Adaptive Data Clustering Ensemble Algorithm Based on Stability Feature Selection and Spectral Clustering
2019 2nd International Conference on Artificial Intelligence and Big Data (ICAIBD)(2019)
摘要
Data Clustering algorithms are essential techniques to pattern recognition. While many clustering algorithms have been proposed, none of them could gain a desirable result on various pattern recognition problems. In this paper, an adaptive clustering ensemble algorithm based on stability feature selection and spectral clustering for data clustering is proposed. The paper chooses strong features by stability feature selection, aiming to improve the quality of clustering. LIMBO is then applied on dataset to engender ensemble instances via calculating the similarity of ensemble members, a proximity matrix could be formed. The cluster ensemble problem is then formalized as a combinatorial spectral clustering problem. Empirical studies on several benchmarks from UC Irvine Machine Learning Repository shows the favorites of the proposed algorithm compares to its competitors, and the promising results yields new inspiration of cluster ensemble and spectral clustering.
更多查看译文
关键词
clustering ensemble,feature selection,matrix eigengap,spectral clustering,similarity matrix
AI 理解论文
溯源树
样例
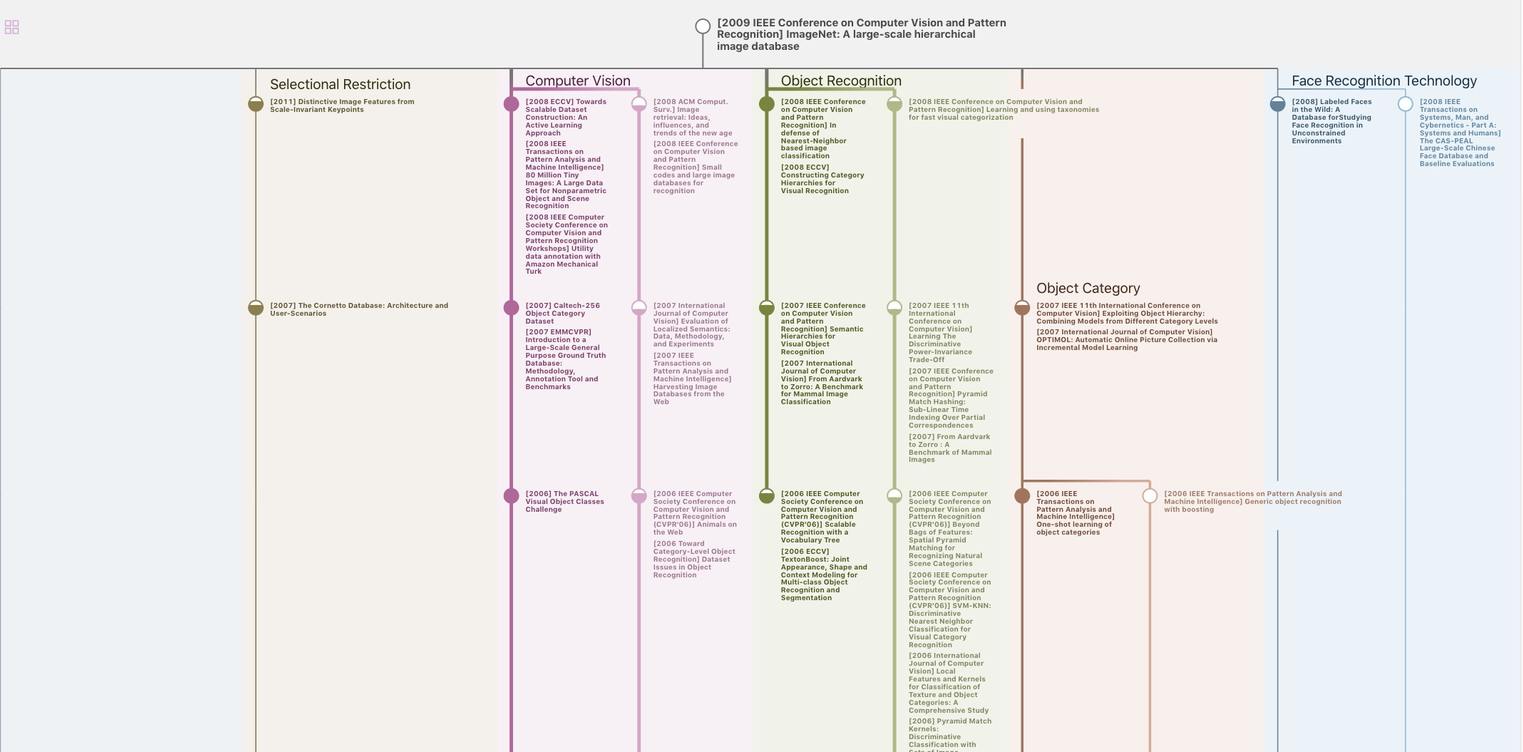
生成溯源树,研究论文发展脉络
Chat Paper
正在生成论文摘要