Towards Real-Time User Qoe Assessment Via Machine Learning On Lte Network Data
2019 IEEE 90TH VEHICULAR TECHNOLOGY CONFERENCE (VTC2019-FALL)(2019)
摘要
It is well known that current reactive network management would be unable to support the exponential increase in complexity and rapidity of change in future cellular networks. Keeping this in perspective, the goal of this paper is to investigate applicability of machine learning and predictive models to assess cell-level user quality of experience (QoE) in real-time. For this purpose, we leverage a 5-week LTE metrics data collected at cell level granularity for a national LTE network operator. Domain knowledge is applied to assess user QoE with network key performance indicators (KPIs), namely scheduled user throughput, inter-frequency handover success rate and intra-frequency handover success rate. Results indicate that applying boosted trees model on a subset of carefully selected non-collinear features allows high accuracy threshold-based estimation of user throughput and inter-frequency handover success rate. We also exploit the periodic nature of cell data characteristics and apply a recently developed time series prediction model known as PROPHET for future QoE estimation. By employing machine learning and data analytics on network data within an end-to-end framework, network operators can proactively identify low performance cell sites along with the influential factors that impact the cell performance. Based on the root cause analysis, appropriate corrective measures may then be taken for low performance cell sites.
更多查看译文
关键词
Machine learning, user quality-of-experience, PROPHET model, gradient boosted trees, mobile network data
AI 理解论文
溯源树
样例
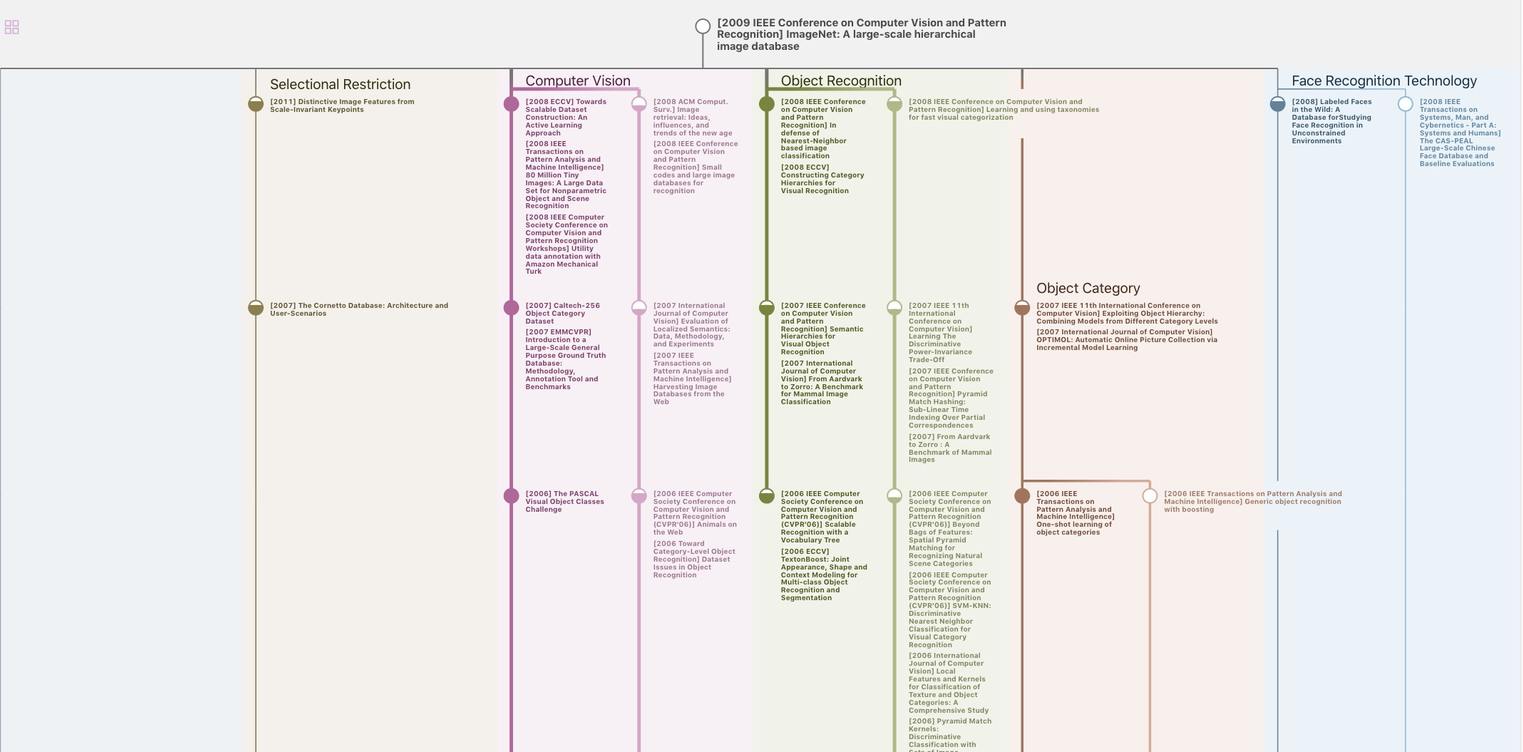
生成溯源树,研究论文发展脉络
Chat Paper
正在生成论文摘要