Make $$\ell _1$$ ℓ 1 regularization effective in training sparse CNN
arxiv(2020)
摘要
Compressed Sensing using
$$\ell _1$$
regularization is among the most powerful and popular sparsification technique in many applications, but why has it not been used to obtain sparse deep learning model such as convolutional neural network (CNN)? This paper is aimed to provide an answer to this question and to show how to make it work.
Following Xiao (J Mach Learn Res 11(Oct):2543–2596, 2010), We first demonstrate that the commonly used stochastic gradient decent and variants training algorithm is not an appropriate match with
$$\ell _1$$
regularization and then replace it with a different training algorithm based on a regularized dual averaging (RDA) method. The RDA method of Xiao (J Mach Learn Res 11(Oct):2543–2596, 2010) was originally designed specifically for convex problem, but with new theoretical insight and algorithmic modifications (using proper initialization and adaptivity), we have made it an effective match with
$$\ell _1$$
regularization to achieve a state-of-the-art sparsity for the highly non-convex CNN compared to other weight pruning methods without compromising accuracy (achieving 95% sparsity for ResNet-18 on CIFAR-10, for example).
更多查看译文
关键词
Sparse optimization,regularization,Dual averaging,CNN
AI 理解论文
溯源树
样例
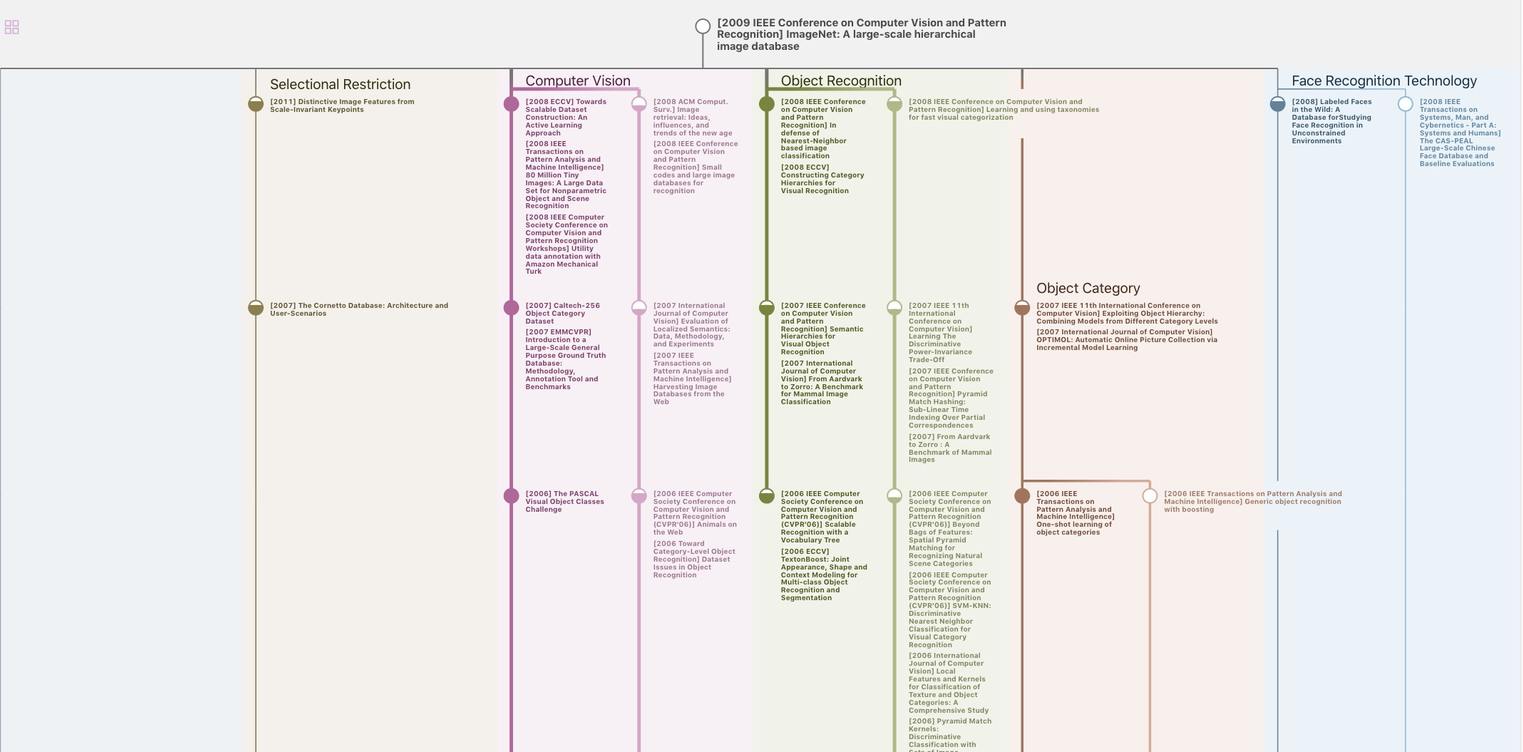
生成溯源树,研究论文发展脉络
Chat Paper
正在生成论文摘要