No Radio Left Behind: Radio Fingerprinting Through Deep Learning of Physical-Layer Hardware Impairments
IEEE Transactions on Cognitive Communications and Networking(2020)
摘要
Due to the unprecedented scale of the Internet of Things, designing scalable, accurate, energy-efficient and tamper-proof authentication mechanisms has now become more important than ever. To this end, in this paper we present ORACLE, a novel system based on convolutional neural networks (CNNs) to “fingerprint” (i.e., identify) a unique radio from a large pool of devices by deep-learning the fine-grained hardware impairments imposed by radio circuitry on physical-layer I/Q samples. First, we show how hardware-specific imperfections are learned by the CNN framework. Then, we extensively evaluate the performance of ORACLE on several first-of-its-kind large-scale datasets of WiFi-transmissions collected “in the wild”, as well as a dataset of nominally-identical (i.e., equal baseband signals) WiFi devices, reaching 80-90% accuracy is many cases with the error gap arising due to channel-induced effects. Finally, we show through an experimental testbed, how this accuracy can reach over 99% by intentionally inserting and learning the effect of controlled impairments at the transmitter side, to completely remove the impact of the wireless channel. Furthermore, to scale this approach for classifying potential thousands of radios, we propose an impairment hopping spread spectrum (IHOP) technique that is resilient to spoofing attacks.
更多查看译文
关键词
Radio transmitters,Radio frequency,Hardware,Wireless communication,Feature extraction,Deep learning,Wireless fidelity
AI 理解论文
溯源树
样例
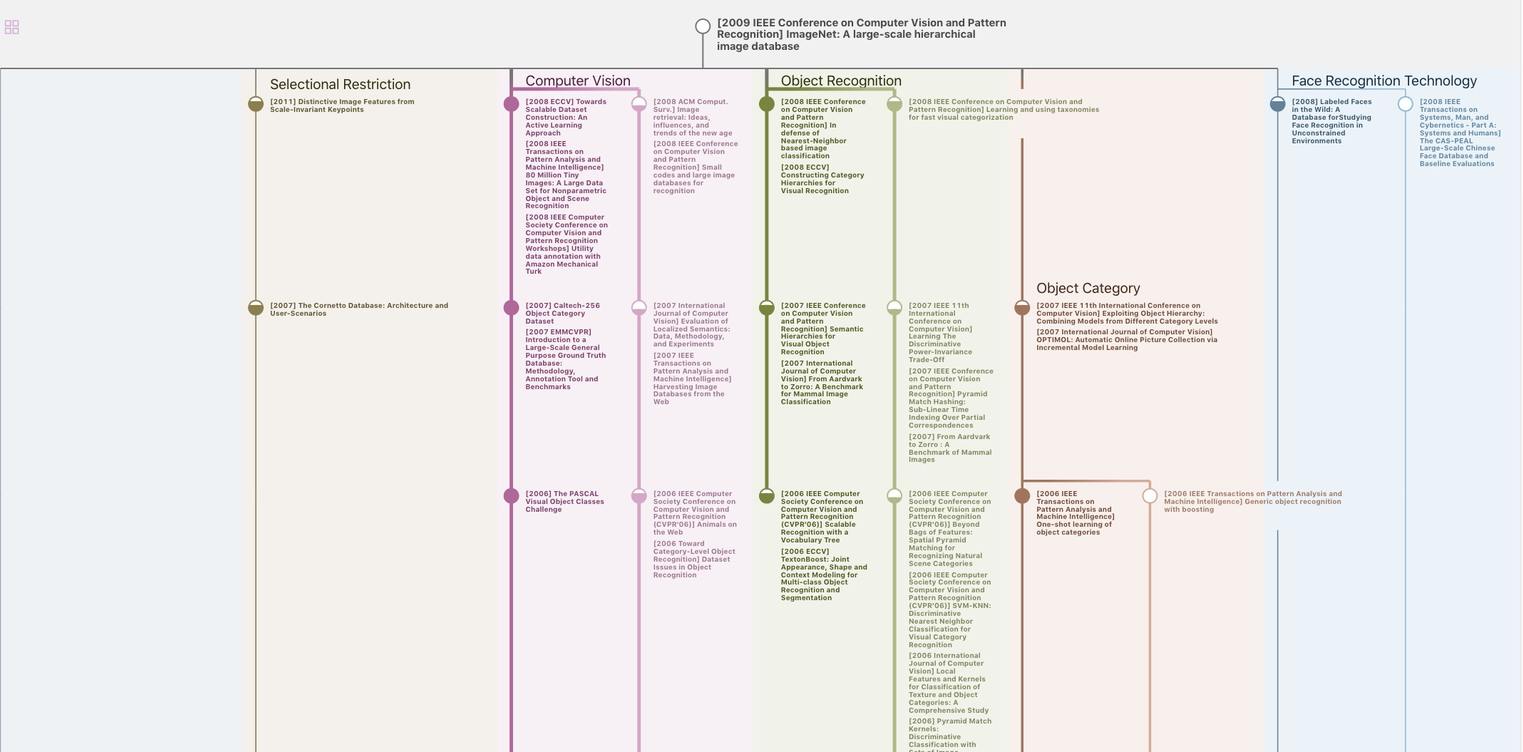
生成溯源树,研究论文发展脉络
Chat Paper
正在生成论文摘要