Locality sensitive discriminant projection for feature extraction and face recognition.
JOURNAL OF ELECTRONIC IMAGING(2019)
摘要
As an effective feature extraction method, locality sensitive discriminant analysis (LSDA) utilizes the neighbor relationship of data to characterize the manifold structure of data and uses label information of data to adapt to classification tasks. However, the performance of LSDA is affected by outliers and the destruction of local structure. Aiming at solving the limitations of LSDA, a locality sensitive discriminant projection (LSDP) algorithm is proposed. LSDP minimizes the distance of intraclass neighbor samples to maintain local structure and minimizes the intraclass non-neighbor samples to increase the compactness of intraclass samples after projection. The problem of outliers is alleviated by increasing the compactness of intraclass samples in subspace. At the same time, we redefine the weights of interclass neighbor samples to maintain the neighbor relationship of different labels samples. Holding the local structure of interclass samples maintains the manifold structure of data. Experiments on face datasets demonstrate the effectiveness of the LSDP algorithm. (C) 2019 SPIE and IS&T
更多查看译文
关键词
feature extraction,locality sensitive discriminant projection,outliers,manifold learning
AI 理解论文
溯源树
样例
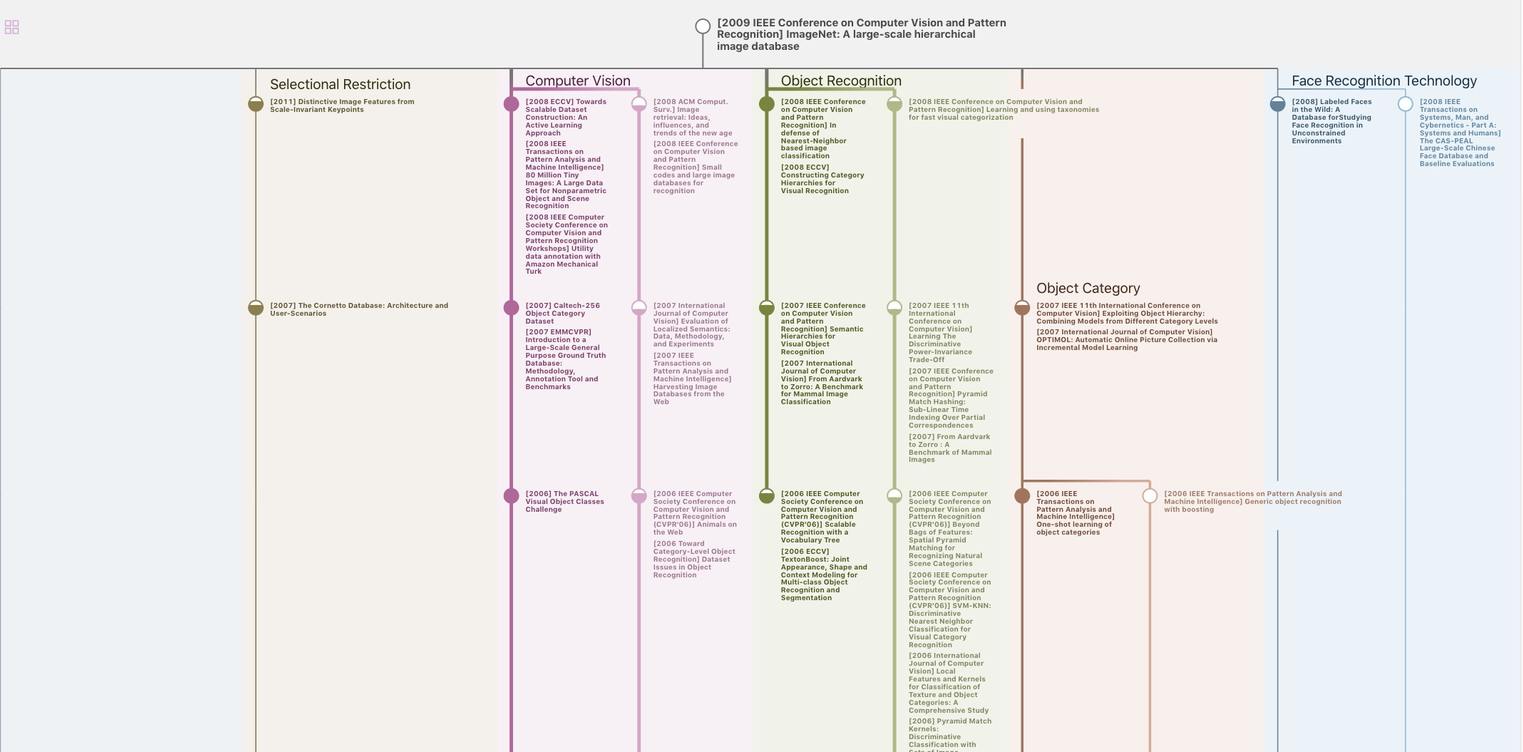
生成溯源树,研究论文发展脉络
Chat Paper
正在生成论文摘要