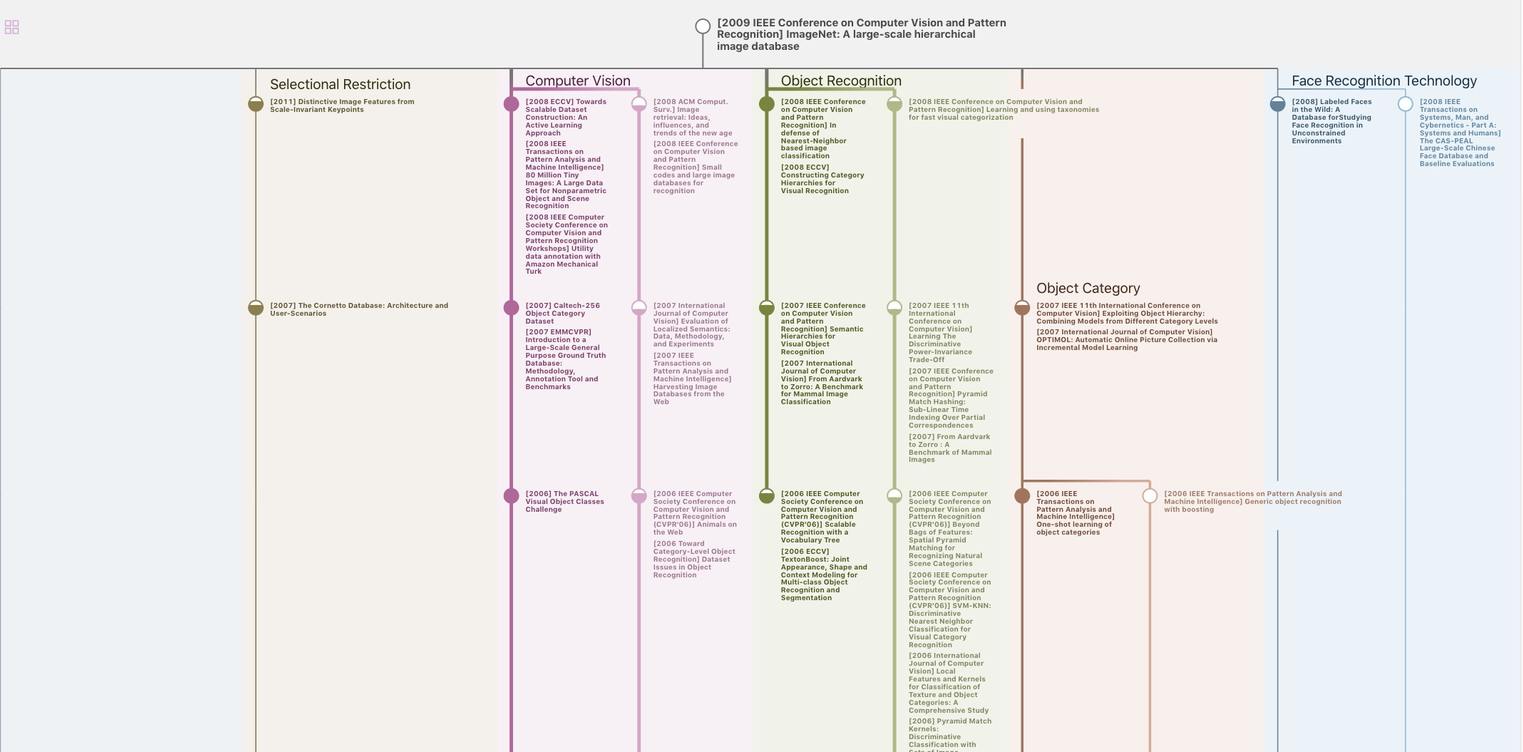
生成溯源树,研究论文发展脉络
Chat Paper
正在生成论文摘要