1018. Using prediction modeling to inform risk-adjustment strategy for hospital antimicrobial use: Can we predict who gets an inpatient antimicrobial?
Open Forum Infectious Diseases(2019)
摘要
Abstract Background Hospital antimicrobial stewardship program (ASP) assessments based on comparisons of antimicrobial use (AU) among multiple hospitals are difficult to interpret without risk-adjustment for patient case-mix. We aimed to determine whether variables of varying complexity, derived retrospectively from the electronic health record (EHR), were predictive of inpatient antimicrobial exposures. Methods We performed a retrospective study of EHR-derived data from adult and pediatric inpatients within the Duke University Health System from October 2015 to September 2017. We used Random Forests machine learning models on two antimicrobial exposure outcomes at the encounter level: binary (ever/never) exposure and days of therapy (DOT). Antimicrobial groups were defined by the NHSN AU Option 2017 baseline. Analyses were stratified by pediatric/adult, location type (ICU/ward), and antimicrobial group. Candidate variables were categorized into four tiers based on feasibility of measurement from the EHR. Tier 1 (easy) included demographics, season, location, while Tier 4 (hard) included all variables from Tier 1–3 and laboratory results, vital signs, and culture data. Data were split into 80/20 training and testing sets to measure model performance using area under the curve (AUC) for the binary outcomes and absolute error for DOT. Results The analysis dataset included 170,294 encounters and 204 candidate variables from three hospitals. A total of 80,190 (47%) encounters had antimicrobial exposure; 64,998 (38%) had 1–6 DOT, and 15,192 (9%) had 7 or greater DOT. Models strongly predicted the binary outcome, with AUCs ranging from 0.70 to 0.95 depending on the stratum (Figure A, B). The addition of more complex variables increased accuracy (Figure Model Tiers 1–4). Model performance varied based on location and antimicrobial group. Models for infrequently used groups performed better (Figure C, D). Models underestimated DOTs of encounters with extremely long lengths of stay. Conclusion Models utilizing EHR-derived variables strongly predicted antimicrobial exposure. Risk-adjustment strategies incorporating measures of patient mix may provide more informative benchmark comparisons for use in Antimicrobial Stewardship Program assessments. Disclosures All authors: No reported disclosures.
更多查看译文
AI 理解论文
溯源树
样例
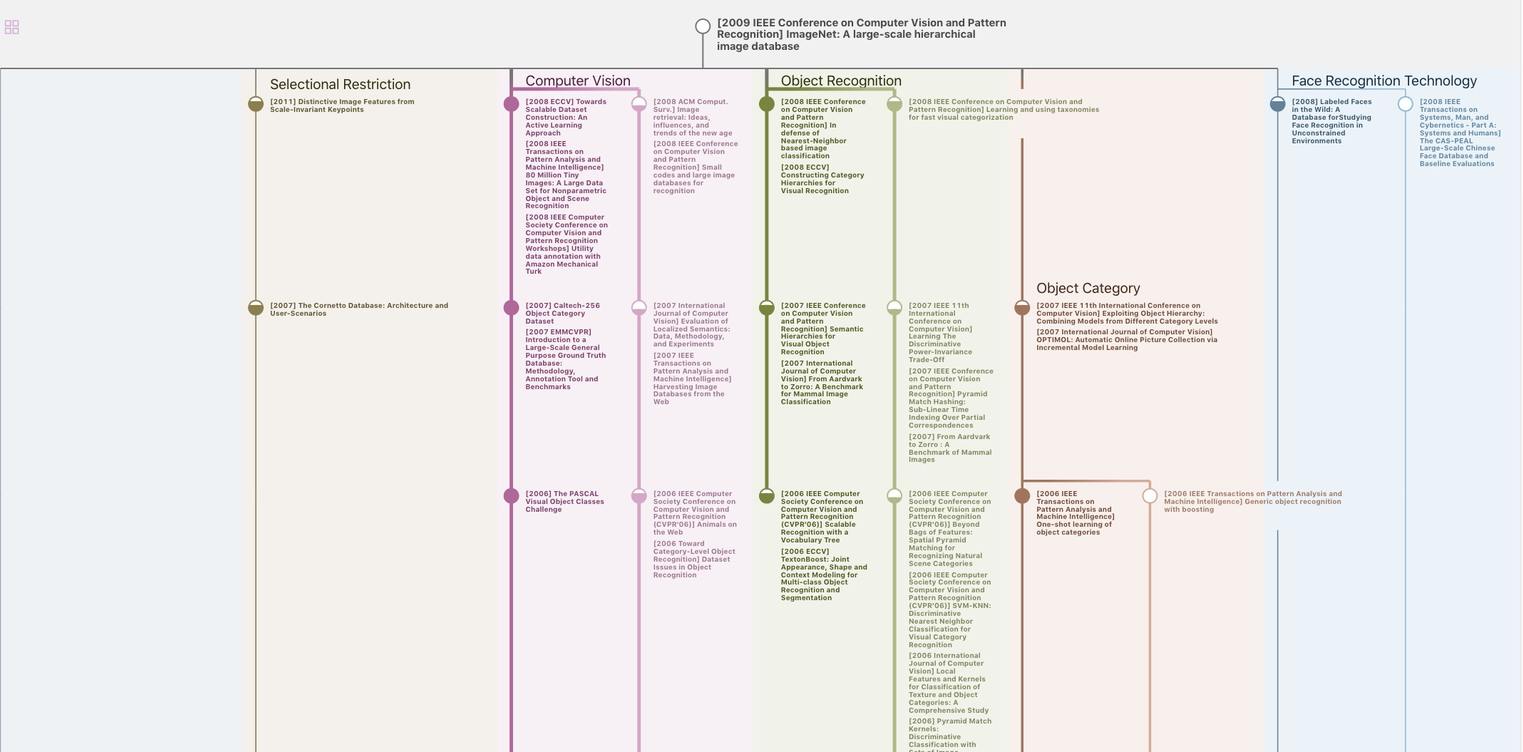
生成溯源树,研究论文发展脉络
Chat Paper
正在生成论文摘要