Reinforcement Learning Based Downlink Interference Control for Ultra-Dense Small Cells
IEEE Transactions on Wireless Communications(2020)
摘要
The dense deployment of small cells in 5G cellular networks raises the issue of controlling downlink inter-cell interference under time-varying channel states. In this paper, we propose a reinforcement learning based power control scheme to suppress downlink inter-cell interference and save energy for ultra-dense small cells. This scheme enables base stations to schedule the downlink transmit power without knowing the interference distribution and the channel states of the neighboring small cells. A deep reinforcement learning based interference control algorithm is designed to further accelerate learning for ultra-dense small cells with a large number of active users. Analytical convergence performance bounds including throughput, energy consumption, inter-cell interference, and the utility of base stations are provided and the computational complexity of our proposed scheme is discussed. Simulation results show that this scheme optimizes the downlink interference control performance after sufficient power control instances and significantly increases the network throughput with less energy consumption compared with a benchmark scheme.
更多查看译文
关键词
Power control,Downlink,Throughput,Microcell networks,Signal to noise ratio
AI 理解论文
溯源树
样例
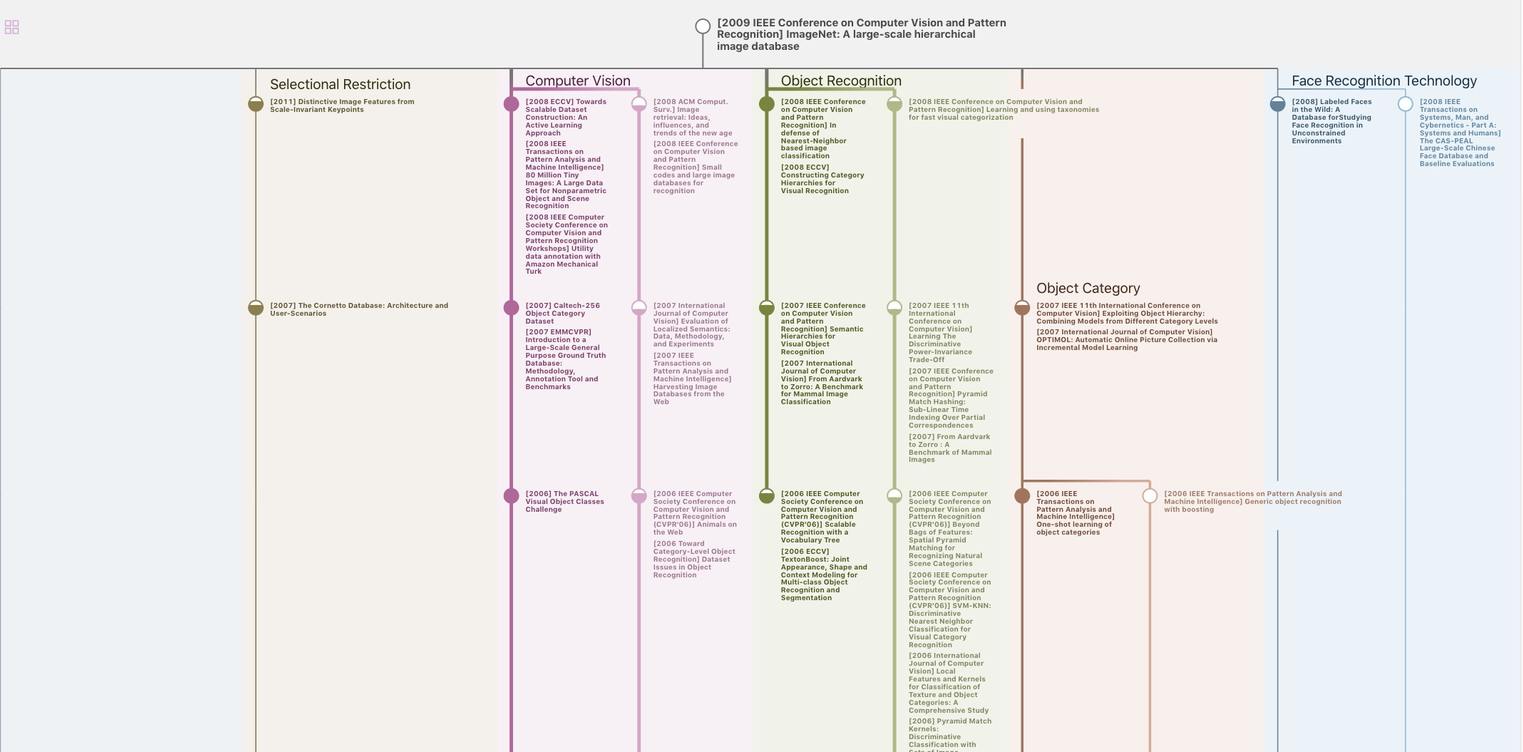
生成溯源树,研究论文发展脉络
Chat Paper
正在生成论文摘要