High speed and energy efficient deep neural network for edge computing.
SEC(2019)
摘要
Edge computing enables data-stream acceleration with realtime data processing without latency, and allows for efficient data processing in that large amounts of data can be processed near the source with the ability to process data without ever putting it into a public cloud adds a useful layer of security for sensitive data. The edge computing-based architecture design and analysis play key impacts for the future Internet of Things (IoT) infrastructure development. In this work, we design a low power hybrid structured deep neural network (Hybrid-DNN), which employs memristive synapses working in a hierarchical information processing fashion and delay-based spiking neural network (SNN) modules as the readout layer, and provide a novel data layout method to allow the Hybrid DNN running a computationally intensive deep learning algorithm on limited resource edge devices. Motivated by the recent findings in neuromorphic computing and edge computing, we design a hybrid structured DNN combining both depth-in-space (spatial) and depth-in-time (temporal) deep learning architectures. Our Hybrid-DNN employs memristive synapses working in a hierarchical information processing fashion and delay-based spiking neural network modules as the readout layer.
更多查看译文
关键词
Edge computing, spiking neural network, deep learning
AI 理解论文
溯源树
样例
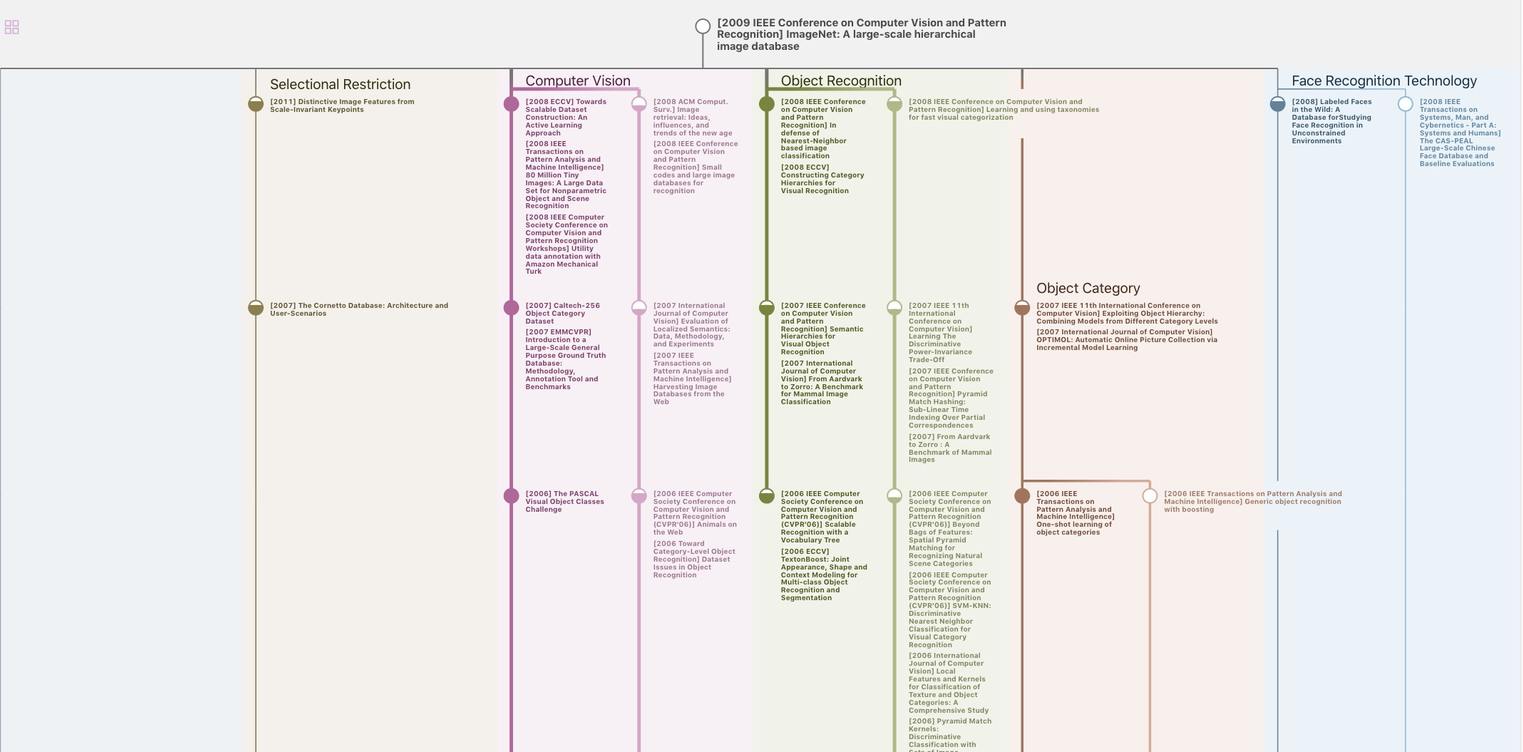
生成溯源树,研究论文发展脉络
Chat Paper
正在生成论文摘要