Local and Global Consistency Regularized Mean Teacher for Semi-supervised Nuclei Classification
Lecture Notes in Computer Science(2019)
摘要
Nucleus classification is a fundamental task in pathology diagnosis for cancers, e.g., Ki-67 index estimation. Supervised deep learning methods have achieved promising classification accuracy. However, the success of these methods heavily relies on massive manually annotated data. Manual annotation for nucleus classification are usually time consuming and laborious. In this paper, we propose a novel semi-supervised deep learning method that can learn from small portion of labeled data and large-scale unlabeled data for nucleus classification. Our method is inspired by the recent state-of-the-art self-ensembling (SE) methods. These methods learn from unlabeled data by enforcing consistency of predictions under different perturbations while ignoring local and global consistency hidden in data structure. In our work, a label propagation (LP) step is integrated into the SE method, and a graph is constructed using the LP predictions that encode the local and global data structure. Finally, a Siamese loss is used to learn the local and global consistency from the graph. Our implementation is based on the state-of-the-art SE method Mean Teacher. Extensive experiments on two nucleus datasets demonstrate that our method outperforms the state-of-the-art SE methods, and achieves F-1 scores close to the supervised methods using only 5%-25% labeled data.
更多查看译文
关键词
Nucleus classification,Semi-supervised learning,Deep learning
AI 理解论文
溯源树
样例
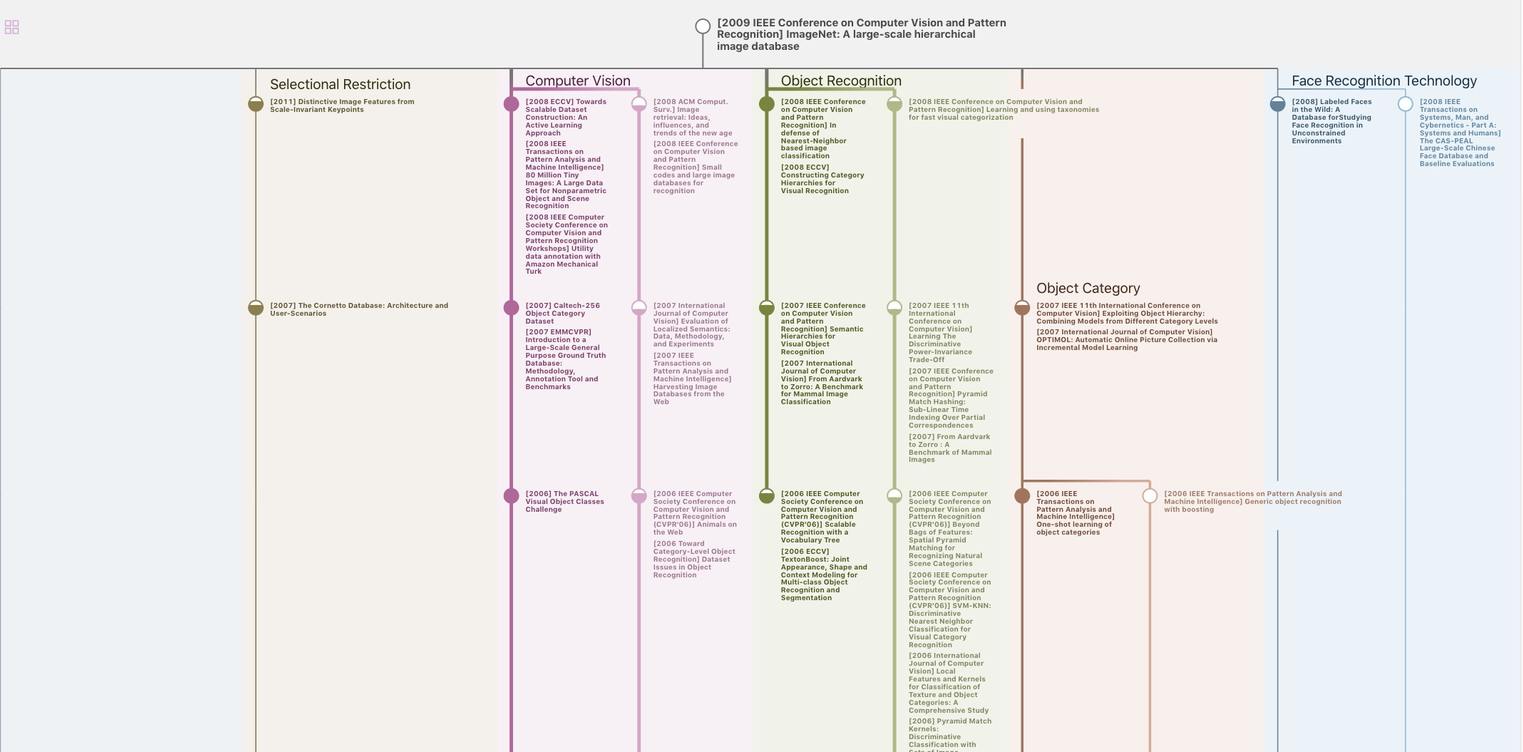
生成溯源树,研究论文发展脉络
Chat Paper
正在生成论文摘要