CSAN: A neural network benchmark model for crime forecasting in spatio-temporal scale
Knowledge-Based Systems(2020)
摘要
Understanding the evolving discipline of crime situations is a long-standing but significant problem. Former methods prefer the stochastic modeling of the crime phenomenon in physics or statistical equations, which are elegant in theoretical explanations but less efficient in real applications. Recently, some data-driven models, especially neural network models, are illustrating promising performance in capturing dynamics of the complex phenomenon, and available massive dataset enables the task-beneficial information utilization. However, there exist several difficulties in regional crime situation awareness, including the high dimensionality, the intractable correlations as well as information redundancies in spatio-temporal dataset. To achieve efficient information processing and disentangle relationships from a recent crime dataset of fifteen years, we construct the crime situation awareness network (CSAN) as a new benchmark forecasting model via integrating structures of variational auto-encoders and context-based sequence generative neural network. Final experiments demonstrate that CSAN mostly outperforms other commonly-used spatio-temporal forecasting algorithms, such as Conv-LSTM, in regional multi-type crime frequency prediction.
更多查看译文
关键词
Crime situation awareness,Crime forecasting,Variational auto-encoder,Sequence generation,Spatio-temporal analysis
AI 理解论文
溯源树
样例
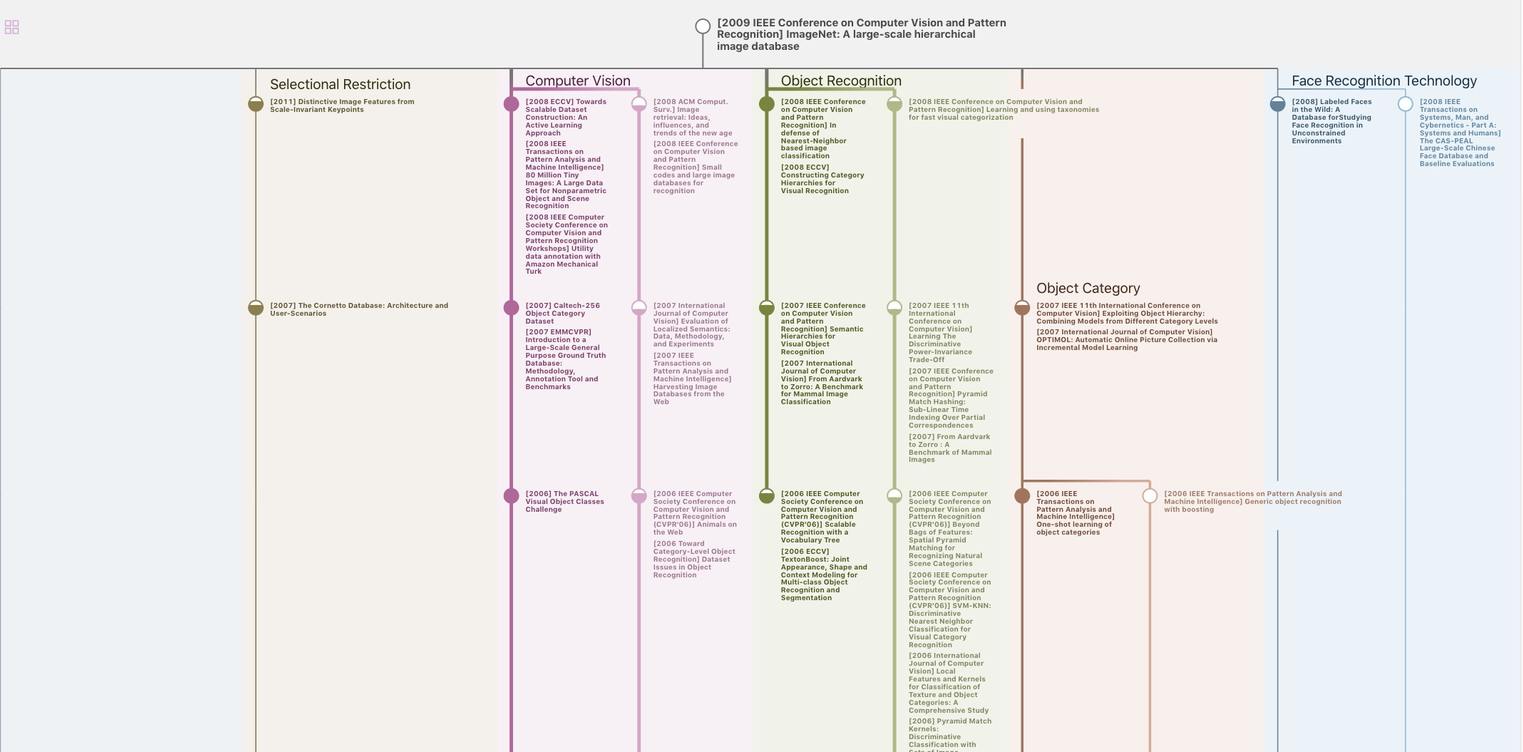
生成溯源树,研究论文发展脉络
Chat Paper
正在生成论文摘要