Sample Balancing for Deep Learning-Based Visual Recognition
IEEE Transactions on Neural Networks and Learning Systems(2020)
摘要
Sample balancing includes sample selection and sample reweighting. Sample selection aims to remove some bad samples that may lead to bad local optima. Sample reweighting aims to assign optimal weights to samples to improve performance. In this article, we integrate a sample selection method based on self-paced learning into deep learning frameworks and study the influence of different sample selection strategies on training deep networks. In addition, most of the existing sample reweighting methods mainly take per-class sample number as a metric, which does not fully consider sample qualities. To improve the performance, we propose a novel metric based on the multiview semantic encoders to reweight the samples more appropriately. Then, we propose an optimization mechanism to embed sample weights into loss functions of deep networks, which can be trained in end-to-end manners. We conduct experiments on the CIFAR data set and the ImageNet data set. The experimental results demonstrate that our proposed sample balancing method can improve the performances of deep learning methods in several visual recognition tasks.
更多查看译文
关键词
Training,Visualization,Semantics,Deep learning,Measurement,Task analysis,Image recognition
AI 理解论文
溯源树
样例
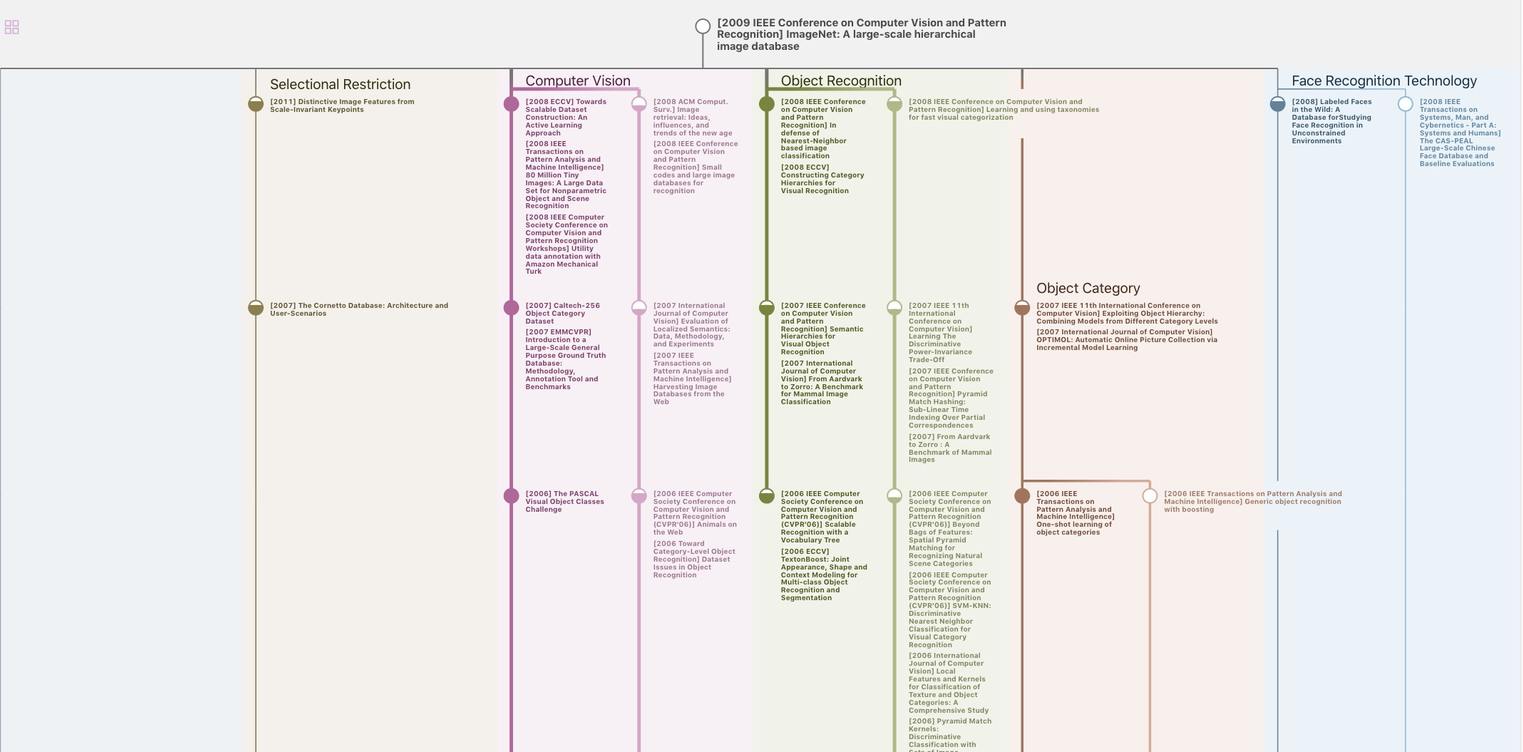
生成溯源树,研究论文发展脉络
Chat Paper
正在生成论文摘要