Machine learning based design space exploration for hybrid main-memory design
Proceedings of the International Symposium on Memory Systems(2019)
摘要
We develop a machine learning (ML) based design space exploration (DSE) method that builds predictive models for various responses of a hybrid main-memory system. To overcome the challenges associated with latency, capacity, and power of memory systems in future extreme-scale machines, the hybrid memory architectures are being considered in which novel non-volatile memory (NVM) systems augment the traditional DRAM. However, way before their actual design and implementation, these emerging hybrid memory systems need to be simulated and analyzed to fully understand their capabilities and limitations. As the conventional architectural-level memory simulators require significant amounts of computational costs and time, we propose to utilize ML techniques for developing various memory-response models that can instantly provide a predicted response corresponding to any new memory configuration. Specifically, in this work, we apply four supervised ML techniques to build regression models for memory latency, bandwidth, power, and total read/write responses. The training and validation data for the ML methods are generated using NVMain memory simulator for DRAM, NVM, and their hybrid combinations. We demonstrate the results of the ML based memory-DSE method in terms of the learning curve characteristics for hyperparameter tuning and the statistical error analyses of the designed predictive models.
更多查看译文
关键词
design space exploration, hybrid main memory, learning curves, machine learning, nonvolatile memory, predictive models
AI 理解论文
溯源树
样例
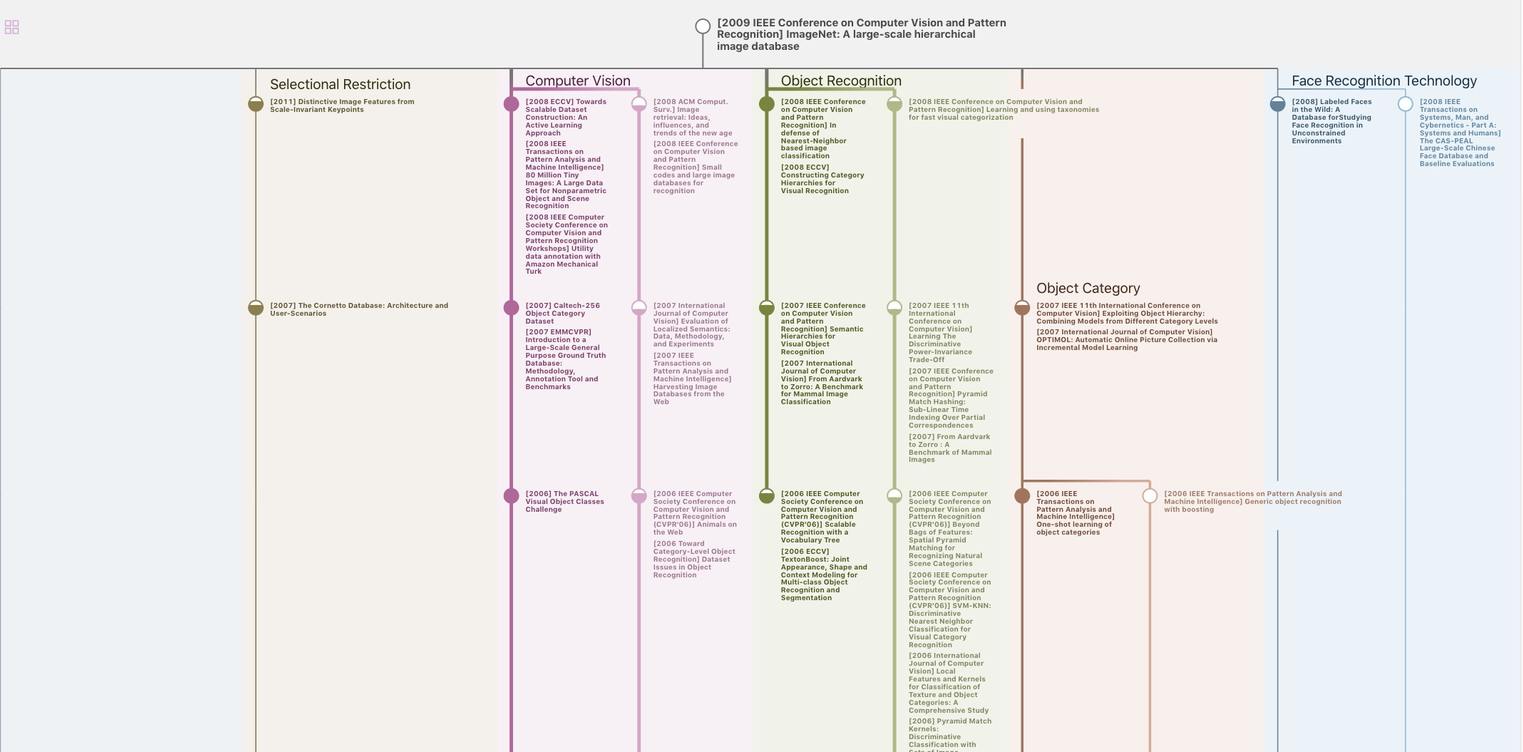
生成溯源树,研究论文发展脉络
Chat Paper
正在生成论文摘要