A note on convex relaxations for the inverse eigenvalue problem
Optimization Letters(2021)
摘要
The affine inverse eigenvalue problem consists of identifying a real symmetric matrix with a prescribed set of eigenvalues in an affine space. Due to its ubiquity in applications, various instances of the problem have been widely studied in the literature. Previous algorithmic solutions were typically nonconvex heuristics and were often developed in a case-by-case manner for specific structured affine spaces. In this short note we describe a general family of convex relaxations for the problem by reformulating it as a question of checking feasibility of a system of polynomial equations, and then leveraging tools from the optimization literature to obtain semidefinite programming relaxations. Our system of polynomial equations may be viewed as a matricial analog of polynomial reformulations of 0/1 combinatorial optimization problems, for which semidefinite relaxations have been extensively investigated. We illustrate numerically the utility of our approach in stylized examples that are drawn from various applications.
更多查看译文
关键词
Combinatorial optimization,Real algebraic geometry,Schur–Horn orbitope,Semidefinite programming,Sums of squares polynomials
AI 理解论文
溯源树
样例
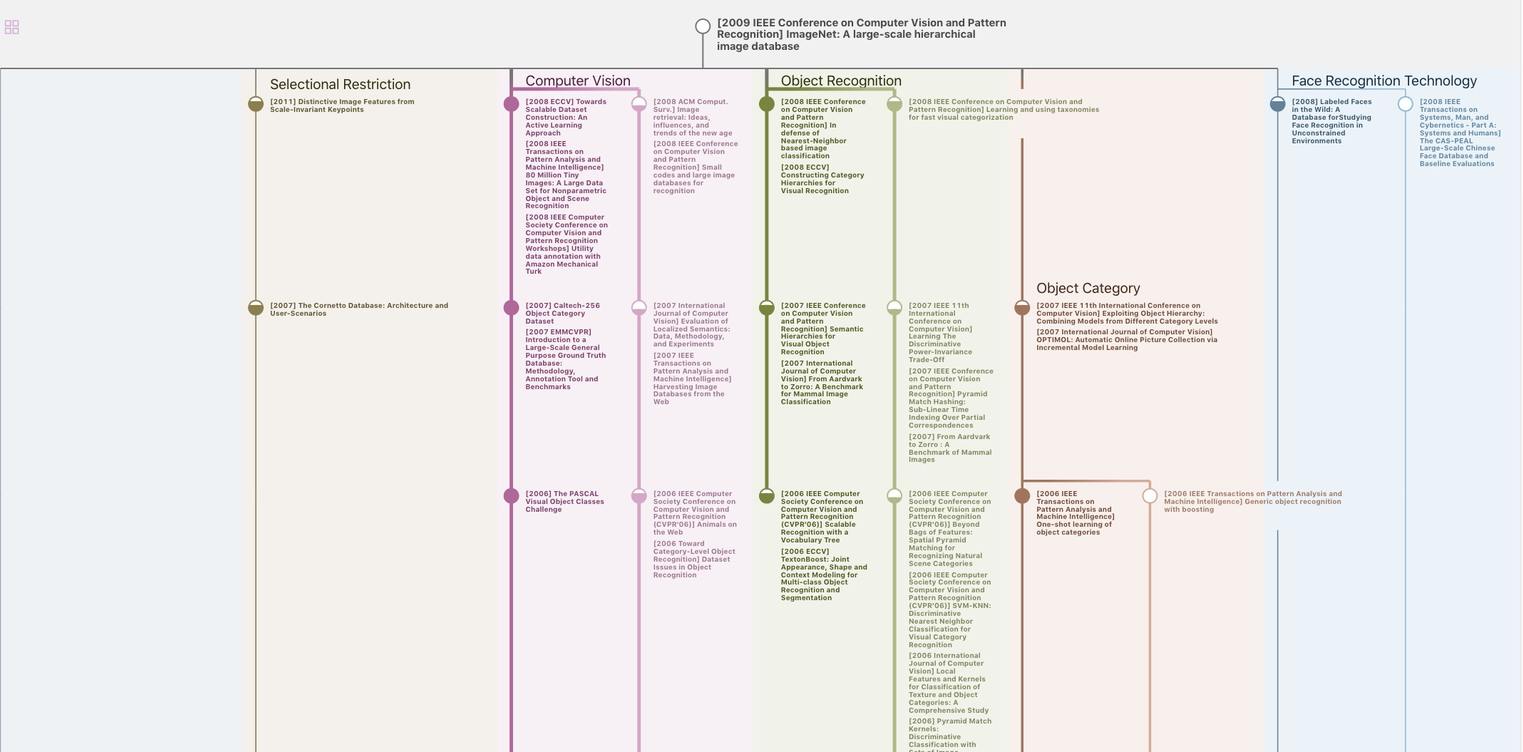
生成溯源树,研究论文发展脉络
Chat Paper
正在生成论文摘要