Online Model Management via Temporally Biased Sampling
ACM SIGMOD Record(2019)
摘要
To maintain the accuracy of supervised learning models in the presence of evolving data streams, we provide temporallybiased sampling schemes that weight recent data most heavily, with inclusion probabilities for a given data item decaying exponentially over time. We then periodically retrain the models on the current sample. We provide and analyze both a simple sampling scheme (T-TBS) that probabilistically maintains a target sample size and a novel reservoirbased scheme (R-TBS) that is the first to provide both control over the decay rate and a guaranteed upper bound on the sample size. The R-TBS and T-TBS schemes are of independent interest, extending the known set of unequalprobability sampling schemes. We discuss distributed implementation strategies; experiments in Spark show that our approach can increase machine learning accuracy and robustness in the face of evolving data.
更多查看译文
AI 理解论文
溯源树
样例
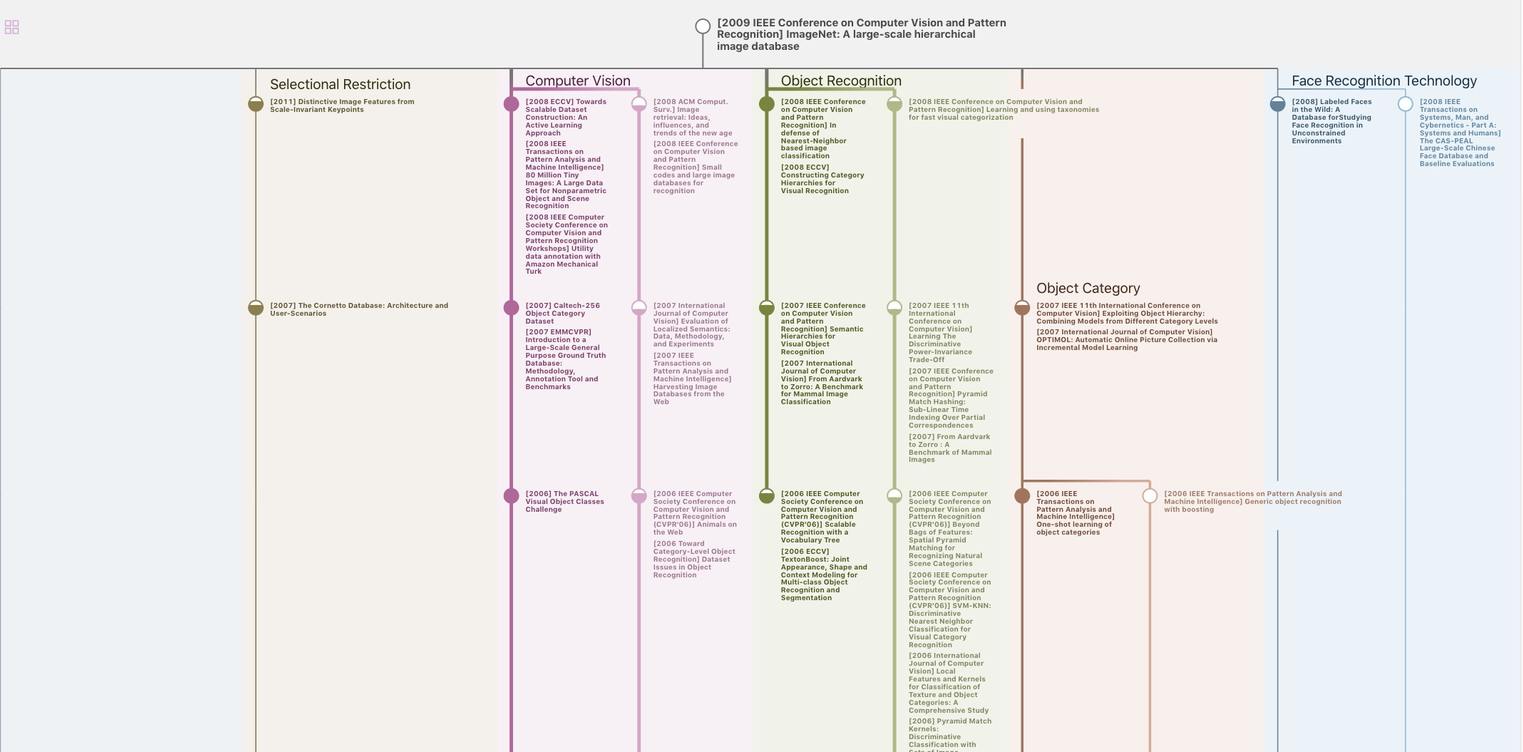
生成溯源树,研究论文发展脉络
Chat Paper
正在生成论文摘要