Two-Stage Learning For Uplink Channel Estimation In One-Bit Massive Mimo
CONFERENCE RECORD OF THE 2019 FIFTY-THIRD ASILOMAR CONFERENCE ON SIGNALS, SYSTEMS & COMPUTERS(2019)
摘要
We develop a two-stage deep learning pipeline architecture to estimate the uplink massive MIMO channel with one-bit ADCs. This deep learning pipeline is composed of two separate generative deep learning models. The first one is a supervised learning model and designed to compensate for the quantization loss. The second one is an unsupervised learning model and optimized for denoising. Our results show that the proposed deep learning-based channel estimator can significantly outperform other state-of-the-art channel estimators for one-bit quantized massive MIMO systems. In particular, our design provides 5-10 dB gain in channel estimation error. Furthermore, it requires a reasonable amount of pilots, on the order of 20 per coherence time interval.
更多查看译文
关键词
two-stage learning,uplink channel estimation,one-bit massive MIMO,deep learning pipeline architecture,uplink massive MIMO channel,one-bit ADC,generative deep learning models,supervised learning model,quantization loss,deep learning-based channel estimator,one-bit quantized massive MIMO systems,channel estimation error,gain 5.0 dB to 10.0 dB
AI 理解论文
溯源树
样例
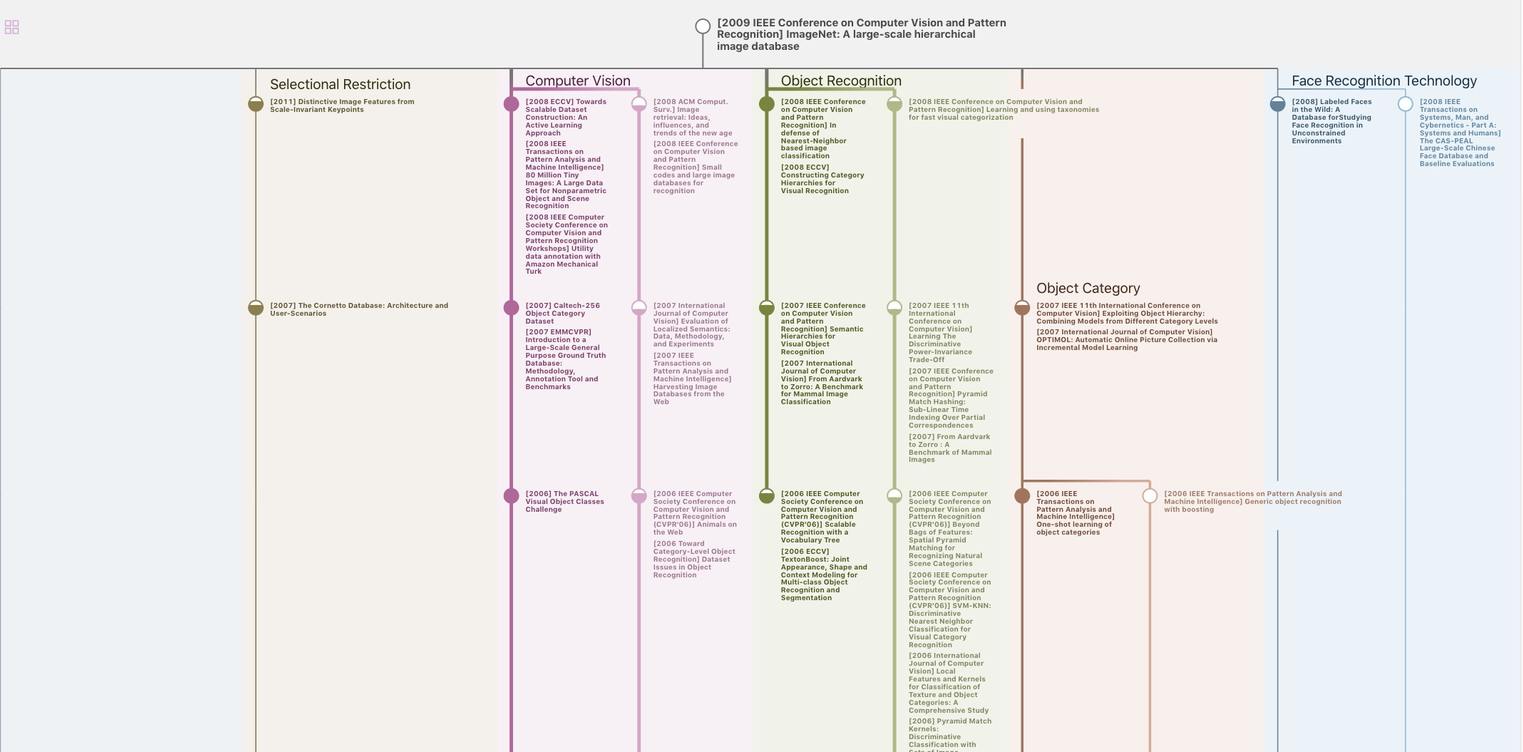
生成溯源树,研究论文发展脉络
Chat Paper
正在生成论文摘要