Fusing Mfcc And Lpc Features Using 1d Triplet Cnn For Speaker Recognition In Severely Degraded Audio Signals
IEEE TRANSACTIONS ON INFORMATION FORENSICS AND SECURITY(2020)
摘要
Speaker recognition algorithms are negatively impacted by the quality of the input speech signal. In this work, we approach the problem of speaker recognition from severely degraded audio data by judiciously combining two commonly used features: Mel Frequency Cepstral Coefficients (MFCC) and Linear Predictive Coding (LPC). Our hypothesis rests on the observation that MFCC and LPC capture two distinct aspects of speech, viz., speech perception and speech production. A carefully crafted 1D Triplet Convolutional Neural Network (1D-Triplet-CNN) is used to combine these two features in a novel manner, thereby enhancing the performance of speaker recognition in challenging scenarios. Extensive evaluation on multiple datasets, different types of audio degradations, multi-lingual speech, varying length of audio samples, etc. convey the efficacy of the proposed approach over existing speaker recognition methods, including those based on iVector and xVector.
更多查看译文
关键词
Speaker recognition, Speech recognition, Noise measurement, Mel frequency cepstral coefficient, Speech processing, Feature extraction, Production, Speaker recognition, degraded audio, deep learning, MFCC, LPC, 1-D CNN, feature-level fusion
AI 理解论文
溯源树
样例
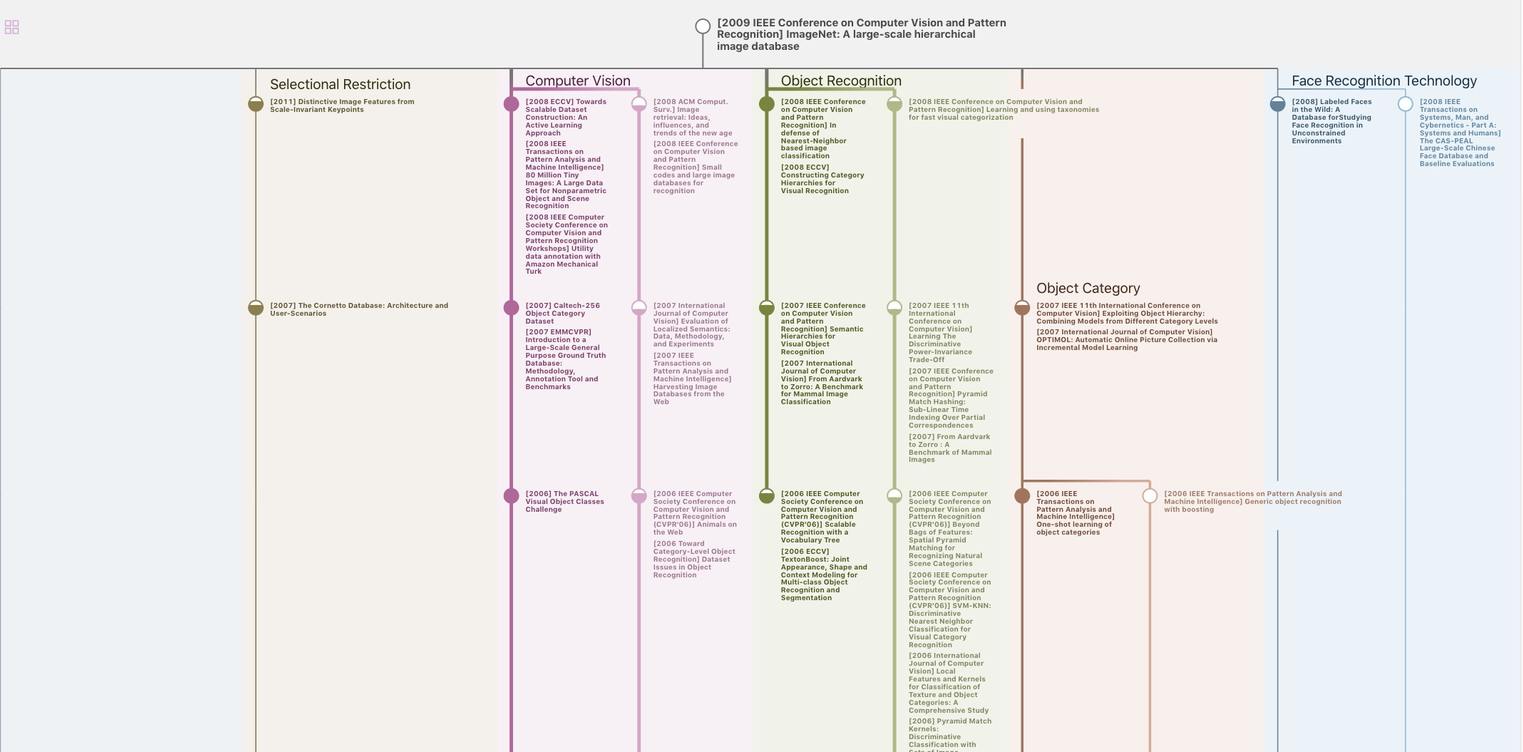
生成溯源树,研究论文发展脉络
Chat Paper
正在生成论文摘要