Privacy-Aware Recommendation with Private-Attribute Protection using Adversarial Learning.
PROCEEDINGS OF THE 13TH INTERNATIONAL CONFERENCE ON WEB SEARCH AND DATA MINING (WSDM '20)(2020)
摘要
Recommendation is one of the critical applications that helps users find information relevant to their interests. However, a malicious attacker can infer users' private information via recommendations. Prior work obfuscates user-item data before sharing it with recommendation system. This approach does not explicitly address the quality of recommendation while performing data obfuscation. Moreover, it cannot protect users against private-attribute inference attacks based on recommendations. This work is the first attempt to build a Recommendation with Attribute Protection (RAP) model which simultaneously recommends relevant items and counters private-attribute inference attacks. The key idea of our approach is to formulate this problem as an adversarial learning problem with two main components: the private attribute inference attacker, and the Bayesian personalized recommender. The attacker seeks to infer users' private-attribute information according to their items list and recommendations. The recommender aims to extract users' interests while employing the attacker to regularize the recommendation process. Experiments show that the proposed model both preserves the quality of recommendation service and protects users against private-attribute inference attacks.
更多查看译文
关键词
Privacy-Aware Recommendation, Private-Attribute Protection, Adversarial Learning, Privacy, Utility
AI 理解论文
溯源树
样例
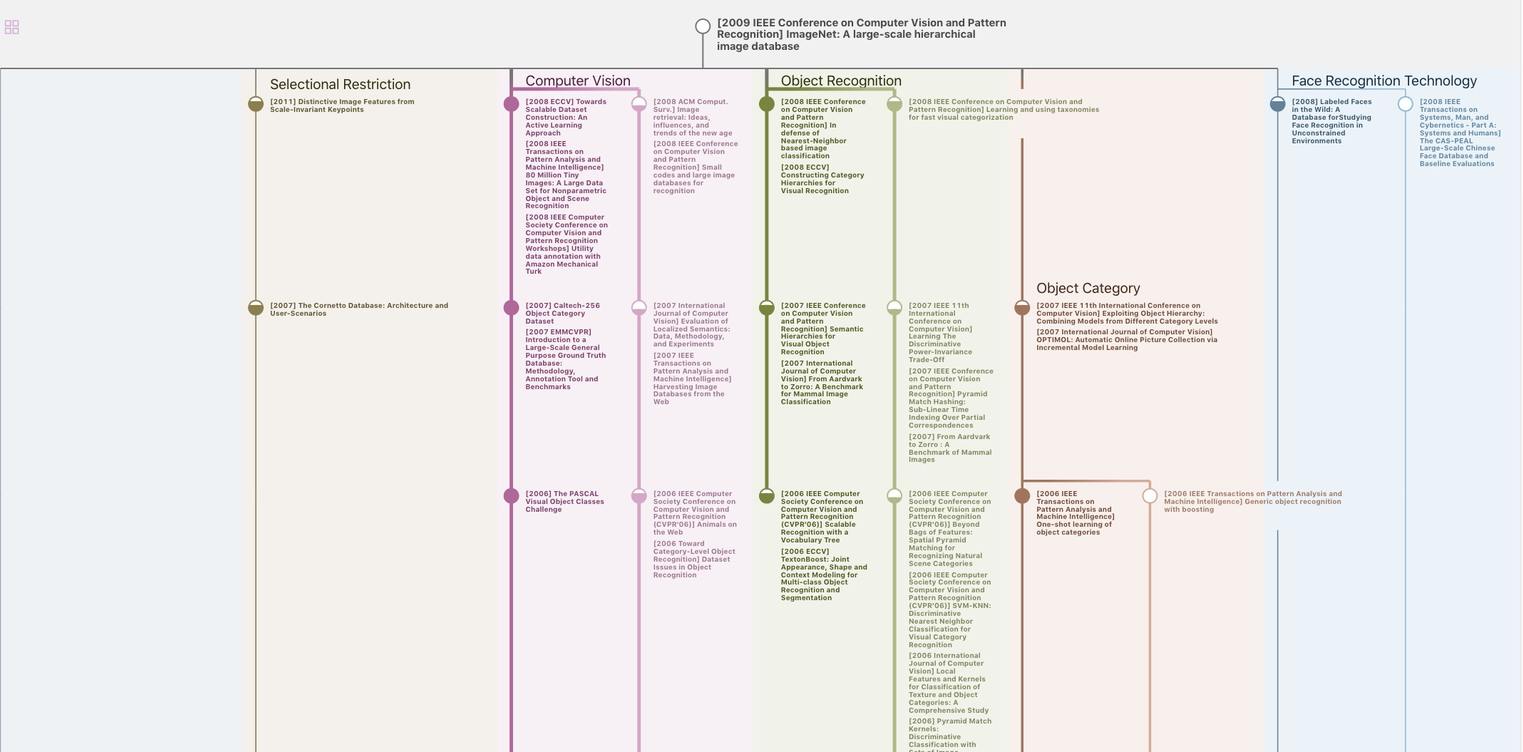
生成溯源树,研究论文发展脉络
Chat Paper
正在生成论文摘要