PREDICT & CLUSTER: Unsupervised Skeleton Based Action Recognition
CVPR(2020)
摘要
We propose a novel system for unsupervised skeleton-based action recognition. Given inputs of body-keypoints sequences obtained during various movements, our system associates the sequences with actions. Our system is based on an encoder-decoder recurrent neural network, where the encoder learns a separable feature representation within its hidden states formed by training the model to perform the prediction task. We show that according to such unsupervised training, the decoder and the encoder self-organize their hidden states into a feature space which clusters similar movements into the same cluster and distinct movements into distant clusters. Current state-of-the-art methods for action recognition are strongly supervised, i.e., rely on providing labels for training. Unsupervised methods have been proposed, however, they require camera and depth inputs (RGB+D) at each time step. In contrast, our system is fully unsupervised, does not require action labels at any stage and can operate with body-keypoints input only. Furthermore, the method can perform on various dimensions of body-keypoints (2D or 3D) and can include additional cues describing movements. We evaluate our system on three action recognition benchmarks with different numbers of actions and examples. Our results outperform prior unsupervised skeleton-based methods, unsupervised RGB+D based methods on cross-view tests and while being unsupervised have similar performance to supervised skeleton-based action recognition.
更多查看译文
关键词
camera,unsupervised skeleton-based action recognition benchmarks,unsupervised RGB+D based methods,body-keypoints input,self-organize their hidden states,unsupervised training,encoder-decoder recurrent neural network,body-keypoints sequences,PREDICT & CLUSTER,supervised skeleton-based action recognition
AI 理解论文
溯源树
样例
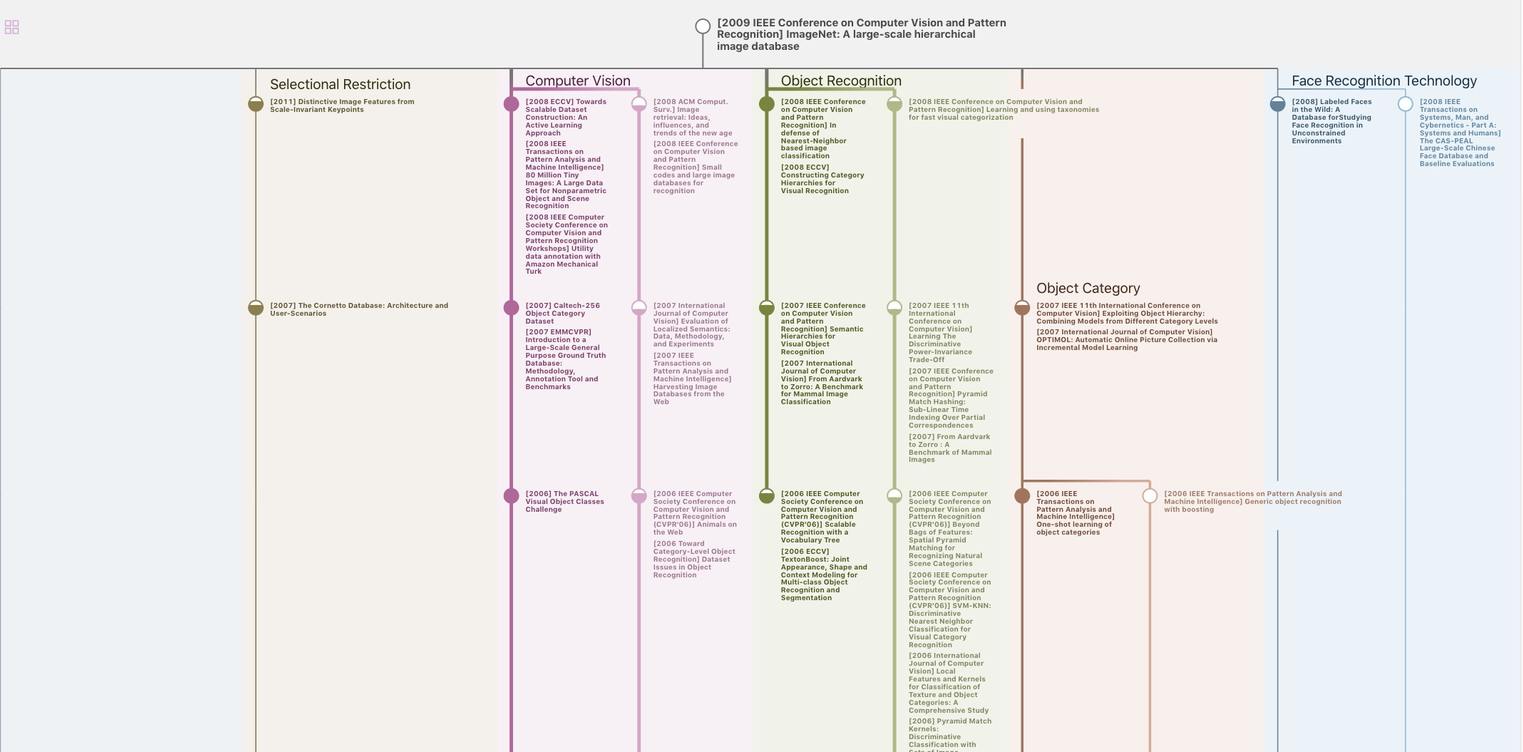
生成溯源树,研究论文发展脉络
Chat Paper
正在生成论文摘要