A Tale Of Two-Timescale Reinforcement Learning With The Tightest Finite-Time Bound
THIRTY-FOURTH AAAI CONFERENCE ON ARTIFICIAL INTELLIGENCE, THE THIRTY-SECOND INNOVATIVE APPLICATIONS OF ARTIFICIAL INTELLIGENCE CONFERENCE AND THE TENTH AAAI SYMPOSIUM ON EDUCATIONAL ADVANCES IN ARTIFICIAL INTELLIGENCE(2020)
摘要
Policy evaluation in reinforcement learning is often conducted using two-timescale stochastic approximation, which results in various gradient temporal difference methods such as GTD(0), GTD2, and TDC. Here, we provide convergence rate bounds for this suite of algorithms. Algorithms such as these have two iterates. theta(n) and w(n), which are updated using two distinct stepsize sequences, alpha(n) and beta(n), respectively. Assuming alpha(n) = n(-alpha) and beta(n) = n(-beta) with 1 > alpha > beta > 0, we show that, with high probability, the two it-erates converge to their respective solutions theta* and w* at rates given by parallel to theta(n )- theta*parallel to* = (O) over tilde (n(-alpha/2)) and parallel to w(n) - w*parallel to = (O) over tilde (n(-alpha/2)); here, O hides logarithmic terms. Via comparable lower bounds, we show that these bounds are, in fact, tight. To the best of our knowledge, ours is the first finite-time analysis which achieves these rates. While it was known that the two timescale components decouple asymptotically, our results depict this phenomenon more explicitly by showing that it in fact happens from some finite time onwards. Lastly, compared to existing works, our result applies to a broader family of stepsizes, including non-square summable ones.
更多查看译文
关键词
learning,two-timescale,finite-time
AI 理解论文
溯源树
样例
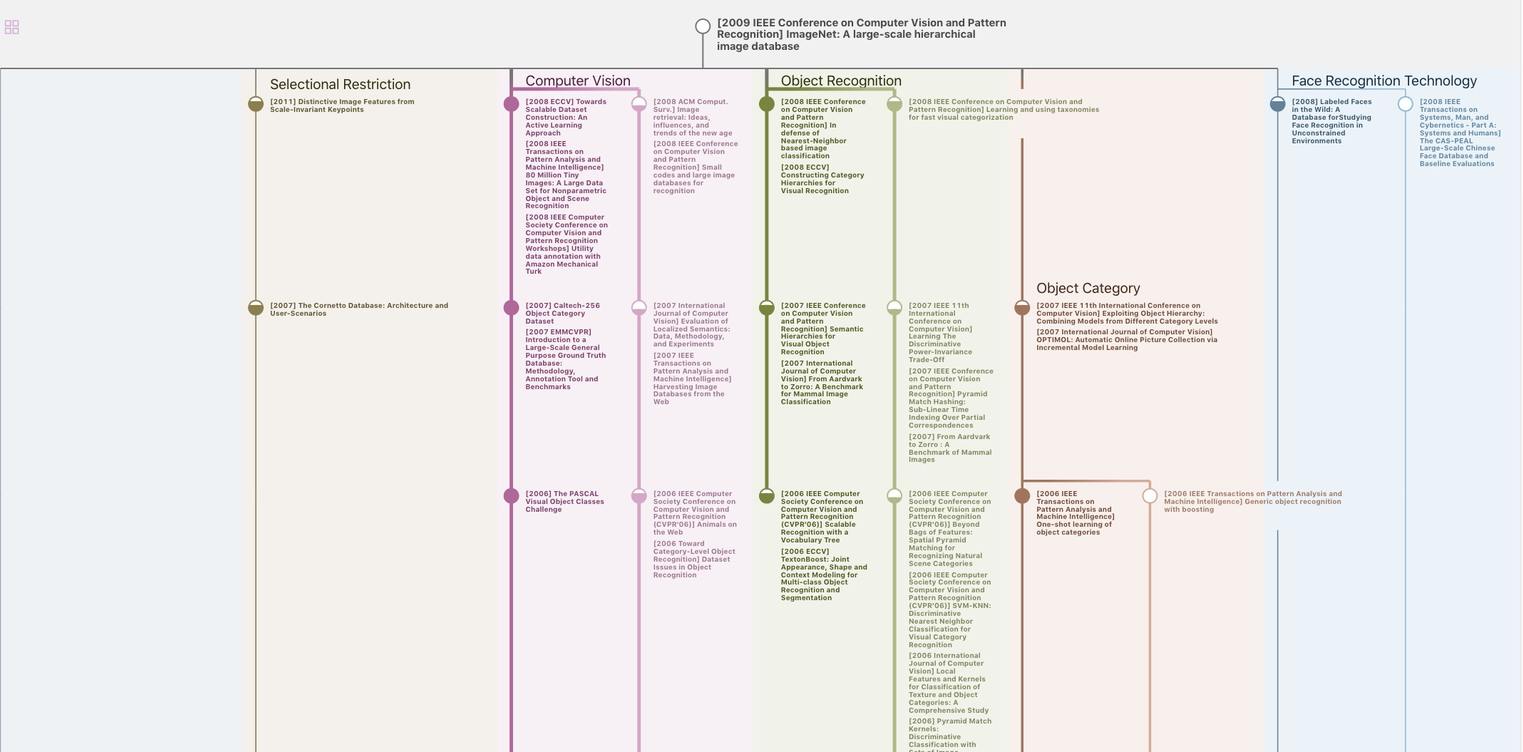
生成溯源树,研究论文发展脉络
Chat Paper
正在生成论文摘要