A Machine Learning Model to Characterize Chronic Kidney Disease with Metabolomics Data
2019 IEEE International Conference on Healthcare Informatics (ICHI)(2019)
摘要
Plasma metabolomics analyses quantify hundreds to thousands of metabolites present in patient plasma samples, serving as proxies for physiological homeostasis and gene- environment interactions. We performed a retrospective cohort study on patients with diverse Chronic kidney disease (CKD) stages, with the aim to investigate the power of metabolomic biomarker model for diagnosis and prediction of disease progression. We built an elastic-net model (ENM) which consists fifteen metabolomic biomarker and serum biomarkers. The model was sensitive in classifying patients into G1 to G4 CKD stages, provided an accuracy up to 0.93. This model was also capable of identifying patients with fast disease progression from those with good prognosis. The hazard ratio for time-to-progression between the two groups was 3.22 (95% CI, 2.34 - 4.87; $\mathrm{p}\lt 0.0001)$, after adjustment for age, sex and baseline CKD stage.
更多查看译文
关键词
chronic kidney disease,metabolomics,elastic-net model,machine learning,prognosis biomarker
AI 理解论文
溯源树
样例
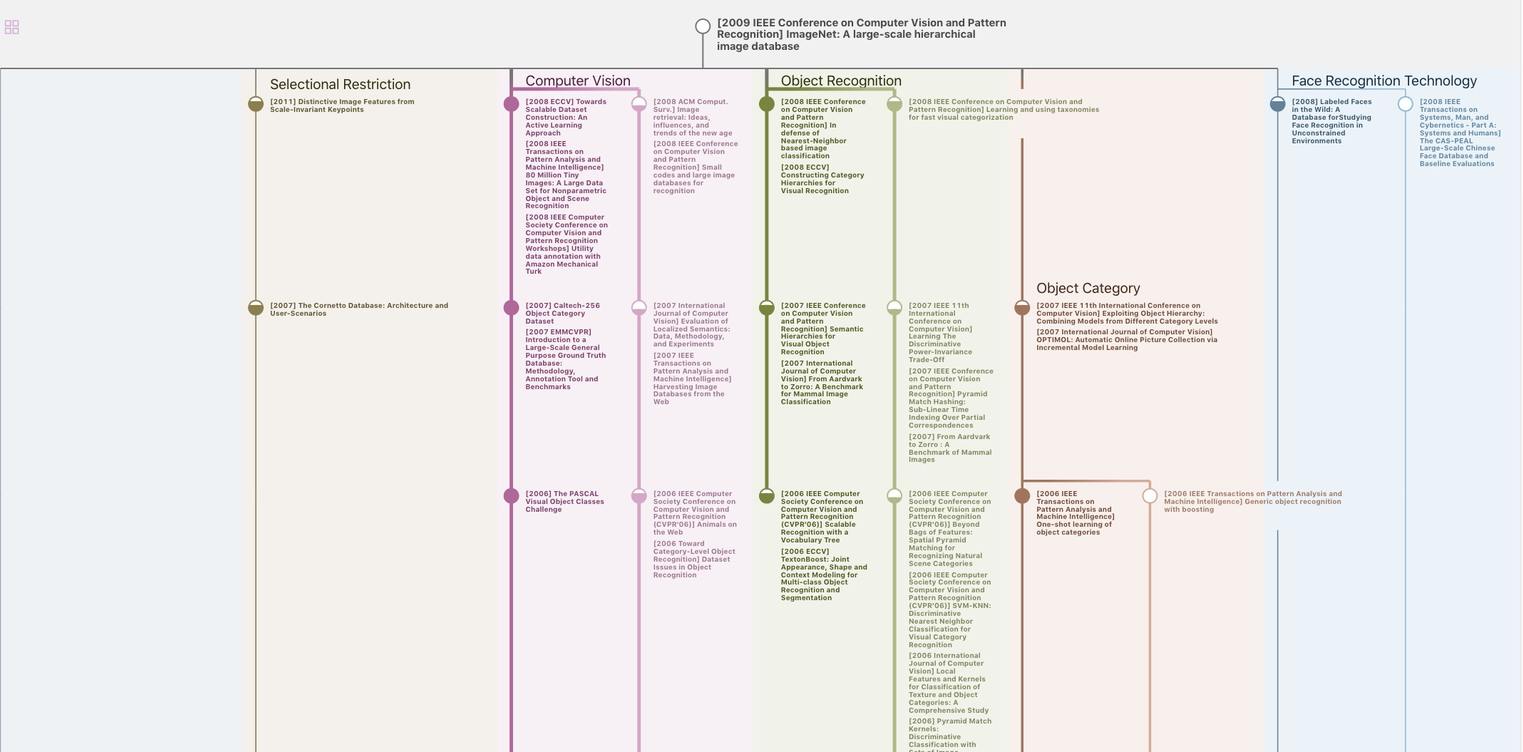
生成溯源树,研究论文发展脉络
Chat Paper
正在生成论文摘要