Information Theoretic Multi-Target Feature Selection via Output Space Quantization.
ENTROPY(2019)
摘要
A key challenge in information theoretic feature selection is to estimate mutual information expressions that capture three desirable terms-the relevancy of a feature with the output, the redundancy and the complementarity between groups of features. The challenge becomes more pronounced in multi-target problems, where the output space is multi-dimensional. Our work presents an algorithm that captures these three desirable terms and is suitable for the well-known multi-target prediction settings of multi-label/dimensional classification and multivariate regression. We achieve this by combining two ideas-deriving low-order information theoretic approximations for the input space and using quantization algorithms for deriving low-dimensional approximations of the output space. Under the above framework we derive a novel criterion, Group-JMI-Rand, which captures various high-order target interactions. In an extensive experimental study we showed that our suggested criterion achieves competing performance against various other information theoretic feature selection criteria suggested in the literature.
更多查看译文
关键词
feature selection,mutual information,multi-target,multi-label,clustering
AI 理解论文
溯源树
样例
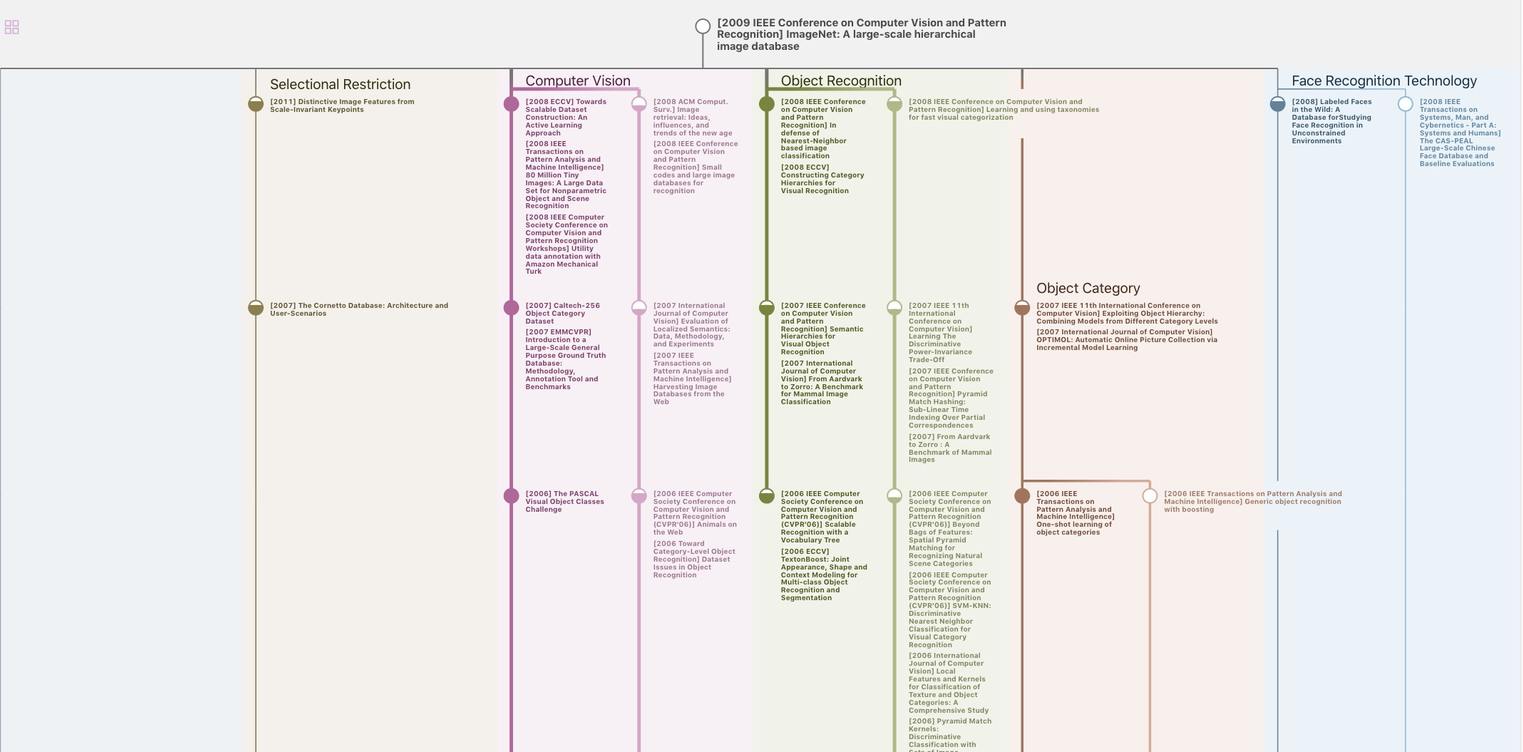
生成溯源树,研究论文发展脉络
Chat Paper
正在生成论文摘要