Multicomponent AM-FM signal analysis based on sparse approximation
Iet Signal Processing(2020)
摘要
The authors propose in this work to formulate multicomponent amplitude modulation (AM)–frequency modulation (FM) representations as a sparse approximation (SA) problem, which is solved using orthogonal matching pursuits (OMP) and least angle regression (LARS). The approach appears to be an efficient tool to separate the frequency content of linear and non-linear signals and behaves in a better way like the well-known data-driven empirical mode decomposition (EMD). Contrary to related works where AM–FM models and/or EMD are used to improve sparsity or learned dictionaries, they propose instead a sparse approach framework in AM–FM modelling and EMD. Recasting EMD as a SA problem provides a new framework towards its comprehension, formulation, and convergence since OMP and LARS converge. Doing so presents an alternative to getting rid of the interpolation process that EMD terribly suffers from. Conducted experiments show noticeable improvements in terms of frequency separation of linear and non-linear signals, tone separation capability and robustness in a noisy environment.
更多查看译文
关键词
learning (artificial intelligence),approximation theory,frequency modulation,signal representation,interpolation,regression analysis,time-frequency analysis,amplitude modulation,signal processing
AI 理解论文
溯源树
样例
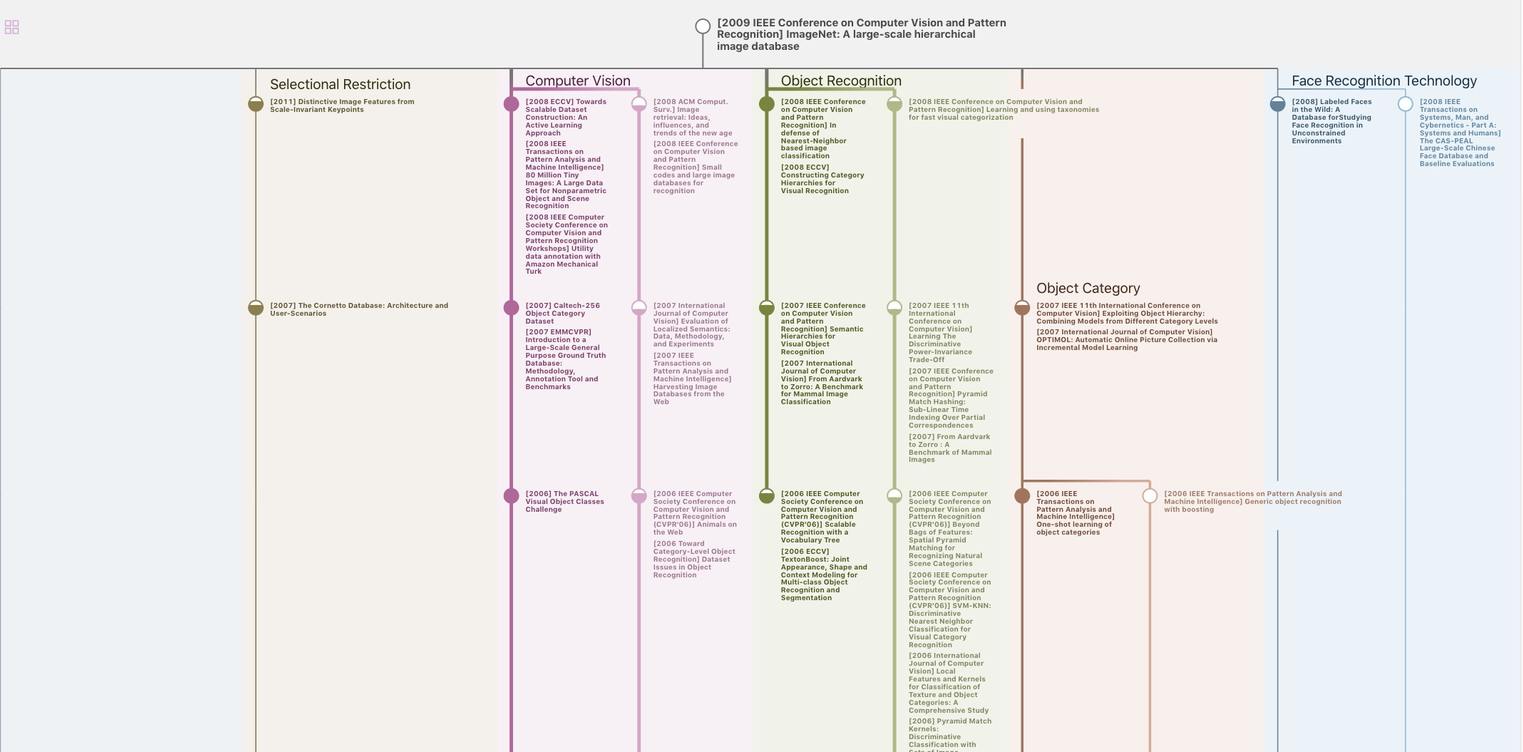
生成溯源树,研究论文发展脉络
Chat Paper
正在生成论文摘要