Proximal Splitting Algorithms for Convex Optimization: A Tour of Recent Advances, with New Twists
arxiv(2023)
摘要
Convex nonsmooth optimization problems, whose solutions live in very high dimensional spaces, have become ubiquitous. To solve them, the class of first-order algorithms known as proximal splitting algorithms is particularly adequate: they consist of simple operations, handling the terms in the objective function separately. In this overview, we demystify a selection of recent proximal splitting algorithms: we present them within a unified framework, which consists in applying splitting methods for monotone inclusions in primal-dual product spaces, with well-chosen metrics. Along the way, we easily derive new variants of the algorithms and revisit existing convergence results, extending the parameter ranges in several cases. In particular, we emphasize that when the smooth term in the objective function is quadratic, e.g., for least-squares problems, convergence is guaranteed with larger values of the relaxation parameter than previously known. Such larger values are usually beneficial for the convergence speed in practice.
更多查看译文
关键词
proximal splitting algorithms,convex optimization
AI 理解论文
溯源树
样例
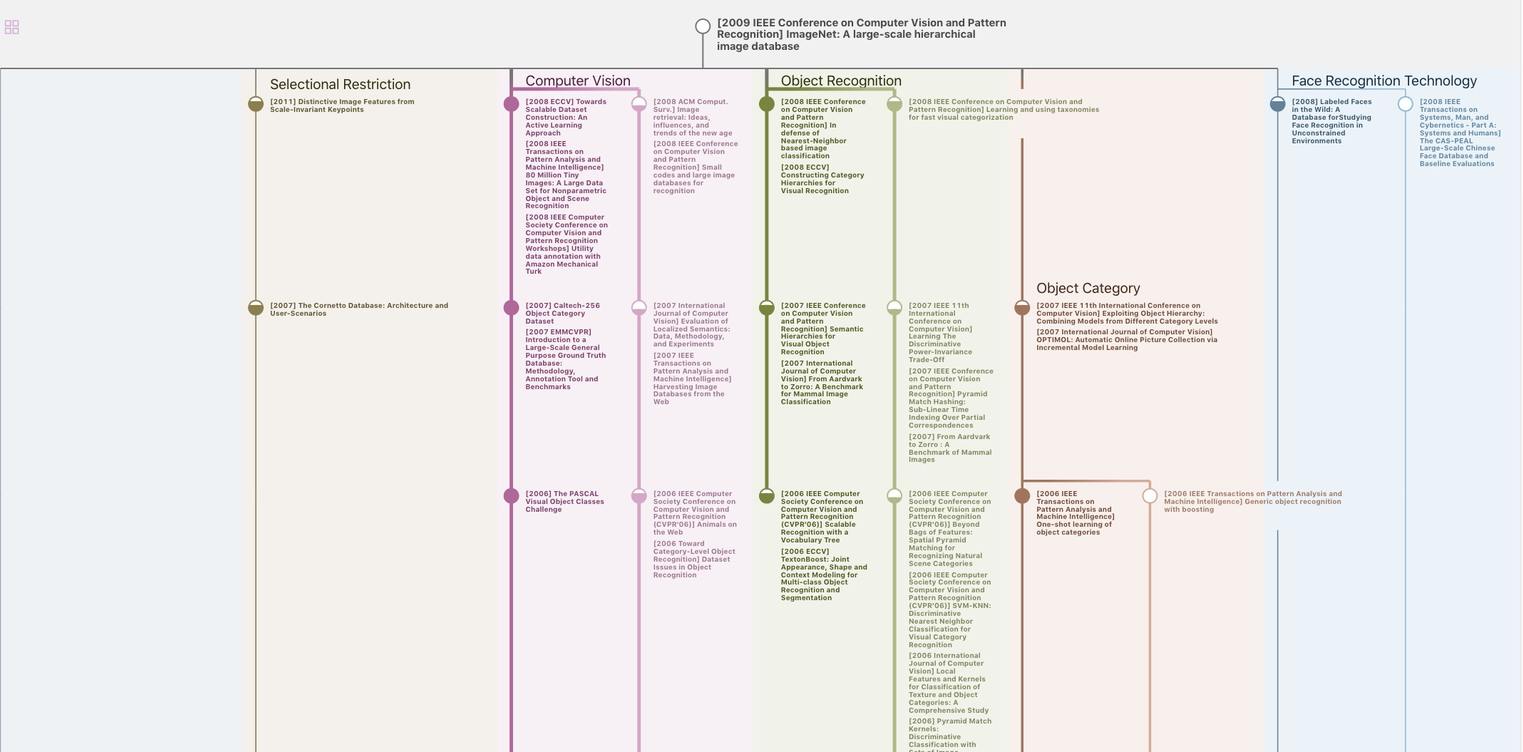
生成溯源树,研究论文发展脉络
Chat Paper
正在生成论文摘要