Graphcpi: Graph Neural Representation Learning For Compound-Protein Interaction
2019 IEEE INTERNATIONAL CONFERENCE ON BIOINFORMATICS AND BIOMEDICINE (BIBM)(2019)
摘要
Accurately predicting compound-protein interactions (CPIs) is of great help to increase the efficiency and reduce costs in drug development. Most of existing machine learning models for CPI prediction often represent compounds and proteins in one-dimensional strings, or use the descriptor-based methods. These models might ignore the fact that molecules are essentially structured by the chemical bond of atoms. However, in real-world scenarios, the topological structure information usually provides an overview of how the atoms are connected, and the local chemical context reveals the functionality of the protein sequence in CPI. These two types of information are complementary to each other and they are both important for modeling compounds and proteins. Motivated by this, this paper suggests an end-to-end deep learning framework called GraphCPI, which captures the structural information of compounds and leverages the chemical context of protein sequences for solving the CPI prediction task. Our framework can integrate any popular graph nerual networks for learning compounds, and it combines with a convolutional neural network for embedding sequences. We conduct extensive experiments based on two benchmark CPI datasets. The experimental results demonstrate that our proposed framework is feasible and also competitive, comparing against classic and state-of-the-art methods.
更多查看译文
关键词
Compound-Protein Interaction, Graph Neural Network, Representation Learning, Drug Discovery
AI 理解论文
溯源树
样例
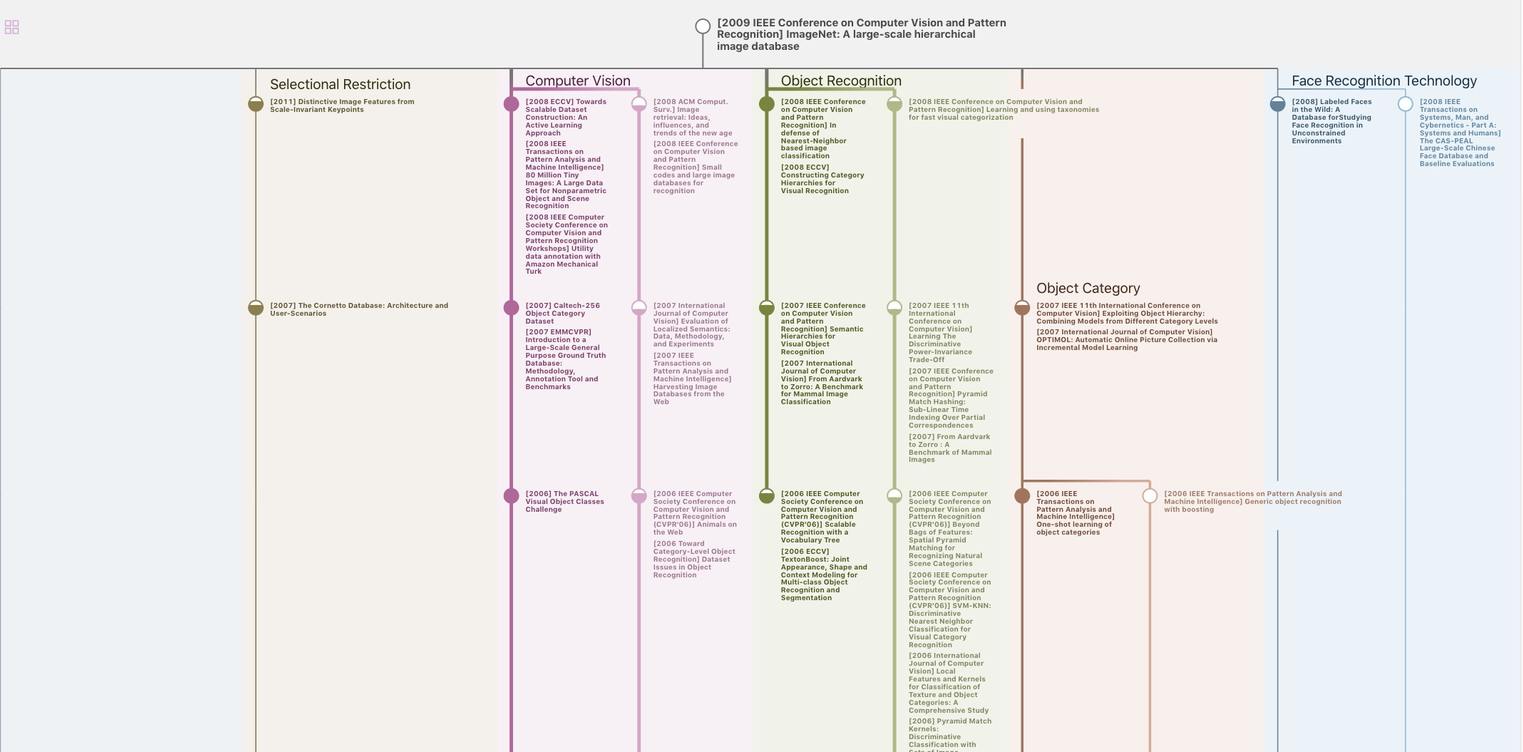
生成溯源树,研究论文发展脉络
Chat Paper
正在生成论文摘要