Speaker-Invariant Feature-Mapping for Distant Speech Recognition via Adversarial Teacher-Student Learning
INTERSPEECH(2019)
摘要
Feature mapping (FM) jointly trained with acoustic model (AFM) is commonly used for single-channel speech enhancement. However, the performance is affected by the inter-speaker variability. In this paper, we propose speaker-invariant AFM (SIAFM) aiming at curtailing the inter-talker variability while achieving speech enhancement. In SIAFM, a feature-mapping network, an acoustic model and a speaker classifier network are jointly optimized to minimize the feature-mapping loss and the senone classification loss, and simultaneously min-maximize the speaker classification loss. Evaluated on AMI dataset, the proposed SIAFM achieves 4.8% and 7.0% relative word error rate (WER) reduction on the overlapped and non-overlapped condition over the baseline acoustic model trained with single distant microphone (SDM) data. Additionally, the SIAFM obtains 3.0% relative overlapped WER and 4.2% relative nonoverlapped WER decrease over the multi-conditional (MCT) acoustic model. To further promote the performance of SIAFM, we employ teacher-student learning (TS), in which the posterior probabilities generated by the individual headset microphone (IHM) data can be used in lieu of labels to train the SIAFM model. The experiments show that compared with MCT model, SIAFM with TS (SIAFM-TS) can reach 4.2% relative overlapped WER and 6.3% relative non-overlapped WER decrease respectively.
更多查看译文
关键词
far-field speech recognition, speech enhancement, adversarial learning, teacher-student learning
AI 理解论文
溯源树
样例
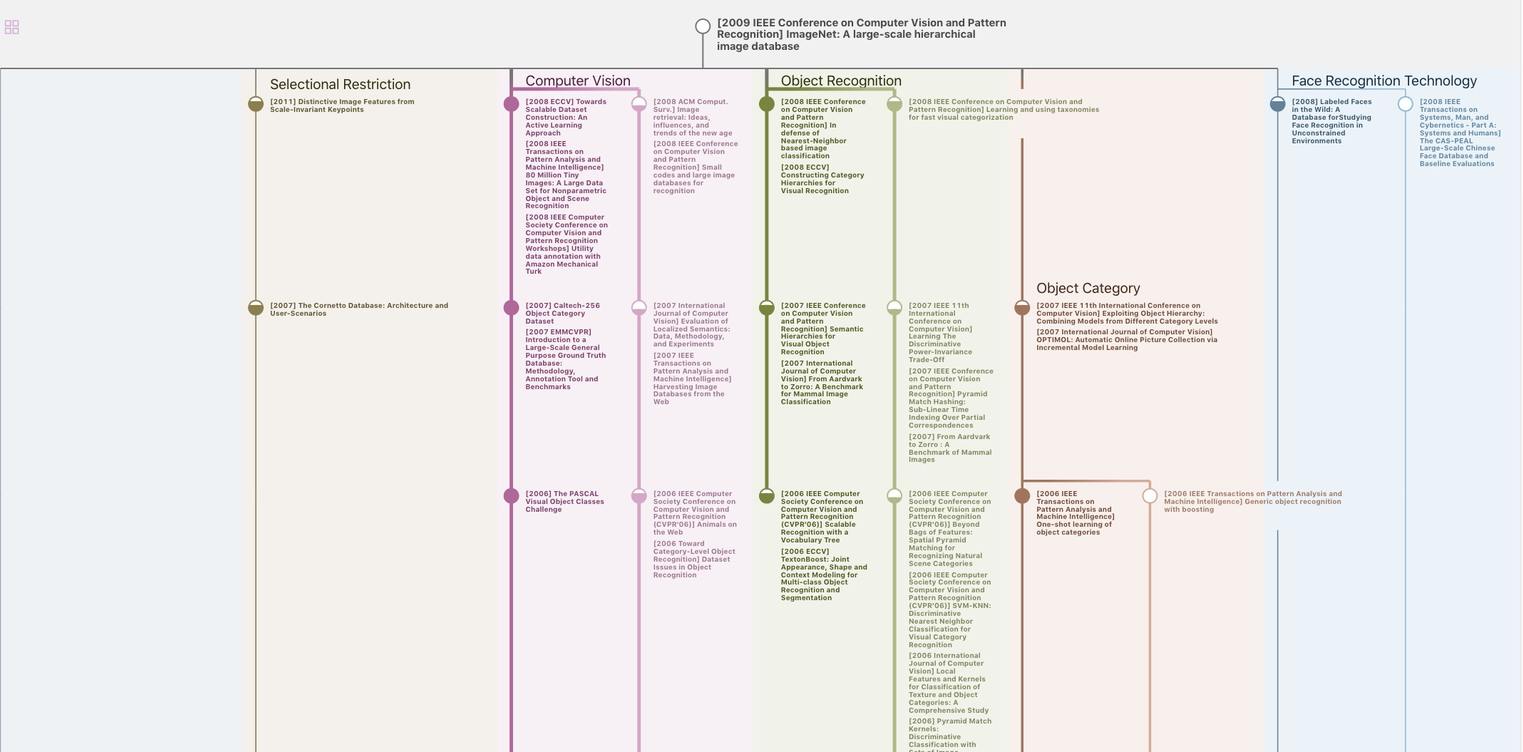
生成溯源树,研究论文发展脉络
Chat Paper
正在生成论文摘要