Learning To Fuse Multiple Semantic Aspects From Rich Texts For Stock Price Prediction
WEB INFORMATION SYSTEMS ENGINEERING - WISE 2019(2019)
摘要
Stock price prediction is challenging due to the non-stationary fluctuation of stock price, which can be influenced by the stochastic trading behaviors in the market. In recent years, researchers have focused on exploiting massive text data such news and tweets to predict stock price, achieving promising outcomes. Existing methods typically compress each text into a fixed-length representation vector, whereas rich texts may involve multiple semantic aspect-level information that has different effects on the future stock price. In this paper, we propose a novel Multi-head Attention Fusion Network (MAFN) to exploit aspect-level semantic information from texts to enhance prediction performance. MAFN employs the encoder-decoder framework, where the encoder adopts the multi-head attention mechanism to automatically learn the aspect-level text representations via different attention heads. Furthermore, we subtly fuse the learned representations by discarding the dross and selecting the essential. The decoder generates stock price sequence by incorporating textual information and historical price dynamically via the hierarchical attention. Experimental results on real data sets show the superior performance of MAFN against several strong baselines as well as the effectiveness of exploiting and fusing fine-grained aspect-level textual information for stock price prediction.
更多查看译文
关键词
Stock price prediction, Multi-head attention, Encoder-decoder
AI 理解论文
溯源树
样例
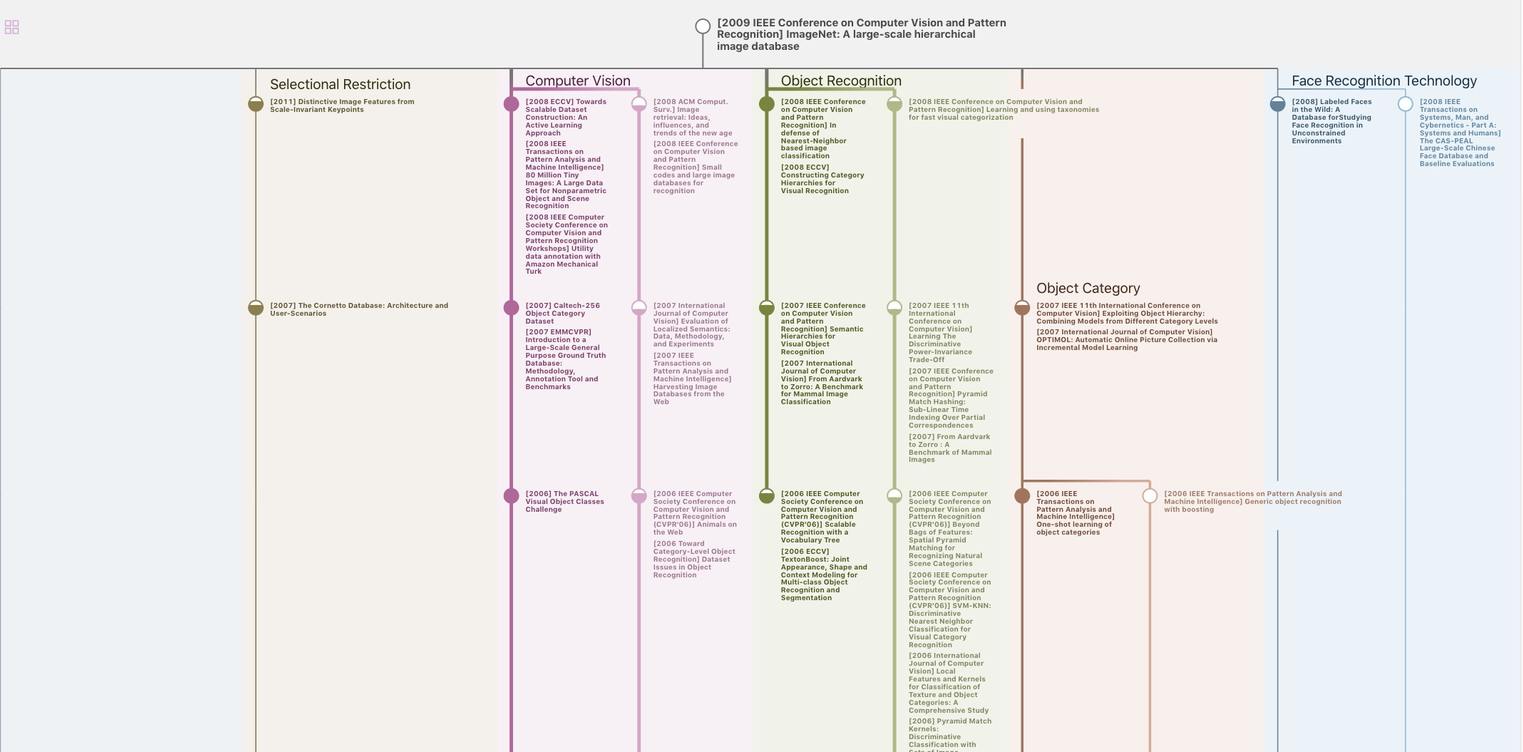
生成溯源树,研究论文发展脉络
Chat Paper
正在生成论文摘要