Cross-layer fusion feature network for material defect detection.
JOURNAL OF ELECTRONIC IMAGING(2019)
摘要
Object detection involves solving two main problems: identifying the object and its location. This transforms the problem into a classification and localization problem. Currently, research shows that a convolutional neural network (CNN) can be used to solve the classification problem, and the object detection module incorporating a region proposal method can be used to locate the object. Although a CNN based on region proposals can achieve high recall, which improves detection accuracy, its performance cannot meet the actual requirements for small-size object detection and precise localization. This is mainly due to the feature maps extracted from the CNN and the quality of the region proposals. We present a cross-layer fusion feature network (CLFF-Net) for both high-quality region proposal generation and accurate object detection. The CLFF-Net is based on the cross-layer fusion feature that extracts hierarchical feature maps and then aggregates them into a unified space. The fused feature map appropriately combines deep layer semantic information, middle layer supplemental information, and shallow layer location information for an image to build the CLFF-Net, which is shared for both generating region proposals and detecting objects via end-to-end training. Extensive experiments using a casting dataset demonstrate its promising performance compared to state-of-the-art approaches. (C) 2019 SPIE and IS&T
更多查看译文
关键词
convolutional neural network,object detection,material defect detection
AI 理解论文
溯源树
样例
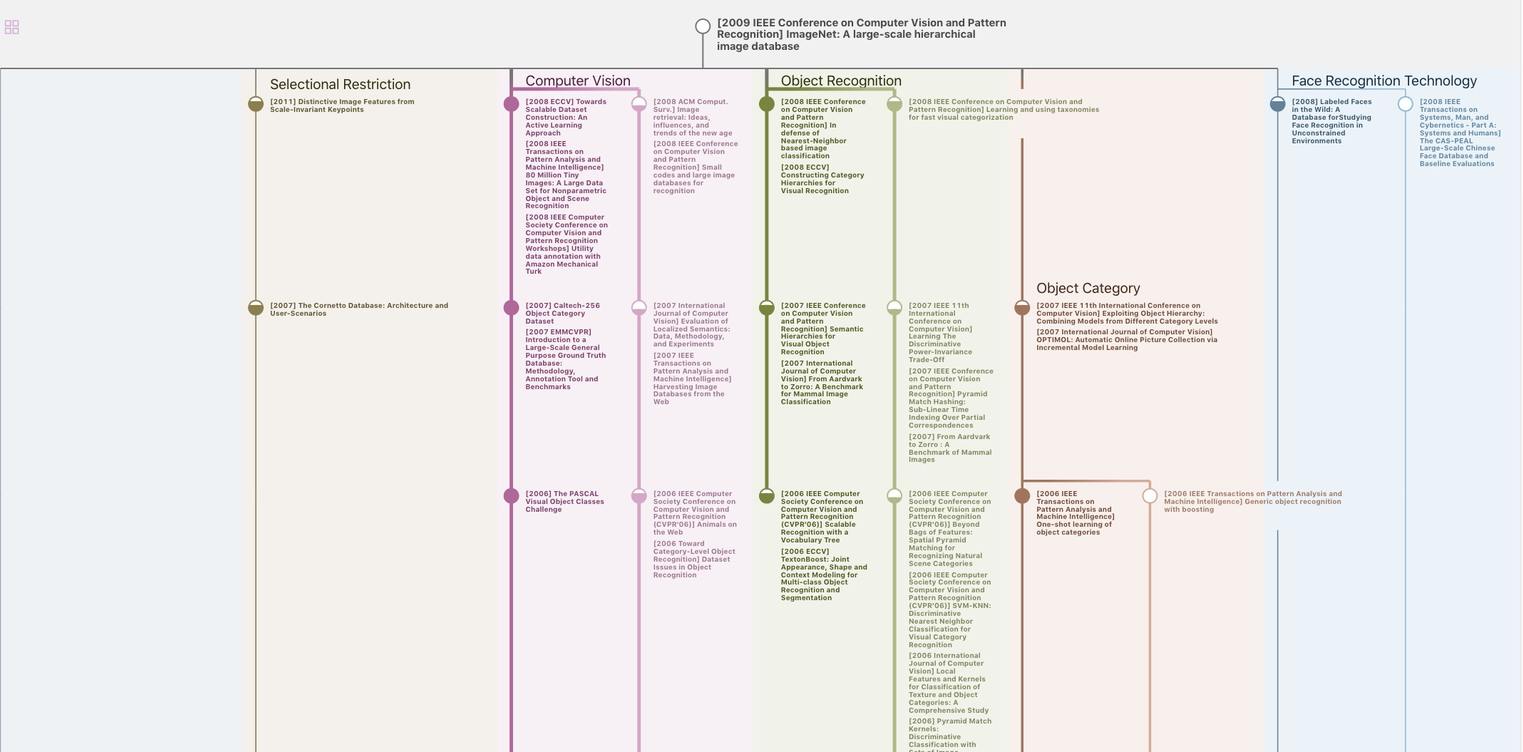
生成溯源树,研究论文发展脉络
Chat Paper
正在生成论文摘要